Humans of AI
– 10 min read
Rethinking the hiring process in the AI era with Hilke Schellmann
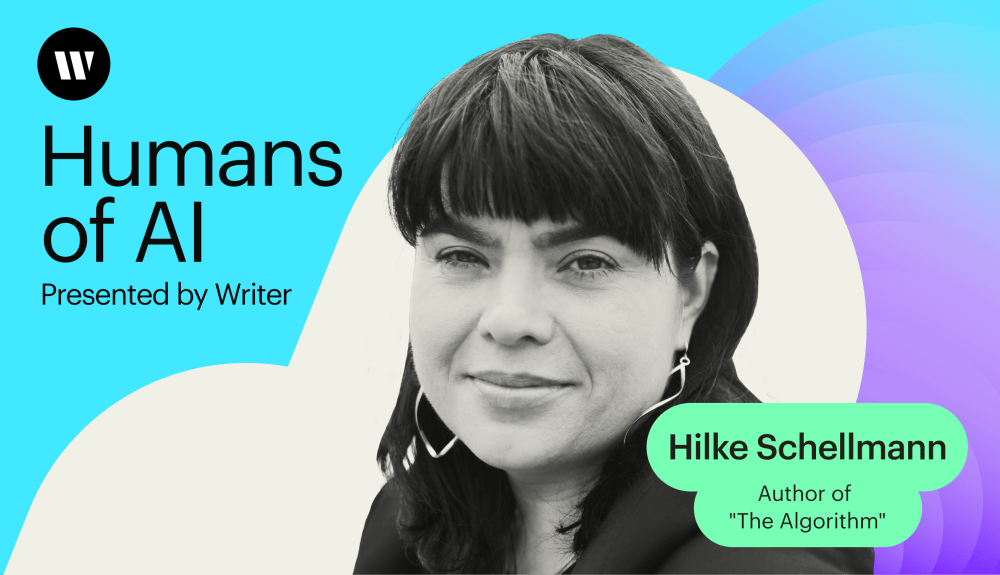
If you’ve participated in a hiring process in recent years — either as a job candidate, as a hiring manger, or as an HR professional, it’s safe to assume you can see where there’s room for improvement. Now that generative AI has entered the scene, is this the opportunity to make the hiring process more efficient and equitable? Or are we running the risk of further spreading systemic biases?
In this installment of Humans of AI, we have a conversation with Hilke Schellmann, an Emmy award-winning journalism professor at NYU and the author of the book The Algorithm: How AI Decides Who Gets Hired, Monitored, Promoted, and Fired and Why We Need to Fight Back Now.
Hilke shares her unexpected journey into tech reporting and discusses the challenges and limitations of AI in the hiring process. She wants HR managers to be more cautious and intentional in their use of AI tools. She also wants vendors to be open about how their algorithms work. And she wants everyone involved in the hiring process, from job candidates to hiring managers to execs, to have informed discussions about what it’ll take to make hiring more efficient and fair.
- Hilke shares her unexpected journey into tech reporting after a chance encounter with a Lyft driver.
- Hilke believes transparency from AI tool vendors is crucial to allow independent assessment and evaluation of their fairness and effectiveness.
- She urges HR managers to approach AI tools with skepticism, testing and scrutinizing them for biases and potential discriminatory effects.
- A human-centric approach to hiring, considering holistic factors beyond resumes, can create a fair and inclusive process for both job seekers and employers.
The crossroads of social justice and tech reporting
Tech reporting was never on Hilke’s agenda. She’d built her career as a journalist covering social justice issues, always drawn to stories that gave a voice to those who often went unheard. She covered a wide range of topics, from sexual assault in Pakistan to student debt and restaurant grading for prestigious publications like Vice on HBO and The Wall Street Journal.
A chance encounter with a Lyft driver in November 2017 shifted Hilke’s path. The driver shared his experience of being interviewed by a “robot” for a job as a baggage handler at a local airport. What he described was likely a conversational AI system employed to pre-screen candidates before moving them forward to have an interview with a human hiring manager.
“The experience had clearly shaken his world. It made him really feel uncertain,” Hilke explains. “And I was surprised, too, because I’d never heard of this. I wanted to know more. I could also tell that this was an emotional experience for the driver, and I felt like I could totally understand that it could be unsettling.”
Hilke’s curiosity was officially piqued. Her journalistic sense told her there was a story about the use of AI in job interviews. She attended an AI accountability conference happening at NYU shortly after. One woman spoke to big data at work, and the basic algorithms at play that had the potential to cause extreme discrimination.
“I was like, ‘Wow, somebody needs to look into this,’” Hilke says. “And I looked around and I noticed there’s not many people here.”
Job platforms have led to an explosion in applications
The rise of job platforms like Indeed, Monster, and LinkedIn revolutionized the job search process, removing barriers to hiring and making it easier for applicants to apply. Hilke notes that this convenience created a new challenge for HR departments: handling an overwhelming number of applications. Companies like Google and IBM receive millions of applications each year, making it impractical for human resources to manually review every resume.
This created an opportunity for vendors to step in and fill the void with digital tools, which also correlated with the development of AI. Now, AI is used across all stages of the hiring process, most notably at the beginning of the funnel where algorithms conduct automatic rejections based on statistical relevance.
Hilke explains what that statistical relevance might look like in action: “Maybe you give an AI tool the resumes of the 50 accountants that currently are in the job. The tool analyzes which keywords are statistically significant. And statistically, if you have this word on your resume, that implies that you will be successful at this company.”
At the outset, matching resumes with statistically significant keywords seems like a pragmatic approach to screening applications. It also removes the human factor, and it’s nearly impossible for humans to act with total objectivity.
“The truth is also that humans are very biased in hiring,” Hilke stresses. “Science is very clear that we have a lot of unconscious bias, so it’s not necessarily always good when humans look at resumes or do job interviews.”
But AI tools can easily misinterpret statistically significant data as meaningful correlations toward job performance — and that still leads to unintended biases.
Biases in AI screening tools and algorithms
Hilke uses an example of the name “Thomas” to illustrate how algorithmic bias works.
Let’s say an AI model trains on examples of resumes of successful employees at a company, and quite a few happened to be named Thomas (one of the most common names for men in the US and England). The AI model may mistakenly interpret the prevalence of the name with the likelihood that the applicant will be successful in a certain job. Without properly informed human intervention, this could perpetuate an algorithmic bias — the model scores anyone named Thomas a higher score in the screening process, and therefore anyone named Thomas has a higher chance of moving forward than someone named, say, Tameka.
“I think [the problem is] us humans trying to connect meaning to any of these findings,” Hilke explains. “We always feel it must be meaningful because the computer found it. Now, if you actually look, there’s no meaning. It’s just some random correlation. There were just a bunch of Thomases in the [training data], but we humans attribute meaning to it.”
Training data inherently reflects the biases of the culture that created it. If marginalized communities are underrepresented in the training data, then the AI system is taught to continue perpetuating these biases. This can create a dangerous cycle where biased inputs lead to biased outputs, as was the case with Amazon’s resume screener a few years ago.
Amazon’s HR department used hundreds and thousands of resumes of people who were currently successful at the company to train the resume screener. The tool predicted that people with the word “woman” or “women” on their resumes weren’t going to be as successful in certain roles.
“I think what we have here is there’s already a gender bias at work,” Hilke says. “… Since Amazon is a tech company, and it probably historically has hired more men into tech jobs than women, that was reflected in the resumes. People with the word ‘women’ were less successful at Amazon. So you have this historical bias that was baked into the AI tool because of the training data.”
Unmasking the veneer of objectivity
AI systems used in hiring often lack transparency, especially when it comes to how they make decisions and rank candidates. While it’s possible to see the training data and the results the models produce, we don’t have a clear understanding of the specific factors that determine a candidate’s ranking. This lack of insight makes it difficult to guarantee fairness and prevent biases from influencing the hiring process.
“I think we as humans don’t think enough about things like, ‘Okay, I built this tool, but how is the tool actually making decisions?’ So we don’t monitor or supervise or fully understand what is happening,” Hilke explains. “Is this method actually a good idea? And what exactly is the tool based on? We don’t ask that enough times.”
The issue of true objectivity comes up once again when companies use expression recognition and vision technology in video interviews. Hilke questions the assumption that all humans have the same set of emotions, and that facial expressions alone can accurately assess a candidate’s suitability for a job.
“It’s really good at reading our facial movements, like ‘mouth is going up’ or ‘forward brow.’ It can sense that and do a readout. But does that mean because I’m smiling, I’m happy? No, it doesn’t. That points to the larger problem that psychologists have now said over and over again, that from a smile or a facial expression, you cannot infer how everyone feels,” Hilke stresses. “We do not feel the same way.”
Hilke isn’t alone in her concern. The recently passed EU AI Act bans “emotion recognition in the workplace and schools, social scoring, predictive policing (when it is based solely on profiling a person or assessing their characteristics), and AI that manipulates human behavior or exploits people’s vulnerabilities.” This landmark legislation will no doubt serve as a regulatory template for other nations such as the US.
Reimagining the hiring process
According to Hilke, traditional methods of hiring — such as relying solely on resumes and conducting one-way video interviews — may not be the most effective way to assess talent. She suggests reimagining the hiring process by adopting a more holistic approach that considers individuals in a 360-degree manner and considers the teams they’ll be joining.
Hilke understands that AI tools are here to stay, which means it’s critical we build tools that not only work effectively, but also eliminate discrimination. She urges HR managers to be more skeptical and actively test these tools, scrutinize technical reports, and understand the assumptions and validation processes behind them.
“You don’t need to spenzd hundreds of thousands of dollars,” Hilke points out. “And then if we had just a little bit more transparency in these tools, I think a lot of civil society groups could step in and actually test these tools. So let’s build our own tools for the public good and put that all in the public domain.”
Hilke acknowledges that the biases that arise from these tools are often unintended consequences rather than deliberate actions. But when we fail to closely monitor these tools and scrutinize them, we’re doing a disservice to job seekers everywhere by continuing to enable historical biases.
“My big hope is that we could have a more educated discussion,” Hilke says. “How should we really hire, which tools should we use, how should we build them? And we shouldn’t just use the old, wrong ways of hiring and now digitize it and put a veneer of objectivity.”
Hilke’s investigation gives us a call to action to work alongside AI to make the future of recruitment and HR something that works for us rather than the other way around. Rather than succumbing to sensationalism or blindly following trends, we should follow Hilke’s example and stay grounded in the human story. Let’s ask questions, experiment, actively participate, and make decisions that are rooted in the reality of our unique circumstances.
Want to hear more stories from the humans working at the crossroads of business and generative AI? Subscribe to Humans of AI wherever you listen to podcasts.