AI in the enterprise
– 9 min read
How enterprise companies can map generative AI use cases for fast, safe implementation
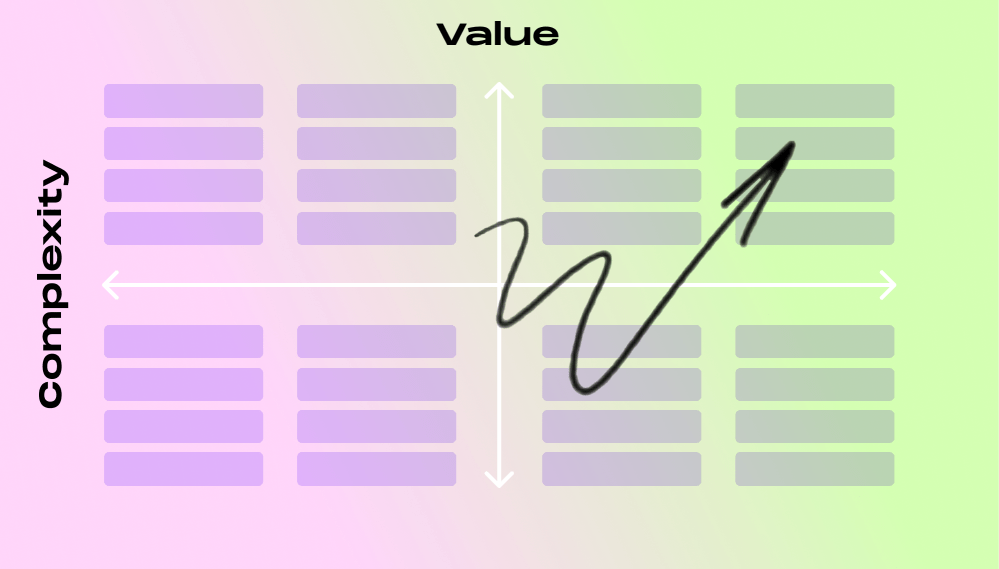
Every IT team is feeling the pressure of the spoken (or unspoken) question: how will our company use generative AI technology? Whether the question is coming from above or below, IT teams know that they have to put a plan in place to show — at a minimum — that they’re thinking about AI in a strategic way.
Due to the breakneck pace of innovation, companies can’t afford to take a “wait and see” approach. Putting off an investment in generative AI for even a year means lost gains in productivity and the ability to grow and market your product. It’s time to move beyond analysis paralysis and put AI plans into action.
While enterprise companies understand the need, they often don’t know how to get started and what tools will best serve them. AI use case mapping allows companies to quickly identify how AI can benefit an organization and provide measurable value, and then rapidly iterate on the findings.
- IT teams know they have to put a plan in place to show they’re thinking about AI in a strategic way.
- AI use case mapping allows companies to quickly identify how AI can benefit an organization.
- The use cases should align with an organization’s goals and overall objectives.
- The emerging generative AI tech landscape encompasses everything from foundation models to apps and full-stack platforms.
What’s AI use case mapping?
AI use case mapping looks at a specific use case within an organization and identifies the potential value of introducing AI. The use cases should align with an organization’s goals and overall objectives.
For some use cases, AI might be solving a problem, such as reducing the manual work employees are doing. In other cases, it might be an opportunity to provide a better customer experience.
You’re not adding AI for the sake of keeping current with technology: with AI use case mapping, you’ve identified a specific problem or opportunity that AI can solve.
What makes a strong AI use case?
AI use case mapping has two dimensions: value and complexity.
Value is the specific outcome for your organization. When you’re calculating the potential ROI, you have to consider how many people will be impacted within the organization. An AI use case that only improves productivity for a few people doesn’t have the same value as a use case that impacts hundreds. You can also consider time saved: an AI use case that saves time on low-value tasks frees up talented employees to perform more high-value work.
You also need to have good underlying data and assumptions to model out the projected ROI — which can sometimes be challenging.
Complexity examines the technical requirements to implement AI. Your organization may have products in place with AI capabilities that aren’t being used, or it may require adding something new to your tech stack. Complexity also examines the need for integrations or third-party products.
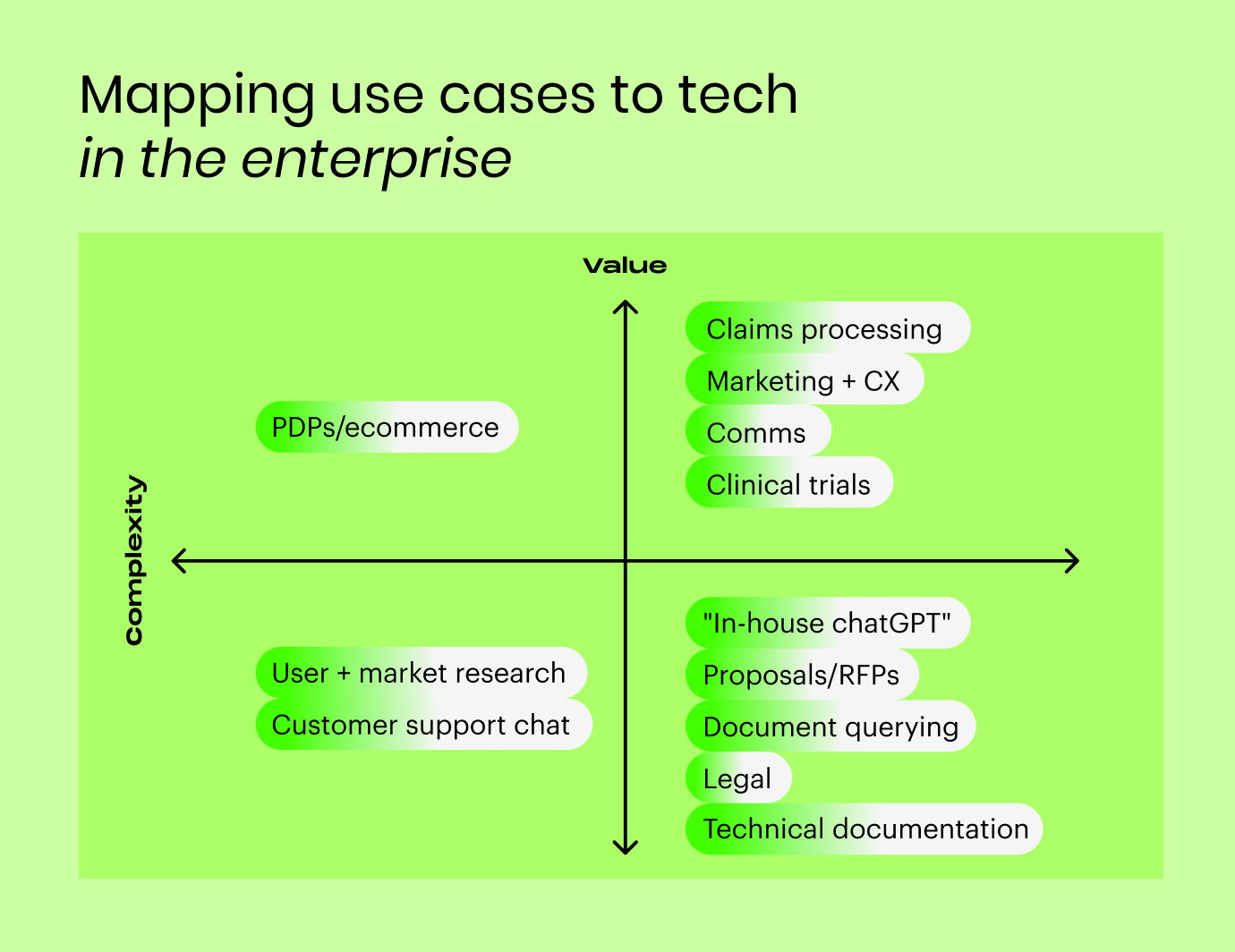
An organization’s readiness to adopt AI is often dependent on existing solutions. An organization with, say, an advanced personalization product has a high level of readiness for AI adoption, which will make AI use cases easier to implement. By contrast, organizations using legacy products or disparate systems face bigger challenges.
Organizations also need to consider people-readiness. If AI is integrated into a workflow, will people adopt it? If people aren’t ready, introducing AI will not bring as much value to the company.
Strong initial use cases should be high value, low complexity.
Determine the right solution for AI use cases
At the intersection of value and complexity, an organization can begin to hone in on a specific use case for AI. This should be done with input from the business unit leaders who’d be implementing the proposed solution.
The next step is to determine the right type of AI solution since AI can come in many forms. While text-based generative AI has captured the world’s attention, there’s also machine learning, retrieval/search, speech synthesis, image generation, and many other forms. Plus, not all generative AI solutions are equal. The emerging generative AI tech landscape encompasses everything from foundation models to apps and full-stack platforms.
The solution will depend on the output formats your organization needs. Business unit leaders should think about how AI fits into the potential workflow and the end result (A report? A Word document? Slides?) Involving the right people at this stage of the process will ensure that a chosen solution meets quality and accuracy expectations, as well as privacy, security, and compliance requirements.
Demonstrate the potential of AI with a pilot project
With an AI use case identified, you need to map it to a potential solution as a pilot project. This should not be a lengthy process, as you’re only trying to “prove out” the use case’s feasibility and expected results. Nor should you try to map out dozens of use cases with a potential solution. Select one or two that’ll have a high impact on the organization without being overly complex.
This is a strategic process that allows your organization to quickly visualize a future state. Decision-makers and end-users should be able to clearly understand the outcome. It’s a manageable way to get started.
From there, your organization can work on an execution plan:
- What will it take to put these use cases into production?
- What are the next steps?
- How do we prioritize the next use cases?
Speed is the name of the game. Don’t spend more than a few weeks mapping your AI use cases. You might try out using a virtual agent for internal information retrieval or creating a template for specialized content generation. If you dig in too deep, you’re delaying implementation and putting more at risk that your chosen solution may not solve the problem. You need to verify that you’re on the right path so you can move on to the next steps: implementing AI and reducing your time to value.
Adore Me (now acquired by Victoria’s Secret) moved quickly when it mapped out its initial AI use case. The company started with writing product descriptions: highly structured output that was, time-consuming work for human copywriters. Adore Me had existing product description examples to use for language model training, so it wasn’t a technically complex project.
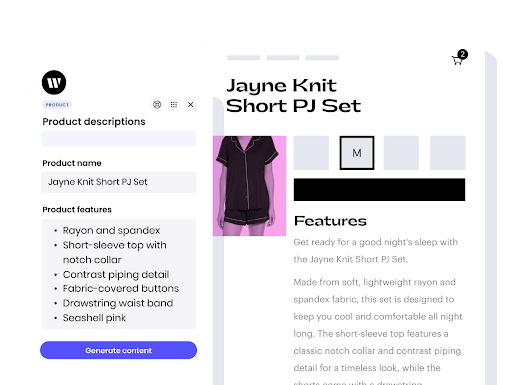
Adore Me proved the success of the pilot project by measuring the conversion rate of AI-generated product descriptions against those written by human copywriters. The conversion rate was unaffected. The company cut the time involved by up to 50% and freed up human employees for more high-value, creative work.
Operationalize AI and adopt an agile strategy
Once the chosen technology is in place, your organization can rapidly move to adopting other use cases.
But we’re not talking about a three- or five-year roadmap. That type of strategy isn’t sufficient with a technology that changes so fast. Nor can you spend time just deciding on the use cases to implement — because you’ll never get there. AI involves continuous learning and taking advantage of the latest technology and features.
An agile strategy moves quickly. You don’t develop a long-term plan and stick to it. Even if your plan for rollout is 18 months, you should have numerous checkpoints along the way.
Your plan serves as a guide that will get you to your future state, but you iterate through your use cases. You’re constantly evaluating the next steps and making adjustments. With AI, in particular, you’ll need to account for changes as the technology continues to evolve and your chosen solutions add new functionality.
At Perficient, we’ve developed a P.A.C.E. framework for operationalizing AI. It’s a holistic approach that allows companies to realize the benefits of AI while also mitigating risks.
- Policies: Create corporate guidelines and boundaries for AI usage
- Advocacy: Promote the understanding and adoption of AI
- Controls: Implement oversight, auditing, and risk management
- Enablement: Provide tools, resources, and support systems
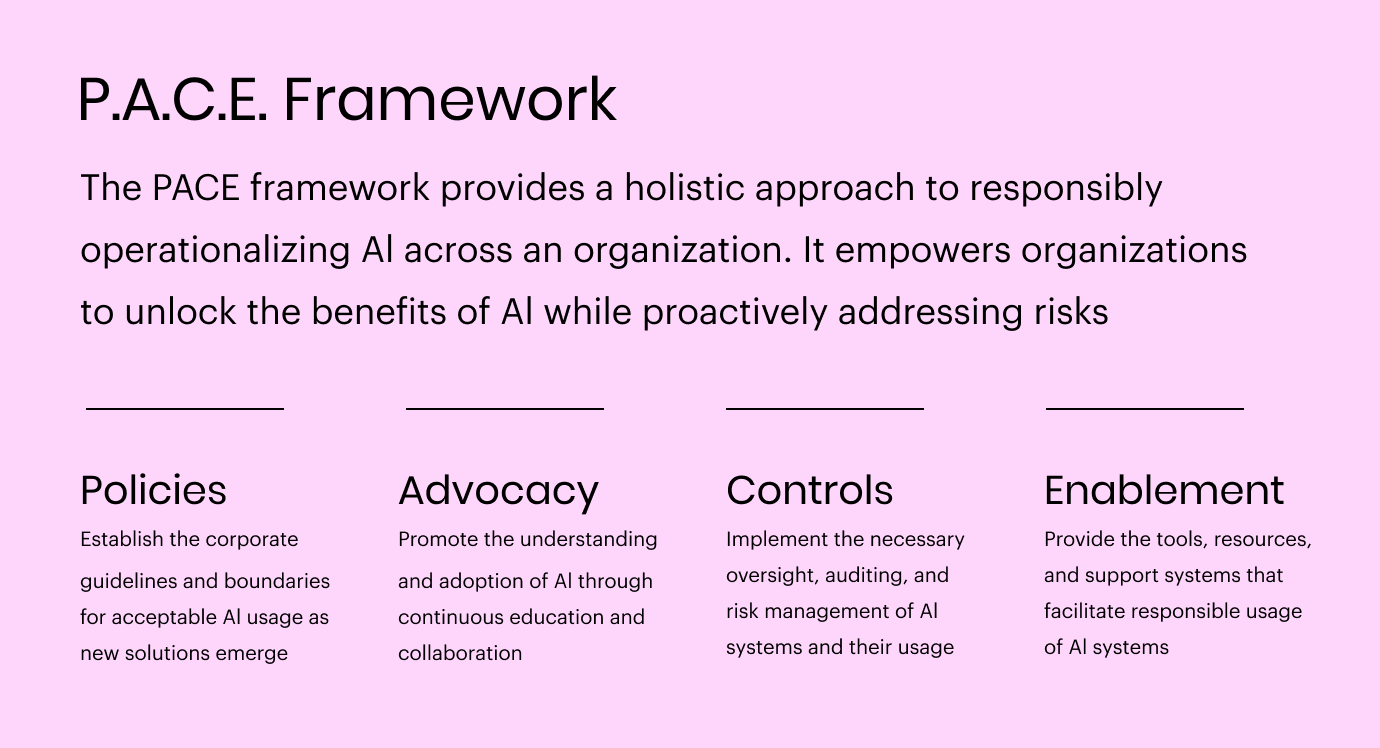
Operationalization is critical as AI is rolled out to additional teams. The systems you put in place will help people along the way, making sure each team is as well-supported as the initial team that adopted your AI use cases.
Keep pace with AI’s curve
While you can’t afford to wait with AI adoption, you also need to understand how AI is continuing to evolve.
You need to be slightly removed from the bleeding edge of technology. You might implement a solution only to find that something better is coming down the pike. Or your vendor plans to replace a feature with something better and it breaks the systems you’ve put in place so it’s important to understand a product’s roadmap.
By investing in scalable technology and operationalizing your AI adoption, you can move at a pace that allows you to quickly and safely see ROI. Work in short cycles, allow each mapped use case to prove its value, and then move on to the next.
Discover more about enterprise generative AI use cases. Read our enterprise AI use case guide.
Brian Flanagan is a Digital Experience Strategist at Perficient. In this role, he serves as a trusted advisor to Perficient’s clients and is responsible for developing digital strategies that drive business results. With over 25 years of experience, Brian has a deep understanding of the digital landscape and a proven track record of delivering innovative and engaging customer experiences. He is known to stay ahead of the curve and is consistently on the lookout for opportunities to enhance the customer experience through next-generation technologies.