AI in the enterprise
– 14 min read
How to evaluate LLM and generative AI vendors for enterprise solutions
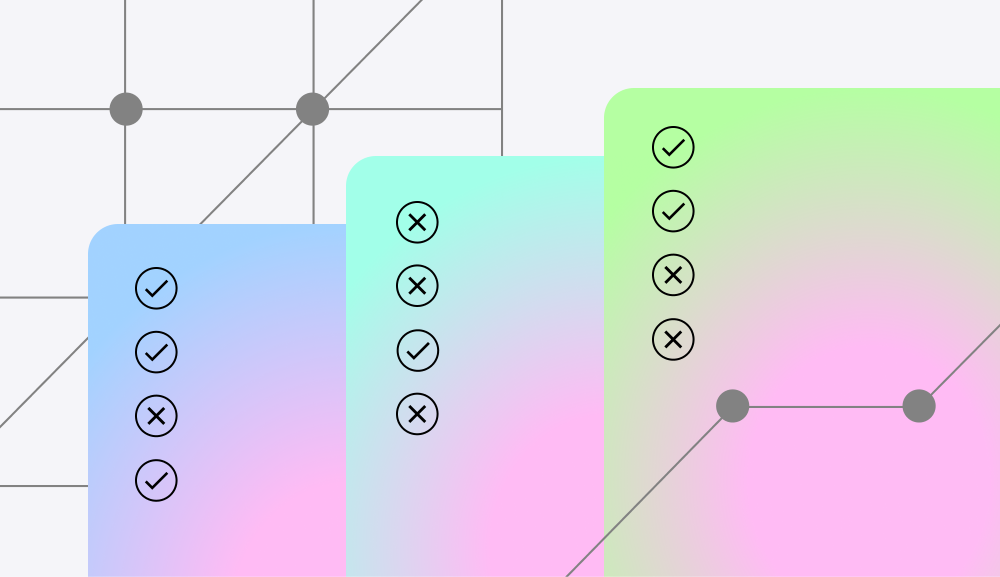
Most of the c-suite execs we work with have already ideated generative AI use cases. Some have even created sandbox environments and started to prototype. But now the hype is calming down and we’re hearing that folks need to innovate real generative AI solutions, show meaningful value, and start to scale. This is a whole ‘nother ball game.
The process can feel like navigating a mountain of governance work without a clear owner. And let’s not forget the task of accurately scoping the overall costs of running generative AI applications at scale. It’s a complex endeavor that requires careful consideration.
At Writer, we’ve had the privilege of working closely with our incredible enterprise customers, learning valuable insights about what it truly takes to succeed with generative AI at an enterprise scale. During our interactions, these customers posed thought-provoking questions to us as they evaluated Writer against other large language models (LLMs) and generative AI approaches.
Now, we’re sharing the knowledge we’ve gained and providing guidance on how to evaluate LLM and generative AI vendors for enterprise solutions.
We’ll dive deep into the key considerations, challenges, and best practices that’ll empower you to make informed decisions. Whether you’re just starting your explorations or looking to enhance existing initiatives, this guide will equip you with the insights you need to drive successful outcomes. So, let’s embark on this journey together and discover the path to evaluating LLM and generative AI vendors for enterprise solutions.
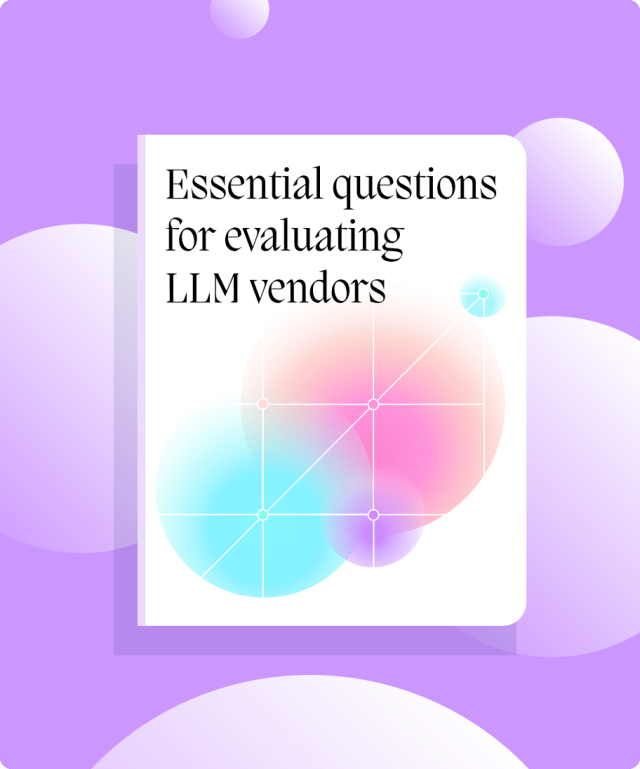
Download the checklist
- Technical Architecture: Understand the vendor’s foundational technology, deployment options, and infrastructure support to ensure scalability, control, and data security.
- Data Privacy and Compliance: Evaluate how the vendor handles data separation, anonymization, and compliance with privacy regulations to protect sensitive information.
- Customization and Integration: Look for vendors that offer customization options and seamless integration with existing systems, allowing you to tailor the solution to your organization’s needs.
- Security and Risk Management: Prioritize vendors with strong authentication methods, adherence to industry standards, access controls, and measures to prevent malicious activities.
- Transparency and Accountability: Seek vendors that provide insights into their decision-making processes, offer monitoring and reporting capabilities, and comply with legal and regulatory requirements.
Investigate technical architecture and deployment
It’s crucial to delve into the foundational technology that powers LLMs and generative AI platforms. You need to understand whether a vendor relies on open-source models, employs a wrapper approach, or has developed proprietary technology. This knowledge will help you assess their capabilities and potential limitations.
- Open-source models: Generative AI foundation models that are publicly available and can be accessed, modified, and used by anyone to develop their own AI solutions.
- Wrappers around another company’s LLM: Wrappers are software components or interfaces that provide a layer of integration and compatibility between a third-party LLM and the user’s own system or application.
- Home-grown, proprietary LLMs: Generative AI language models that are developed in-house by an organization, using their own resources and expertise.
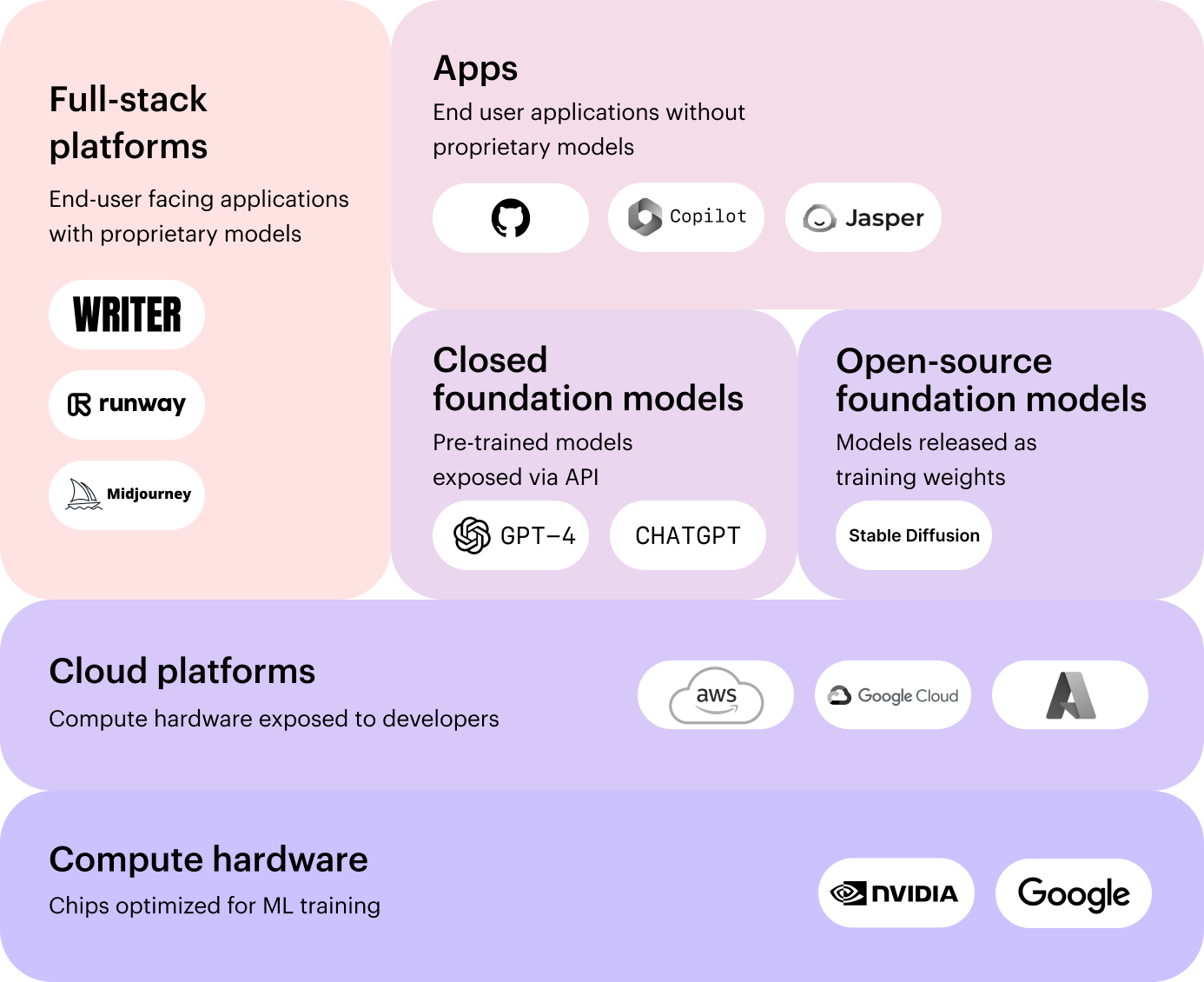
Consider vendor deployment options. Are they single-tenant or private cloud? This knowledge is essential as it affects scalability, control, and the ability to tailor the solution to your organization’s needs.
Don’t overlook the infrastructure needs. Assess if the vendor provides robust infrastructure support and if self-hosting is an option.
Evaluate how the vendor handles data separation, ensuring that sensitive information is protected and processed securely. Look for features like redaction capabilities to maintain compliance with privacy regulations and protect your organization’s sensitive data.
Questions to ask:
- What is the foundational technology behind your LLMs—open-source, wrapper, or proprietary?
- Is deployment available in a single-tenant or private cloud?
- What computing resources are required for deployment? What services do you offer to aid in self-hosting?
- How do you manage data separation and secure processing?
- Can your product integrate with multiple or client-provided LLMs?
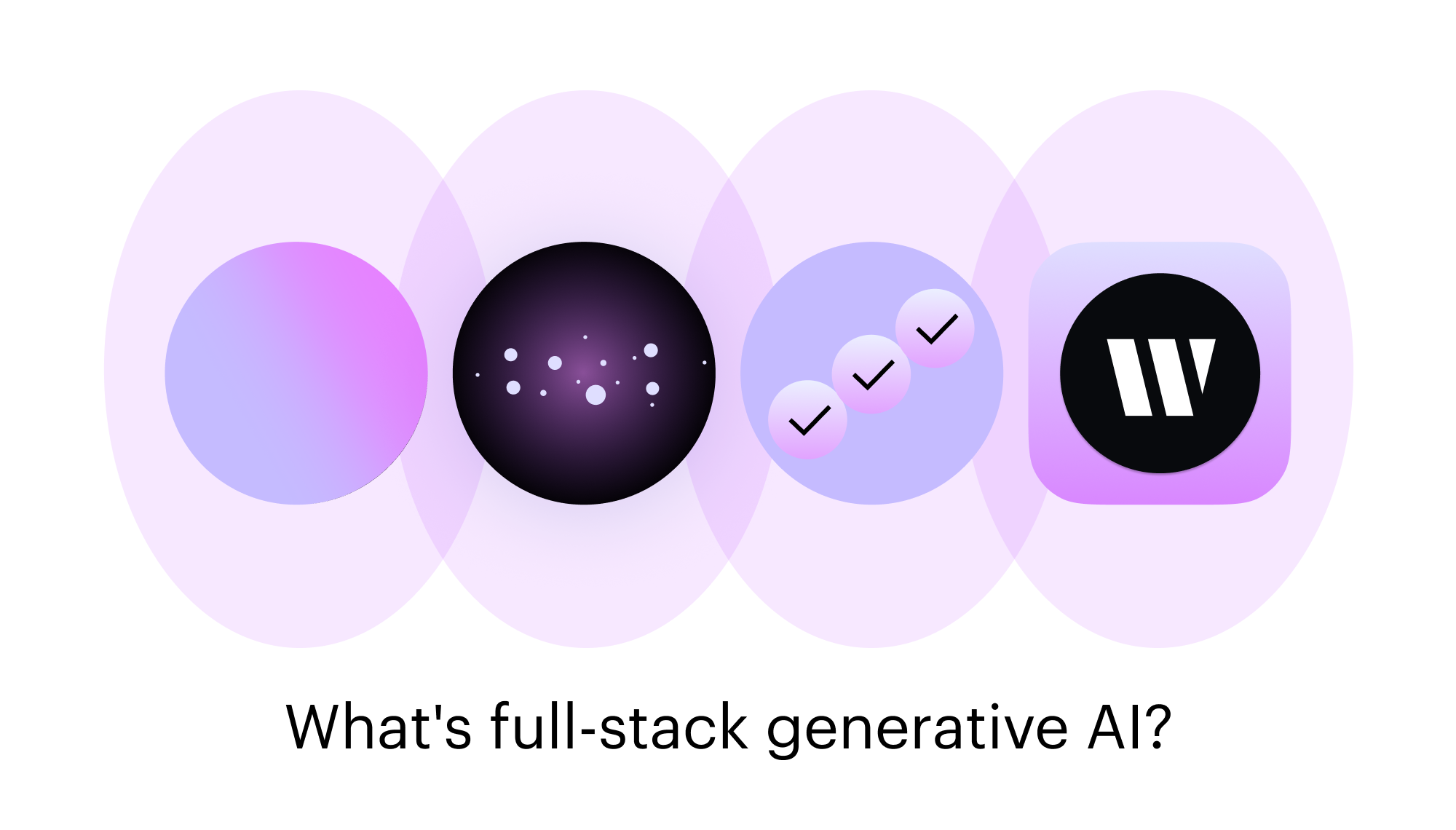
Learn more: What’s full-stack generative AI?
Ask about data lifecycle management
When it comes to managing the lifecycle of your data, understanding the sources and how they shape the foundation model is key. You want to make sure that the vendor you choose aligns with your data requirements and follows best practices.
Data privacy is a top concern. Find generative AI vendors who separate customer data and have strict safeguards to protect sensitive information, including data anonymization, consent management, and compliance with data protection regulations.
Companies should be clear on what data is owned by whom and when hands change.
Questions to ask:
- Are security controls in place to prevent customer data from informing the broader model?
- How are companies planning on using the data that’s sent to the LLM for use case purposes?
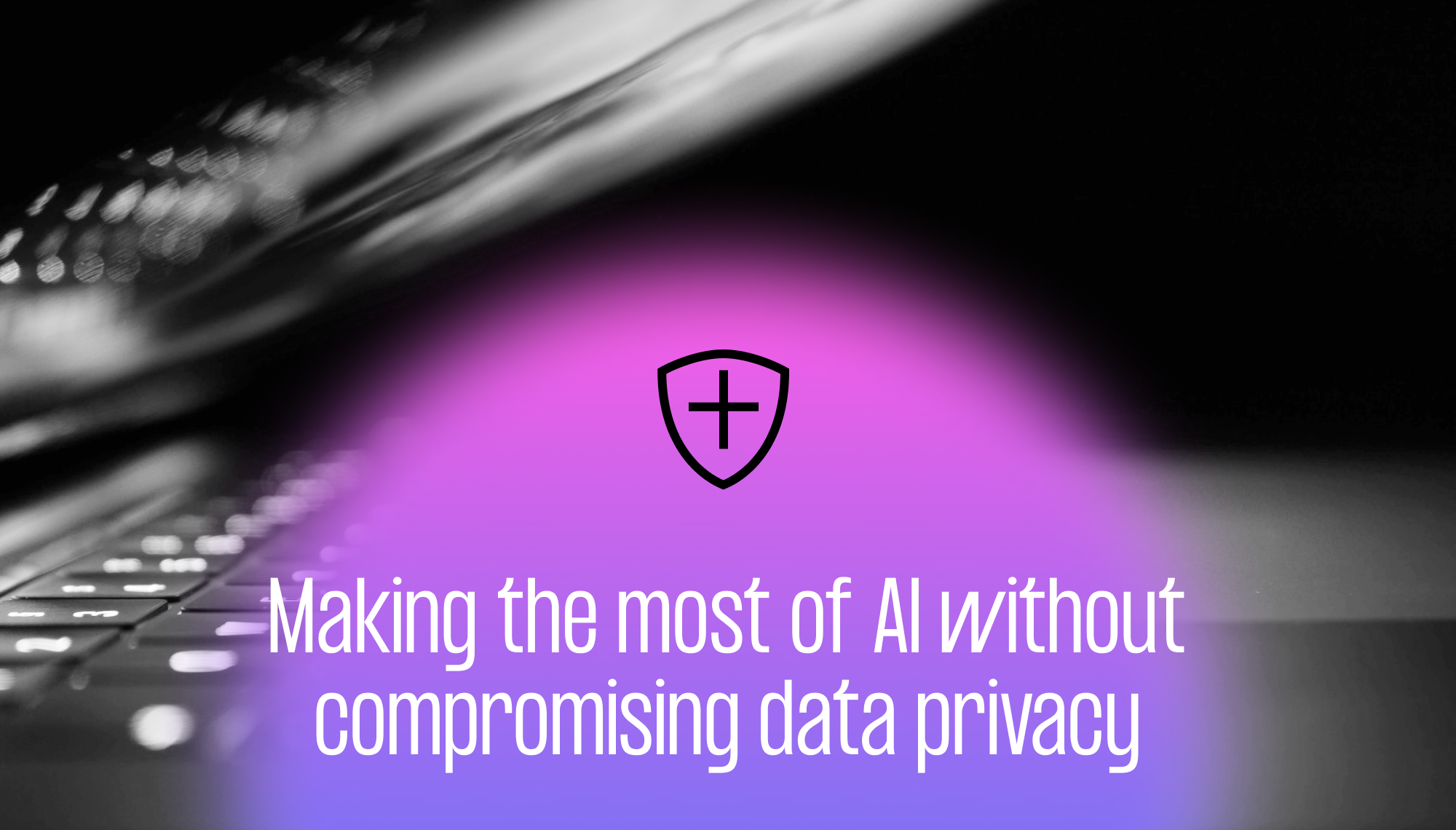
Learn more: Making the most of AI without compromising data privacy
Explore customization and integration features
Look for a vendor with customization and integration flexibility, and a smooth integration process with your existing systems.
The ability to customize the generative AI models is like having a tailor who can create bespoke solutions for your business challenges. Seek a vendor that allows you to fine-tune AI algorithms using your proprietary datasets, enabling you to surface valuable insights that directly impact your operations.
In addition to customization, integration is key to maximizing generative AI’s benefits. Look for a vendor that understands the importance of integrating their solution with your existing infrastructure and workflows.
Consider the vendor’s compatibility with third-party services and applications commonly used in your industry. This enables you to integrate generative AI seamlessly into your existing tools and systems, enhancing productivity and collaboration across your organization.
By prioritizing customization and integration features, you can select a generative AI solution that aligns perfectly with your organization’s needs and seamlessly integrates into your operations. It’s all about finding a vendor that offers the right balance of customization, integration, and ease of implementation to unlock the full potential of generative AI for your business.
Questions to ask:
- Can proprietary datasets be used to fine-tune your LLM?
- Which resources get deployed within a client’s private cloud during implementation?
- Does your product seamlessly integrate with third-party services or applications commonly used in enterprises?
Learn more: Explore the Writer API
Look into enterprise-grade security features
Choosing a generative AI solution that prioritizes robust security measures is crucial. Here are some key considerations to keep in mind when evaluating generative AI vendors:
- Look for strong authentication methods to ensure only authorized users access the generative AI platform.
- Check if the vendor adheres to important regulations such as SOC2 Type II, HIPAA, GDPR, and PCI. You want a vendor that takes compliance seriously and follows the necessary protocols to protect your sensitive data.
- Access controls and ownership of foundation models are important factors to consider. Single Sign-On (SSO) is a convenient feature to look for.
- A reliable generative AI vendor will have measures to prevent malicious actors from injecting harmful prompts or code.
When it comes to enterprise security, it’s all about finding a vendor that values the security of your data as much as you do, providing you with peace of mind in your AI endeavors.
Questions to ask:
- What authentication methods do you support?
- Is your product in compliance with standards like SOC2 Type II, HIPAA, GDPR, and PCI?
- Who has access to the foundation models?
- Do you support single sign-on capabilities? Do you support SCIM?
- What measures are in place to prevent malicious actors from injecting harmful prompts or code?
- What safeguards and monitoring mechanisms are in place to identify and mitigate jailbreak attempts?
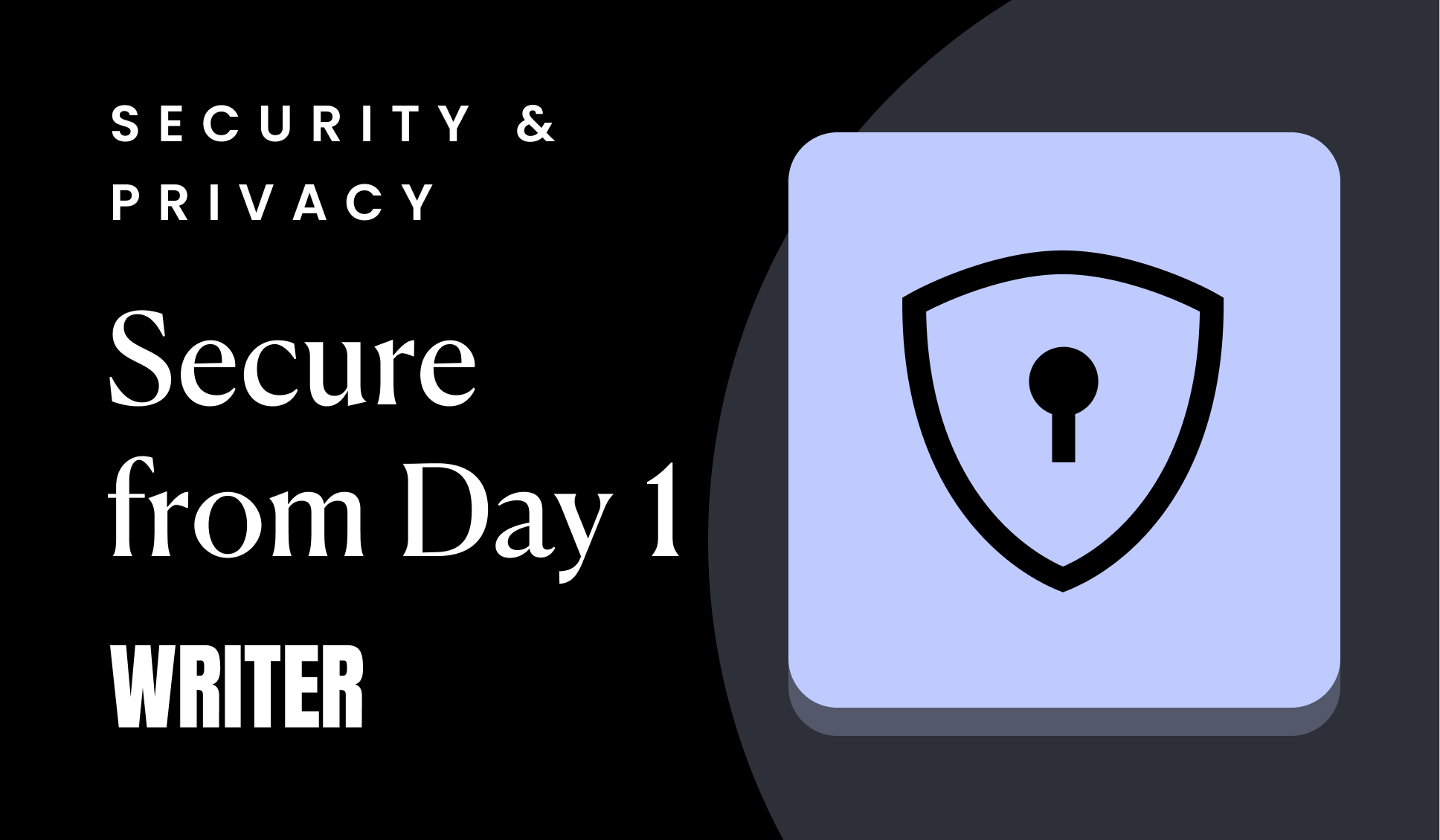
Learn more: Discover how Writer approaches privacy and security
What about LLM output compliance?
Ensuring the content generated by an LLM is free from bias and toxicity is paramount.
First, seek out vendors with robust anti-bias and toxicity mechanisms. Industry standards, benchmarks, or thresholds for toxicity detection are crucial. Look for vendors who have established guidelines to identify and filter out toxic content.
Transparency is key when it comes to understanding the sources of bias data and the methodologies used for training and fine-tuning AI models. A responsible vendor will provide insights into their process.
Consider the availability of automated post-output filtering and measures to ensure diversity in the generated content. A vendor that offers tools to filter and enhance the output shows their dedication to providing a wide range of perspectives and avoiding content that may perpetuate misinformation and biases.
Questions to ask:
- What mechanisms are in place to mitigate bias and inappropriate content?
- Do you have any industry standards, benchmarks or thresholds you adhere to for toxicity detection? How are these updated and refined over time?
- What are the sources of bias data, and what mechanisms and methodologies are used for training and fine-tuning the AI model to manage bias?
- Is there any automated post-output filtering we should be aware of?
- How do you ensure the diversity of the LLM’s generated output?
- Are there other types of content or policies that can be implemented to identify and warn/block inappropriate or harmful content?
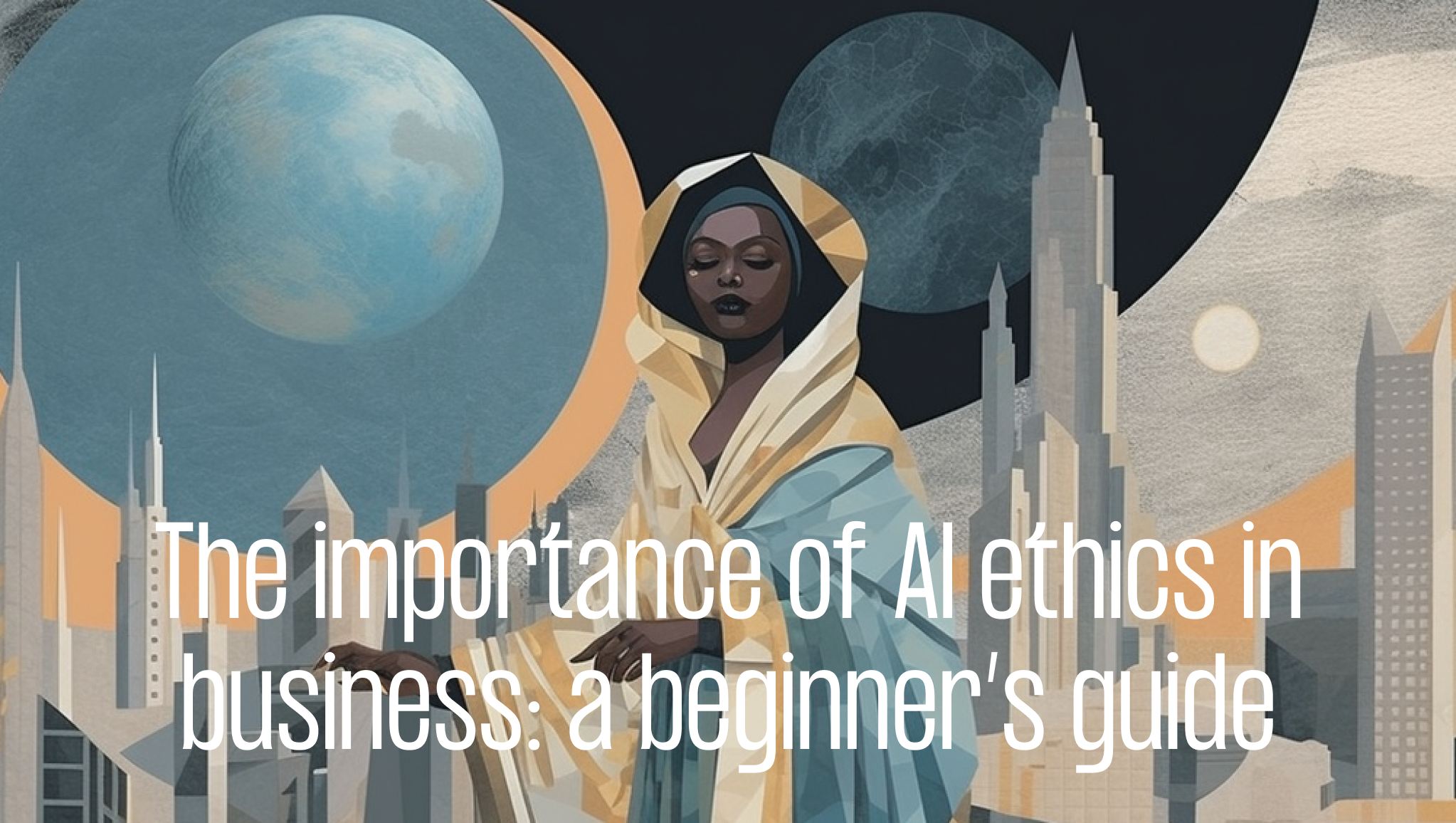
Learn more: The importance of AI ethics in business: a beginner’s guide
Address legal and regulatory compliance
Make sure the vendors you choose for your enterprise solutions meet legal and regulatory requirements. Here are some key considerations:
- Address intellectual property rights and data ownership. Understanding who owns the generated content and ensuring it matches your organization’s policies is vital for a successful partnership.
- Consider the vendor’s ability to prevent certain data from being input. Look for mechanisms to filter out sensitive or confidential information, to comply with privacy regulations and to safeguard your data.
- Be aware of any legal actions against the generative AI vendor. Research their history and assess risks.
- Explore third-party audits or certifications related to bias detection, toxicity detection, personally identifiable information (PII) handling, and security. Look for vendors who have undergone external assessments to validate their compliance with industry standards and regulations.
By carefully evaluating the legal and regulatory compliance of LLM and generative AI vendors, you can establish a secure and legally sound partnership that aligns with your organization’s requirements, protecting your data and intellectual property.
Questions to ask:
- How do you ensure that generated outputs do not infringe on third-party intellectual property rights?
- Who retains ownership of the data inputs and generated outputs?
- Is there functionality to prevent certain kinds of data from being input?
- Are there any ongoing, past, or threatened legal actions against your product?
- Have your models undergone independent, third-party reviews such as HELM, NYC Bias Audit, or EU AI Act readiness? Provide any third-party audits or certifications related to bias detection, toxicity detection, PII handling, and security.
- What compliance standards, such as GDPR, are followed for PII protection?
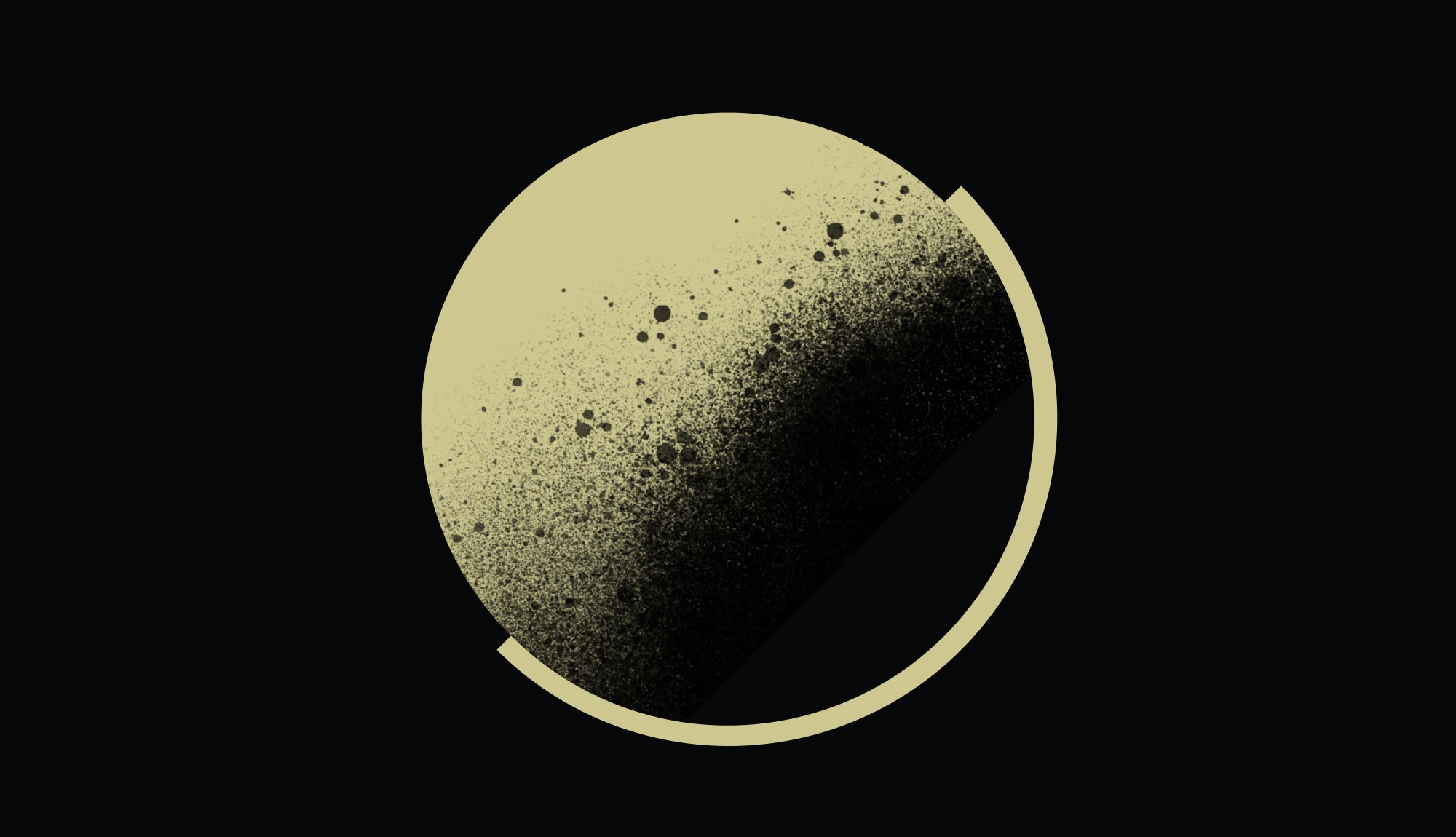
Learn more: Generative AI risks and countermeasures for businesses
Consider scalability and performance
Scalability and performance are critical considerations when evaluating LLM and generative AI vendors for enterprise solutions. To ensure smooth operations with large datasets, look for vendors with robust infrastructure and systems capable of efficiently processing and generating content at scale. They should handle increasing data volumes without compromising performance or quality.
In high-demand scenarios, it’s crucial to assess how vendors mitigate AI hallucinations and inaccuracies. Look for vendors with mechanisms in place to detect and address these issues, ensuring that the generated output remains reliable and coherent. This helps maintain the integrity of the content and prevents the generation of nonsensical or inaccurate information.
Human oversight and reviews play a vital role in maintaining accuracy, relevance, and quality. While AI models are essential for content generation, human input and expertise are invaluable. Seek vendors who support a strong human review process so it’s easy for human editors and reviewers work alongside AI models to refine and enhance the generated content. This ensures a balance between AI automation and human oversight, resulting in high-quality output.
Questions to ask:
- How does your solution handle scalability and performance when dealing with large datasets?
- How do you handle high demand scenarios?
- How do you address hallucinations? Does the platform have human oversight and reviews in place?
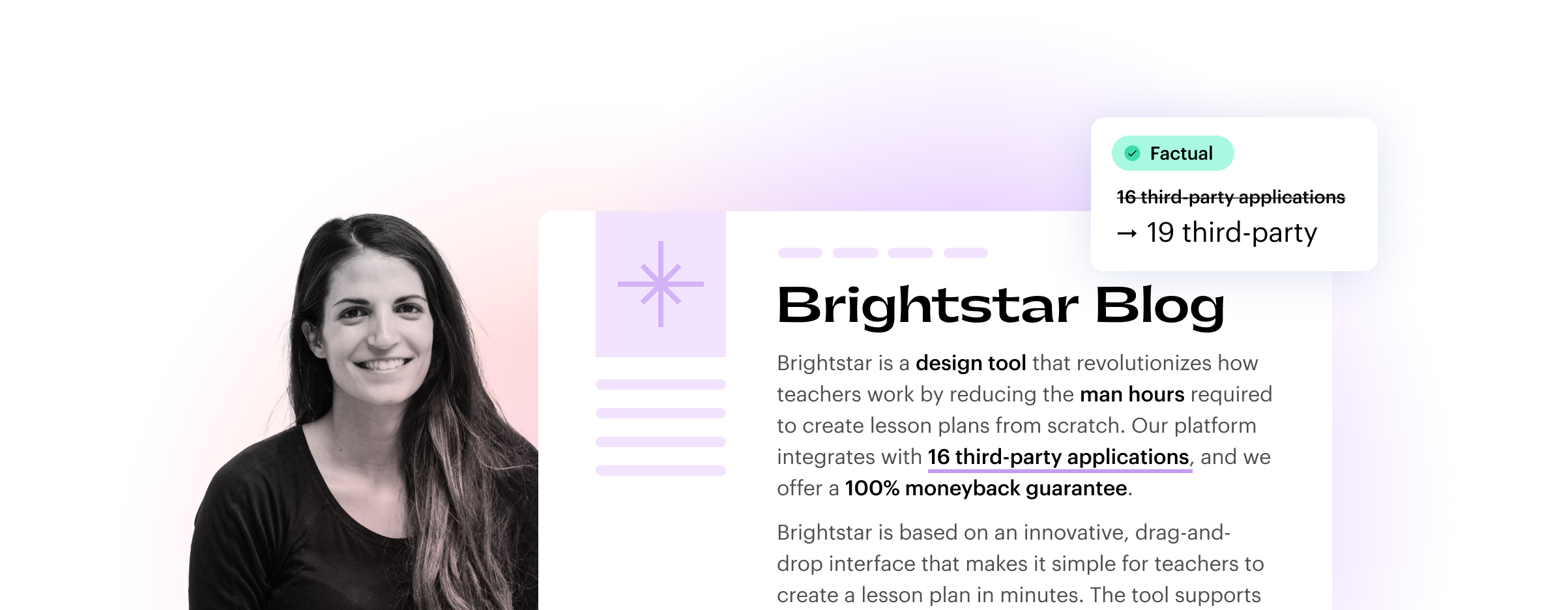
Learn more: How Writer helps companies enforce rules, accuracy, and consistency — at scale
Review monitoring and reporting capabilities
You need AI monitoring and reporting to be transparent and accountable when choosing an LLM and generative AI vendor for enterprise solutions.
To begin, consider the transparency and explainability of the decision-making process in AI models. Look for vendors who provide insights into the underlying algorithms and factors considered, allowing you to understand and validate the reasoning behind the generated content.
Next, assess the visibility of telemetry and security events in the generative AI platform. Access to data that provides insights into performance, usage, and security allows you to monitor and analyze the behavior of the models, ensuring compliance and identifying potential issues or vulnerabilities.
Lastly, explore the types of reports available to assess the effectiveness, accuracy, and user feedback of generative AI models. Look for vendors who provide comprehensive reports highlighting key metrics such as content quality, user satisfaction, and model performance. These reports enable you to evaluate the impact and value of the AI models, making informed decisions about their usage within your enterprise.
Questions to ask:
- What tools and features are available to provide insights into the decision-making process of the AI model? How does your software address concerns related to opaque AI systems, including explainability and transparency?
- What level of visibility is provided for telemetry and security events, and how can this data be accessed?
- What types of reports can be generated to assess the effectiveness and accuracy of controls?
- What reports can be generated to provide insight into the accuracy of generated outputs?
- How do you collect and respond to user feedback?
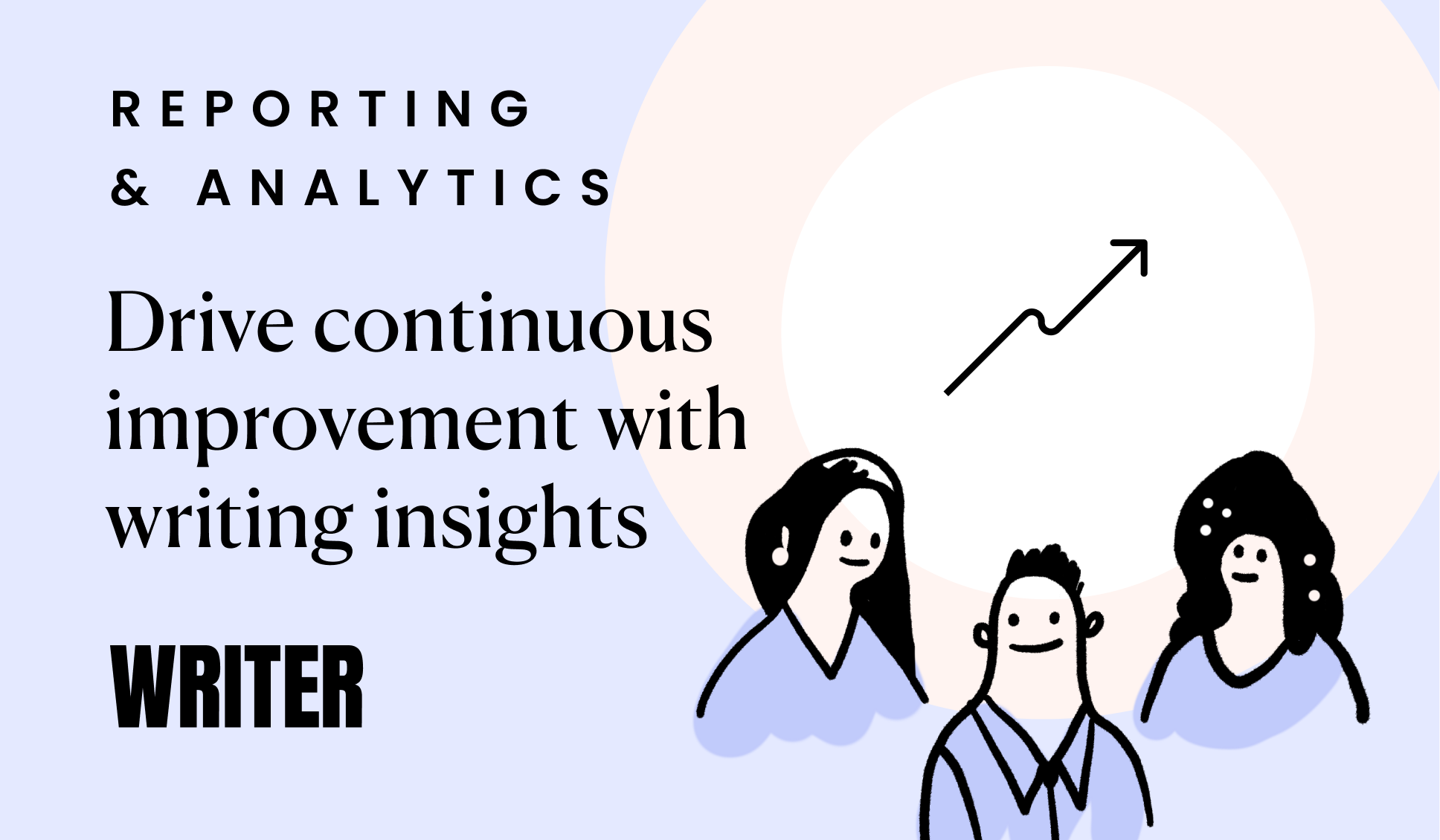
Clarify costs and financial considerations
Financial and operational aspects are major factors when choosing an LLM or generative AI vendor. Some key considerations include costs, support, and customization.
Assess any associated costs for fine-tuning or customization of the generative AI models. Understand the pricing structure and determine if it aligns with your budget and expected return on investment.
Using AI is a huge cultural shift. It requires a significant change management effort. It’s not enough to just throw technology at the problem. Consider the availability of support and training for your personnel. Look for vendors who provide comprehensive support, documentation, and training resources to ensure a smooth integration and ongoing operational success.
Explore the possibility of disabling certain generative AI features at an enterprise level. Ensure the vendor offers the necessary controls and options to align with your operational needs and compliance standards.
Questions to ask:
- Are there extra costs for fine-tuning or customization?
- What types of support and training are available to personnel?
- Is it possible to disable certain generative AI features at an enterprise level?
Learn more: Check out Writer pricing plans (or reach out to our sales team for a custom quote)
Ask the right questions, find the right vendor
As enterprise leaders, it’s essential to make informed decisions when selecting vendors for your AI solutions. Research and evaluate different vendors, considering their track record, reputation, and customer reviews. Engage in thorough discussions with potential vendors, asking relevant questions and seeking clarity on any concerns or requirements specific to your organization.
We’ve create a vendor evaluation checklist of questions to help you get started.
By following these guidelines, you can confidently choose a generative AI vendor that not only meets your business needs but also aligns with your ethical standards and long-term objectives. Remember, the right vendor partnership can unlock the full potential of generative AI and drive innovation within your enterprise solutions.
Do you have questions about Writer? Reach out to our sales team. We’ll give you the answers — and insights — you need.