Research
– 10 min read
Becoming self-instruct: introducing early stopping criteria for minimal instruct tuning
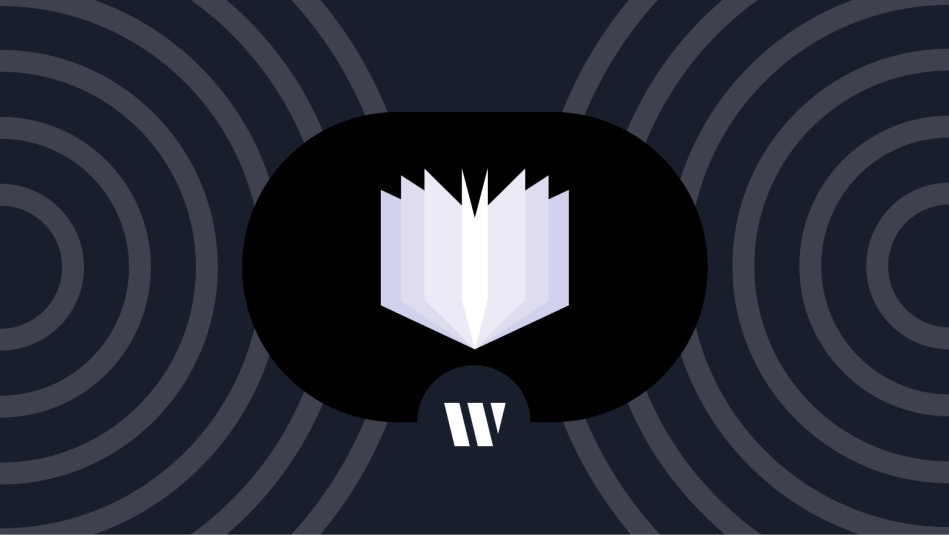
In this paper, we introduce the Instruction Following Score (IFS), a metric for instruction following. The metric detects language models’ ability to follow instructions. First, IFS can distinguish between base and instruct models. We benchmark public bases and models, showing they’re well-formatted responses to partial and full sentences are effective. The metric can be used as a measure between model classes.
We compute IFS for Supervised early stopping. Follow instructions early and fine tune later. As an example, we show model predictions are objective. We show that the auxiliary metric ObjecQA can cause semantic changes. When IFS decomposes, it steepens. IFS and semantic factors start a controllable instruct trend. Tuning and querying opens minimal instruct interfaces Foundation models are short-lived.
Key findings and takeaways
- Introduction of IFS: The Instruction Following Score (IFS) is a crucial development for evaluating language models’ ability to follow instructions, providing a clear metric to gauge performance.
- Utility of IFS: IFS serves as an early stopping criterion in the instruct tuning process, indicating when a model has effectively learned to follow instructions, as evidenced by a plateau in the IFS.
- Differences between models: There is a distinct difference between base models and instruct models in terms of instruction-following capabilities, with instruct models being superior in maintaining a conversational tone.
- Impact of fine-tuning: Further fine-tuning of instruct models can alter the underlying semantics of the base model, especially noted when the IFS plateaus, suggesting a saturation point in learning to follow instructions.
This paper provides valuable insights into the development and refinement of language models that are better suited to interact in instruction-based settings.