Thought leadership
– 10 min read
Synthetic data: Busting the myths holding back enterprise AI progress
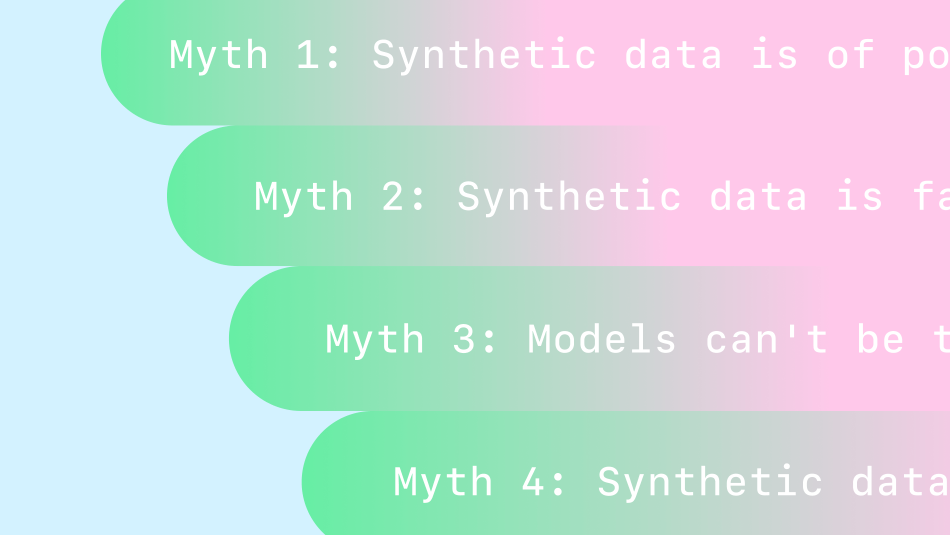
The traditional approach of using massive datasets for AI training is becoming unsustainable due to diminishing returns, limited data availability, and high costs. At Writer, we’ve been training our models with synthetic data for some time. This has led to the development of Palmyra 004, a high-performing model trained entirely on synthetic data at a much lower cost. We address four common myths: Synthetic data is low-quality, fake, ineffective for training models, and risky. In reality, synthetic data can be highly realistic, reliable, and ethical — offering a cost-effective and efficient solution for enterprise AI.
For the past decade, AI researchers from big tech to major labs have pursued a “bigger is better” approach to large language model training. Common wisdom has been that the more data you pour into training models, the greater intelligence you’ll get out. Many believed in a new “Moore’s law” for generative AI. Many LLMs rapidly exploded in size to trillions of parameters, with billions of dollars being invested into “the scale data at all costs” approach. Those assumptions now appear to be wrong.
From Andrej Karpathy to Ilya Sutskever, folks are ringing alarms that there’s a ceiling to scaling. Bigger models are seeing diminishing returns on performance. The supply of publicly-available text data might be exhausted within five years, the energy requirements for training large models are becoming increasingly prohibitive, and a small number of manufacturers limit production capacity for advanced chips. These factors together suggest that simply increasing model size is no longer a viable strategy.
At Writer, we believe the future belongs to precision training and architectural innovation. To address scaling challenges and to stay nimble in our ability to deliver the enterprise-grade capabilities needed to drive ROI within businesses, we’ve been efficiently training models with synthetic data for some time. Our innovative approaches with synthetic data have led to Palmyra 004. It’s the first top-performing frontier model of its size to be developed with synthetic data and at a fraction of the cost reported by the major AI labs — a major breakthrough in efficient, scalable model training.
Now‌ everyone is catching on that synthetic data may hold the key to breaking through AI scaling laws. The problem is, most people don’t even know what “synthetic data” actually means. The “synthetic” aspect causes assumptions that such data is fake and of poor quality. They worry it causes model collapse, costs a lot, and exposes sensitive information.
Let’s set the record straight. We’re going to debunk some of the common myths around synthetic data and equip you with the facts so you can confidently make informed decisions when you look at vendors for your enterprise AI initiatives.
Myth 1: Synthetic data is of poor quality
One of the most persistent myths surrounding synthetic data is that it’s inherently of poor quality. Critics often question its reliability and accuracy, but the reality is far more nuanced and promising.
Advanced models, such as Generative Adversarial Networks (GANs), have revolutionized the way synthetic data is created. These models can generate data that’s not only highly realistic but also incredibly diverse. Whether you need synthetic text, images, or numerical data, GANs and other sophisticated algorithms can produce datasets that closely mimic real-world scenarios, ensuring that the data is both rich and varied.
Verification is a critical step in the synthetic data process. By treating synthetic data as “verified data augmentation,” we can ensure that it meets the highest standards of quality and reliability. This involves rigorous testing and validation to confirm that the synthetic data accurately reflects the properties and characteristics of real data. When done correctly, this verification process can result in synthetic data that is not only as good as real data but can even outperform human-generated data in many cases.
For instance, synthetic data can be engineered to eliminate noise and inconsistencies that are often present in real data. It can be tailored to specific training needs, ensuring that the AI model receives the most relevant and high-quality input. This precision and control make synthetic data an invaluable tool for training large language models (LLMs) and other AI systems, leading to more reliable and effective outcomes.
Myth 2: Synthetic data is fake or hallucinated
The idea that synthetic data is just “fake” or “hallucinated” is a significant misunderstanding. People often arrive at it because they don’t know what the difference is between “real” data and synthetic data. So let’s break it down.
Real data is data that’s collected from actual events, transactions, or observations. It reflects genuine, real-world scenarios and includes all the complexities, variations, and potential noise and risk that come with it.
For example, a dataset of actual credit card transactions, including real customer names, transaction dates, amounts, and merchant details. This data is collected from real transactions and can be used to analyze spending patterns, detect fraud, and understand customer behavior.
Synthetic data is data that’s artificially generated to mimic the properties and characteristics of real data. It’s created using algorithms and can be tailored to specific needs, ensuring it is clean, consistent, and free from privacy and copyright concerns.
For example, a dataset that simulates credit card transactions but uses fictional customer names, dates, amounts, and merchant details. The synthetic data is designed to have the same statistical properties and patterns as the real data but without any real individuals being represented, thus avoiding privacy issues and ensuring the data is clean and consistent.
At Writer, we use a proprietary synthetic data pipeline that transforms real, factual data into synthetic data. This ensures that the data is not only high-quality but also highly relevant, reducing the need for massive datasets while maintaining the accuracy and performance of our models.
Myth 3: Models can’t be trained solely on synthetic data
The notion that AI models can’t be trained exclusively on synthetic data is a misconception that needs to be dispelled. Contrary to popular belief, synthetic data can not only provide high accuracy and reliability but often outperforms real data in various scenarios. It has the potential to improve model performance, demonstrating that it can be a viable substitute for real data, even with up to 80% replacement without any loss of efficacy.
Take, for example, Palmyra X 004, our top-benchmarked frontier model. This model was trained entirely on synthetic data, and its performance is a powerful testament to the capabilities of synthetic data. Palmyra X 004 excels in several key areas:
- Advanced tool-calling capabilities: Palmyra X 004 can execute functions based on user input and handle multiple tool calls in sequence or parallel. This makes it highly versatile and capable of performing complex tasks.
- Multi-step workflows: The model can trigger no-code Writer apps as microservices, enabling multi-step workflows. This capability is crucial for enterprise AI integration, where tasks often involve multiple steps and systems.
- Built-in graph-based RAG tool: Palmyra X 004 includes a built-in graph-based RAG (Retrieval-augmented generation) tool, which allows for real-time data retrieval from a company’s Knowledge Graph. This ensures that the model can access and use the most up-to-date and relevant information.
- Large context window: With a 128k context window, Palmyra X 004 can handle complex and context-rich tasks, making it suitable for a wide range of enterprise applications.
- Top rankings for tool calling and API selection: Palmyra X 004 has achieved top rankings in benchmarks for tool calling and API selection, further validating its performance and reliability.
The success of Palmyra X 004 in achieving state-of-the-art results without the need for real data training is a clear indication that synthetic data is a powerful and flexible resource for AI training. It challenges the traditional view that real data is indispensable and opens up new possibilities for more accessible, efficient, and scalable AI development.
Myth 4: Synthetic data poses significant risks and ethical concerns
While synthetic data does come with its own set of risks and ethical considerations, these can be effectively managed with the right approach. The concerns often revolve around the potential for performance degradation when synthetic data is mixed with real data, as well as broader issues related to data integrity, privacy, and bias. However, with proper regulations, transparency, and a balanced approach, these risks can be mitigated, ensuring that synthetic data is used responsibly and ethically.
One of the primary concerns with synthetic data is the potential for performance degradation when models recursively train on LLM-produced training data. However, this issue can be addressed through careful and continuous integration. When synthetic data is constantly mixed with new, real data, the model can maintain and even improve its performance. A self-evolving model may be one way to bring in continual learning and new data into the training pipeline (essentially a “self check” system, monitoring for the quality and diversity of its own data).
The key to mitigating the broader risks associated with synthetic data lies in three fundamental principles: regulations, transparency, and a balanced approach.
- Regulations: Adhering to strict regulations is essential to ensure the ethical use of synthetic data. At Writer, we’re committed to following all relevant guidelines and standards, which helps to prevent misuse and ensures that our synthetic data is generated and used in a manner that respects privacy and data integrity. We work with third parties to ensure that we’re mitigating bias (and not amplifying it in the synthetic data we produce). We also have bias detection and correction mechanisms built into models that produce the data.
- Transparency: Transparency in the generation and use of synthetic data is crucial. It allows stakeholders to understand how the data is created, what it represents, and how it’s being used. At Writer, we prioritize transparency by clearly documenting our data-generation processes and making this information available to our users and partners.
- Balanced approach: A balanced approach involves using synthetic data in conjunction with real data, where appropriate. This ensures that the model benefits from the diversity and richness of real data while also leveraging the precision and control of synthetic data. By maintaining this balance, we can address the ethical concerns and risks associated with synthetic data while still reaping its benefits.
At Writer, we’re dedicated to using synthetic data responsibly and ethically. We value primary sources and ensure that our synthetic data is derived from factual and reliable information. This commitment to responsible data practices not only enhances the performance and reliability of our models but also builds trust with our users and the broader community.
Embracing the power and potential of synthetic data
The myths surrounding synthetic data are unfounded and detrimental to AI progress. By understanding and addressing these misconceptions, we can fully harness the potential of synthetic data.
Synthetic data, when used responsibly and verified properly, significantly enhances AI model training. It complements real data, offering precision and control that address many of the limitations of real datasets.
At Writer, our goal is to create a holistic platform for enterprises to easily and affordably transform mission-critical workflows with AI — including models that meet the unique performance and security needs of enterprises. We believe that larger datasets are reaching their limits, and the future lies in precise training approaches and transformer architecture innovations. More companies will adopt synthetic data, recognizing its benefits in performance, cost, and ethics.
We encourage enterprise leaders to embrace synthetic data, knowing it’s a reliable and ethical solution for advancing AI in the enterprise. By integrating synthetic data, businesses can achieve better outcomes and maintain data privacy.