Thought leadership
– 5 min read
Navigating the challenges of generative AI and “vendor lock-in” in enterprises
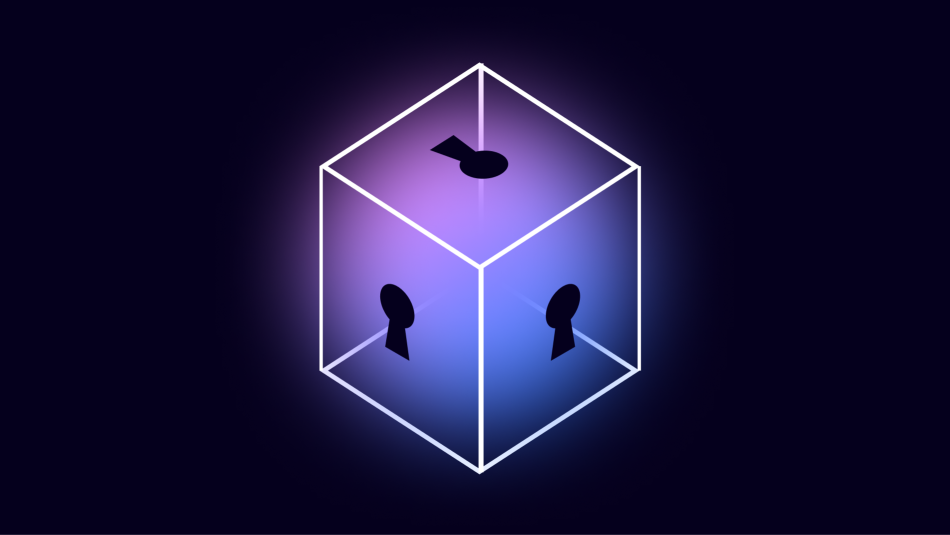
“Vendor lock-in” in the enterprise is a big issue, but generative AI turns the traditional conversation on its head. Vendor lock-in refers to the dependency on a single vendor, making it difficult to switch providers without significant costs or disruptions.
While enterprises traditionally concern themselves with vendor lock-in, that issue becomes complicated in the context of generative AI. It’s harder to find a direct equivalent to previous technology waves — such as choosing SAML (Security Assertion Markup Language) over Active Directory or opting for a hybrid cloud instead of committing to a single cloud provider — in generative AI.
That’s because there’s almost no such thing as an LLM-agnostic application. By definition, you’re “locked-in.” You can’t build an app where you can easily swap one model for another with no re-work. This deep integration means that any transition involves significant redevelopment, not just simple plug-and-play. Continuous rewrites to accommodate LLM updates might be manageable for a few apps or use cases — but this approach is hard to scale and a major reason why so few companies have hundreds of apps or use cases in production.
In a world where enterprises are spreading around their generative AI budgets, building self-reliance is more important than the sisyphean effort to prevent “lock-in.”
Defining self-reliance in enterprise generative AI
Enterprises achieve self-reliance when they can develop and sustain their own generative AI programs independently from external vendors. Your proprietary assets as an enterprise are the golden four components necessary for building a self-reliant generative AI program.
Use cases and business logic
Your use cases and business logic will determine the specific applications and processes generative AI will improve in your organization. By mapping generative AI use cases, enterprises can customize their AI solutions to address their unique business requirements. Business logic sets the operational rules for AI within these scenarios, aligning AI outputs with the company’s goals and standards.
Data and examples
Proprietary data and examples are the training and refinement ground for generative AI models, enhancing model accuracy, contextual understanding, and adaptability. Using high-quality LLM training data allows enterprises to tailor their AI models to their specific industry needs. This improves precision and reliability while also allowing for the customization necessary to reduce bias and ensure equitable outputs.
In-house talent
You need in-house talent that can build, iterate, scale, and maintain these apps. Internal expertise allows the organization to create and adapt AI solutions without relying heavily on external vendors, thereby reducing dependency. In-house talent makes sure that the enterprise can manage and update AI models, integrate them with business logic, and handle data effectively.
Organizational capacity for learning and change
One of the main barriers to AI adoption is cultural resistance. Your organization needs to have the capacity to learn and change if you want to effectively adapt to these new technologies and methodologies. This capacity ensures that the organization can continuously update and refine its AI applications, integrate new data and insights, and respond to evolving business needs.
Choosing the right partners
Enterprises should be trying to choose vendors who can truly partner to help build the golden four — partners that don’t look at an enterprise as just another API key. It’s important that these partners provide a scalable platform and a no-code environment that supports easy updates and ensures backward compatibility of AI models. Evaluate generative AI LLM vendors based on these criteria:
- Technical architecture
- Data privacy and compliance
- Customization and integration
- Security and risk management
- Transparency and accountability
The right partnership is a collaborative relationship rather than a mere service provider role.
Building a self-reliant generative AI strategy with Writer
When selecting a vendor for generative AI, Writer stands out as a leader with its comprehensive, full-stack approach tailored to enterprise needs. Our integrated platform combines Palmyra LLMs, graph-based RAG, and customizable AI guardrails with tools that cater to both technical and business users. This approach ensures enterprises can deploy AI applications that are not only efficient and scalable but also deliver transformative ROI by addressing complex use cases with precision.
Furthermore, Writer understands that technology adoption is as much about people as it is about tools. With dedicated change management support and a focus on cultural transformation, Writer ensures organizations not only implement AI solutions but also fully integrate them into their operations. This commitment to driving adoption and long-term success makes Writer an unparalleled partner for enterprises embarking on their AI journey.
It’s utterly meaningless to have no “vendor lock-in” on LLMs — because it all falls down anyway if you change the LLM — and your only path to self-reliance is to have the above. What enterprises should instead be focused on is choosing partners who can help them build scalable generative AI programs. Schedule a demo with Writer to form a strategic partnership and start building your internal capabilities.