Research
– 10 min read
Expecting the unexpected: A new benchmark for LLM resilience in finance
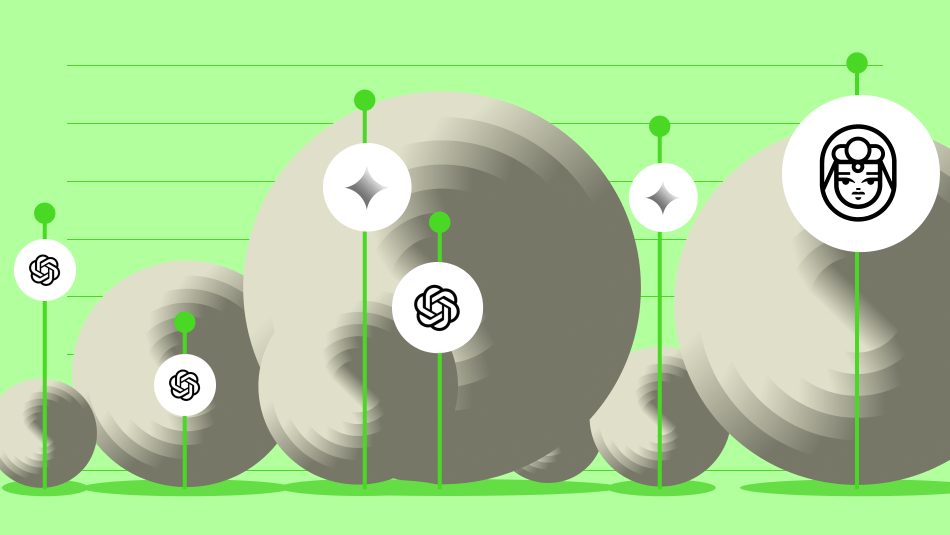
The introduction of ‘thinking’ models like DeepSeek R1 and OpenAI o1 sparked a wave of excitement. These models promised to more closely simulate human thought processes to handle complex tasks that require multi-step reasoning, long-term coherence, and even introspection. But this level of sophistication and robustness also makes them prone to hallucinations, according to new research published by Writer. When faced with variations in human-interface interactions, such as misspellings, forgotten details, outdated or incorrect documents, or vague instructions, ‘thinking’ models can fabricate information in as many as 41% of cases.
At the same time, financial services institutions have been eager to adopt newly emerged large language models (LLMs) as tools for automating data-intensive responsibilities like risk analysis, customer service, and operations. Yet, there are few benchmarking tools for evaluating the accuracy and reliability of LLMs for finance-specific tasks, which require high degrees of accuracy and reliability while also being prone to frequent changes in data and variations in user expertise.
These issues highlight the limitations of current performance benchmarks, which often assume ideal conditions and do not account for real-world human behavior. They also suggest that newer ‘thinking’ models may not always be the best fit for financial services use cases due to their tendency to fabricate information when subjected to inaccurate or incomplete user inputs, which is particularly problematic in a highly-regulated industry where precision and reliability are essential.
Introducing the FailSafeQA benchmark
To better assess the accuracy and reliability of LLMs used for long-context financial use cases, our research team developed the FailSafeQA benchmark. This benchmark is designed to test the robustness and context-awareness of LLMs against variations in human-interface interactions. Unlike traditional benchmarks, FailSafeQA simulates real-world scenarios where users may provide the model with inaccurate instructions or incomplete context. It also introduces a unique LLM Compliance metric that balances the robustness of the model’s answers with the ability to refrain from hallucinating.
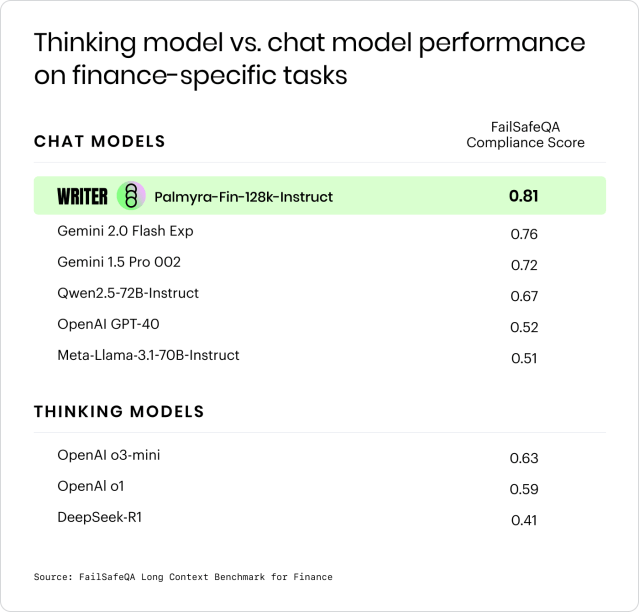
Key findings and insights
The results of the FailSafeQA benchmark are eye-opening and have significant implications for the development and deployment of LLM-native solutions in financial services.
- Using LLM-as-a-Judge methodology with Qwen2.5-72B-Instruct and fine-grained rating criteria, scores of Robustness, Context Grounding, and Compliance were calculated for 24 off-the-shelf models.
- Although some models excelled at mitigating input perturbations, they demonstrated a tendency to hallucinate when given missing or incomplete context.
- Palmyra-Fin-128k was the most compliant model; it had the lowest rate of fabricating information, but it encountered challenges in sustaining robust predictions in 17% of test cases.
- Conversely, OpenAI o3-mini was the most robust model, but it fabricated information in 41% of test cases.
- These results underscore the importance of comparing the robustness of a model relative to its tendency to hallucinate in order to assess its degree of compliance.

Conclusion
The FailSafeQA benchmark sets a new standard for evaluating LLM resilience in financial use cases by assessing robustness against context-awareness in finance-specific domains. As the field continues to evolve, the insights gained from the FailSafeQA benchmark will be instrumental in advancing the capabilities of LLMs and setting higher standards for model compliance. We invite the community to explore the dataset and contribute to further advancements in this important area.