Humans of AI
– 9 min read
Selling AI without the hype
Conveyor’s Daniel Kish on trust and technology
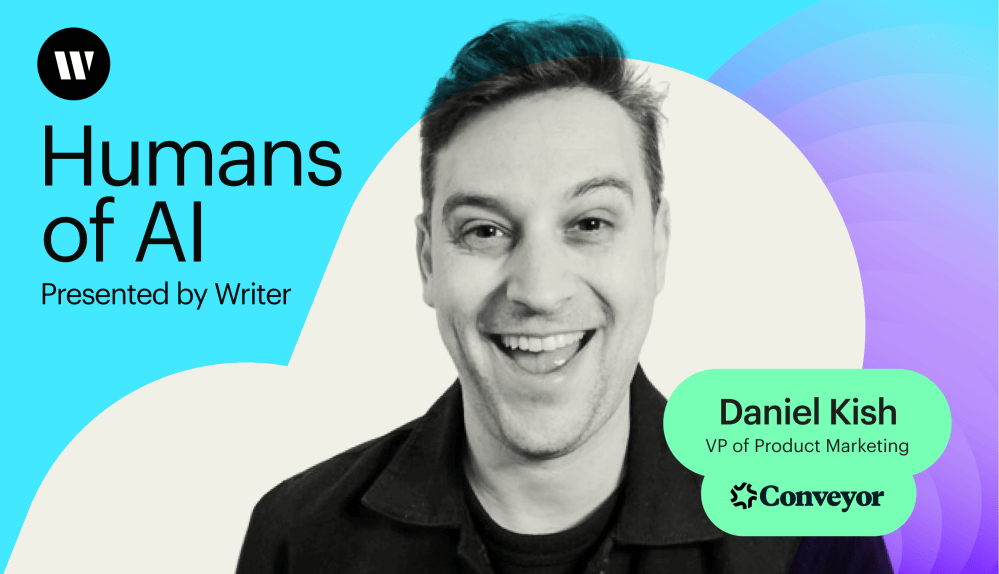
Imagine starting your career with dreams of brewing the perfect beer, only to find yourself spearheading product marketing at a leading AI software company.
That’s Daniel Kish for you, our guest on this week’s episode of Humans of AI. Daniel is VP of Product Marketing at Conveyor, a software company that automates the security review process for vendors. With a career spanning impactful roles at B2B SaaS mainstays like Salesforce and Gong, Daniel’s journey is a testament to the power of problem-solving and adaptability.
His approach to product marketing in the AI era is built on trust and human-centric values. Daniel leads via the player-coach model, diving deep into the details alongside his team to truly understand and address their needs.
- Daniel emphasizes that building trust with businesses in the AI era involves transparency, accountability, and a genuine commitment to solving customer problems.
- He believes that trust should be the number one priority and that it starts with better communication, especially around failures.
- Daniel suggests showcasing the potential of AI by connecting its deployment to measurable business impacts and productivity benefits.
- He recommends a player-coach model in product marketing, where leaders are both part of the team and capable of teaching and coaching others, focusing on solving integrated workflow problems rather than just touting AI’s accuracy.
An atypical path to product marketing
Every successful product marketer starts out as a chemical engineer and then turns to consulting…
Wait, what?
“I thought I was gonna go brew beer,” Daniel says. “That was my big plan. It turned out I was not so good of a chemical engineer, but I was pretty good at the problem solving side of things.”
Daniel jokes about being a “failed engineer,” but it’s this very journey through failure that led him to excel in product marketing. Granted a top-secret security clearance at Booz Allen Hamilton, he worked on cutting-edge technology for the U.S. Special Forces.
(What is it with generative AI leaders having some sort of early experience with the U.S. military?)
Funnily enough, he attributes this unique experience as the foundation for his understanding of the complex needs of clients — a skill he carried into his role in product marketing.
“When you understand the lowest levels of technology, you can talk about them at a high and a low level of value. You start to develop a little bit of a superpower. I wasn’t a good engineer, but I could talk about the technology and I understood it really well.”
He landed a job at Salesforce in the pricing strategy department — an area he says will forever be the best training ground for any product marketer.
“You learn a lot about what is the value of that thing we’re selling, and also what’s the underlying technology that’s driving it,” Daniel says. “How can I talk about it in a unique and differentiated way? And so by doing that I then develop a really good skill set around not just the economics, but the technology in the market.”
He translates those lessons learned into his current role at Conveyor, where his ultimate goal is to build trust in AI.
Earning consumer trust through transparent product marketing
Daniel focuses on the aspect of change management that addresses interdepartmental issues without overwhelming existing structures.
In these situations, Daniel says, the main point is to choose a problem that’s big enough to need attention, but not so big that it needs its own internal team.
“Ultimately for these companies who are looking at cross-functional and alignment problems, what you need is trust,” Daniel explains. “You need to trust the people who are coming in who are saying, ‘We’re not doing this just because we can make a buck, we’re doing it because we actually see this problem all over the place and we finally figured out the solution.’”
Daniel admits that there will always be a financial benefit in this situation, but he just wants to find a way to solve a hard problem for customers who don’t have the resources to do so internally.
To establish trust, Daniel says, you have to hold yourself accountable.
Accountability isn’t just a buzzword for Daniel — it’s a commitment. He sets clear benchmarks for his team and holds them responsible, making sure that every failure is a step towards improvement and that every success is scalable.
Underpromising and overdelivering on the benefits of AI
Before Conveyor, Daniel was the Director of Product Marketing and Pricing Strategy at Gong for three years. At Gong, the focus was squarely on solving core problems related to revenue intelligence through an integrated workflow approach.
Although AI has been integral to their product, Gong chose not to spotlight AI in their initial marketing efforts.
Instead, they let the results speak for themselves. This led to customers often expressing surprise and delight at the AI’s effectiveness, noting how “the AI was really good” without ever being prompted.
By sidestepping the common pitfall of overemphasizing accuracy of AI-generated outputs, Gong focused on real-world applications and encouraged customers to witness the AI’s capabilities firsthand. This strategy built trust in the technology organically.
“Let’s not oversell on accuracy at this point because that’s not what customers are buying. They’re buying a benefit, but we always have to remember it’s about the personal benefit, the personal workflow and the integration of those workloads in order to drive productivity and revenue,” Daniel explains. “Gong really found a way in the early days to unlock the visibility of that workflow. And then talk a little bit more about what made the AI unique as the market matured.”
Daniel doesn’t just talk the talk — he walks the walk by actively demonstrating how AI integrates and thrives in real business environments. This hands-on approach is vital. It allows marketers to highlight the value of AI and build a genuine understanding and trust in the technology.
“I can’t ask somebody to go do that if I haven’t myself actually integrated it in my own workloads,” he says.
Embracing the player-coach model
For Daniel, leadership isn’t just about making decisions from the top. It’s about being in the trenches with your team and knowing first-hand what they’re going through.
The player-coach model is an engaging approach where leaders wear dual hats: that of a strategist and also an active participant.
“What it meant to me was you could never disassociate yourself so far from the work that you couldn’t teach people,” Daniel explains. “If you wanted to hire A players, if you wanted to be around A players, if you wanted to cultivate them, it meant that you needed to teach them. And that was incumbent upon you as a leader to know the skills and to be a master. It also forces you to never get so far removed from the essence of what’s happening on the front lines.”
This approach is exactly what’s needed in the era of AI. It means that when leaders talk about the capabilities and challenges of AI, their words carry the weight of firsthand experience.
“One of the things that we’re really good at learning about with generative AI is it’s not a panacea for every problem,” Daniel says. “But the way that we learn is by failing in a lot of those use cases in order to generate really high intuition and confidence when we pitch a particular set of use cases.”
Escaping “proof of concept purgatory”
Daniel sees enterprises as very willing to experiment and deploy AI. But they linger too long in the experimentation phase, and these solutions fail to scale because they’re not quite sure how to deploy them in a way that’s both safe and effective.
Daniel suggests starting with the business impact first and setting clear and measurable KPIs.
He references an example with a large human capital management software company that implemented a pilot project using retrieval-augmented generation (RAG).
The company zeroed in on key metrics like service level agreement (SLA) turnaround times, aiming to slash them from 10 business days down to just hours. They also kept a close eye on how these changes boosted win rates and sped up deal closures — especially for those big-ticket deals where even a slight increase in speed could mean a multimillion-dollar difference.
AI not only cut down SLA times dramatically but also ramped up deal velocity and win rates, leading to a hefty boost in sales. This clear, measurable success was just what they needed to build confidence and scale up the deployment.
It’s a great example of how honing in on the right metrics can showcase AI’s ability to revolutionize business operations, turning even minor improvements into major gains.
“The meat of it really is the same — no matter if you’re deploying AI or if you’re solving cost optimization somewhere,” Daniel explains. “We always connect it to the business impact. We then connect it back to that North Star of where the company needs to go, and we finally make sure that we organize the field in such a way that they feel like this is working to their productivity benefit. It shouldn’t feel like we’re just shoving in a new technology for the sake of it. It has to really have an internal feeling of ‘This is making me better.’”
Daniel’s journey teaches us that moving past the trough of disillusionment in AI adoption requires more than just technological innovation. It demands a rethinking of leadership roles and a deeper engagement with the problems and pains at the front lines of business.
It’s about being a player-coach, someone who is as much a part of the team as they are a leader.
Want to hear more stories from the humans working at the crossroads of business and generative AI? Subscribe to Humans of AI wherever you listen to podcasts.