AI in the enterprise
– 10 min read
Build vs. buy: What’s the best solution for your enterprise generative AI program?
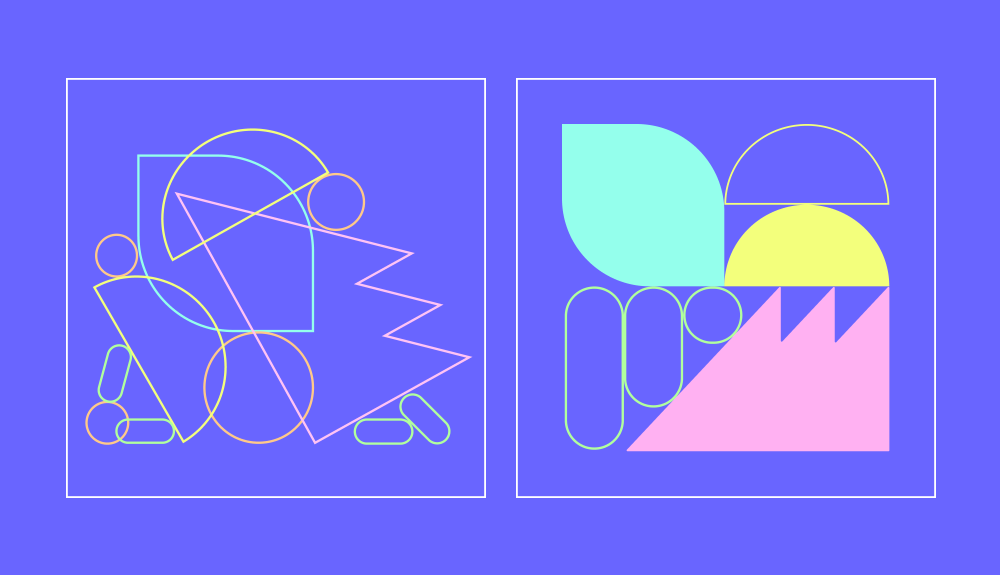
Any enterprise company looking to add a new tool to its tech stack has a lot of considerations. It’s not as simple as “Sign Up Now!” when you’re considering use cases across multiple departments, the underlying technology, and security and/or compliance requirements.
This often begs the question: should we, as a company, buy an enterprise solution to meet our needs, or build our own? As complicated as this classic question is for implementing project management software or an enterprise CRM, generative AI solutions are more nuanced and might feel even more overwhelming.
In my strategy work as a Director of Data Solutions at Perficient, I help clients find the right approach for their business needs — including AI solutions. “Build versus buy” is often a conversation driven by several departments, including executives, a development or technical team, and department leads who understand specific business requirements.
If your company is contemplating building a generative AI solution versus buying a product, you have to think beyond the output. Building involves input as well, in terms of time and expertise. The tradeoff is a solution fully tailored to your needs, but it still comes with quantifiable costs.
Because generative AI is new and rapidly evolving, you may not know your options. You have to dig in to understand what’s available (if you buy) and what’s involved (if you build).
- If you’re contemplating building a generative AI solution versus buying a product, you have to think beyond the output.
- You must look at what you’d need to invest to either build or buy.
- Building and buying will have associated “hard” costs, such as licensing of the necessary components, and staffing.
Why consider building and why consider buying
In my opinion, if your company does business like 80% of the rest of the world, then ideally, you can find a generative AI solution to buy. A smaller percentage of companies sit at the periphery, where you may still be able to buy a product and customize it or integrate it with other products to fit your needs. Everybody has a finance team, marketing team, HR team — and the use cases are fairly consistent, even if there are variations based on the size of the company or industry.
If your company finds that your use cases are very distinct from your peers — and you can successfully build solutions — then you can support those unique use cases. However, you’ll also need to think about long-term maintenance. It’s not a “one-and-done” with building. You’ll have to address bugs and develop new features your team may need over time. You’ll also have to source talent, assuming you don’t already have internal staff with the necessary expertise.
By contrast, buying a solution is easier and faster. It doesn’t rely on your technical resources to piece together the necessary components. That’s not to say there’s zero need for internal resources. You may have an AI program manager or another role to support the configuration and rollout of generative AI use cases.
The right vendor partner can guide your internal resources with best practices for your use cases — provided that the vendor has experience with enterprise companies. Ideally, the vendor has specific knowledge of your industry and its requirements. Without that, your AI program manager will be spending a lot more time trying to figure things out on their own.
How to think about the total cost of ownership
Building and buying both have a mix of costs and resources. Building your solution may save on product licensing fees but carries higher internal resource costs. With buying, it’s the opposite ratio.
The two approaches won’t sum to the same total cost of ownership — and those ratios will vary by organization — but you have to look at what you’d need to invest to either build or buy.
Technical requirements
Nothing is built entirely from scratch. A production-ready generative AI solution could have ten to fifteen different applications, services, or technologies that need to be pieced together, from the cloud environment to the large language model (LLM) to integrations with your other apps. Because the AI ecosystem is very new, you’d need to research the best components to build an application that’ll meet your organization’s long-term needs. And you should expect these answers to change as the AI ecosystem continues to evolve.
When you buy, you’ll rely on the LLM and application built by your chosen vendor. You’ll want to consider safeguards for your data and how your data will be hosted.
In both cases, you’ll also need to consider your industry’s privacy and compliance requirements. Your internal team would need to implement those, or your vendor would need to meet the requirements.
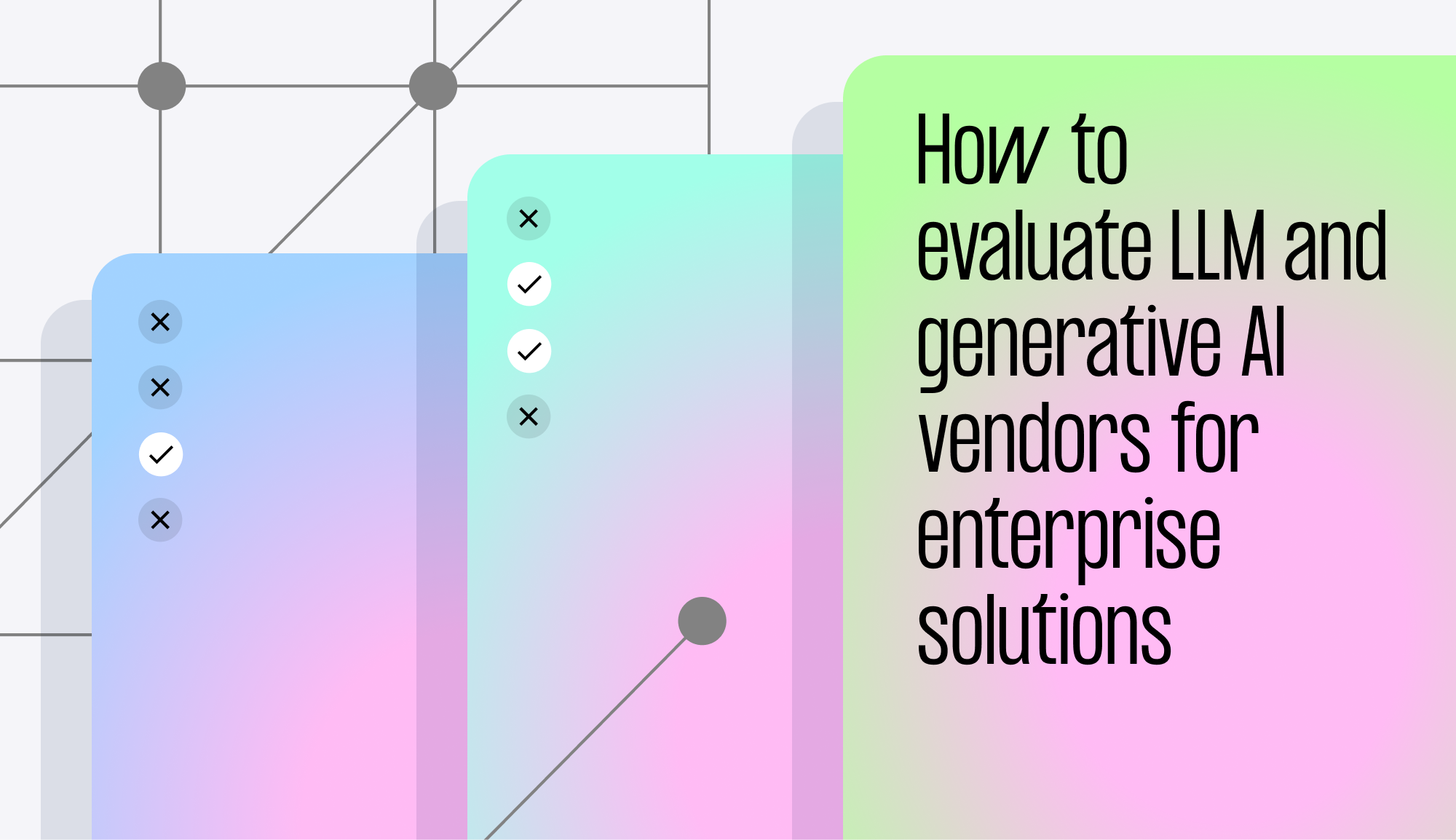
Learn more: How to evaluate LLM and generative AI vendors for enterprise solutions
Resource requirements
When you build, you’ll need resources to develop an application, data engineers to collect and shape input data, and data scientists to fine-tune the LLM. But it’s also a question of, “Which business unit is owning the development?” Some organizations try to tackle this as solely an IT team project, when it’s a product team that’s better equipped to outline the use cases and how employees would interact with the app.
When you buy, your resources will be focused on change management and configuration. It might still span multiple departments but will be considerably less resource-intensive.
Both building and buying will have associated hard costs. Building will have the cloud environment, licensing of the necessary components, and staffing. With buying, you’re spending to purchase access to the product, which could be initial license fees, subscription fees, or both.
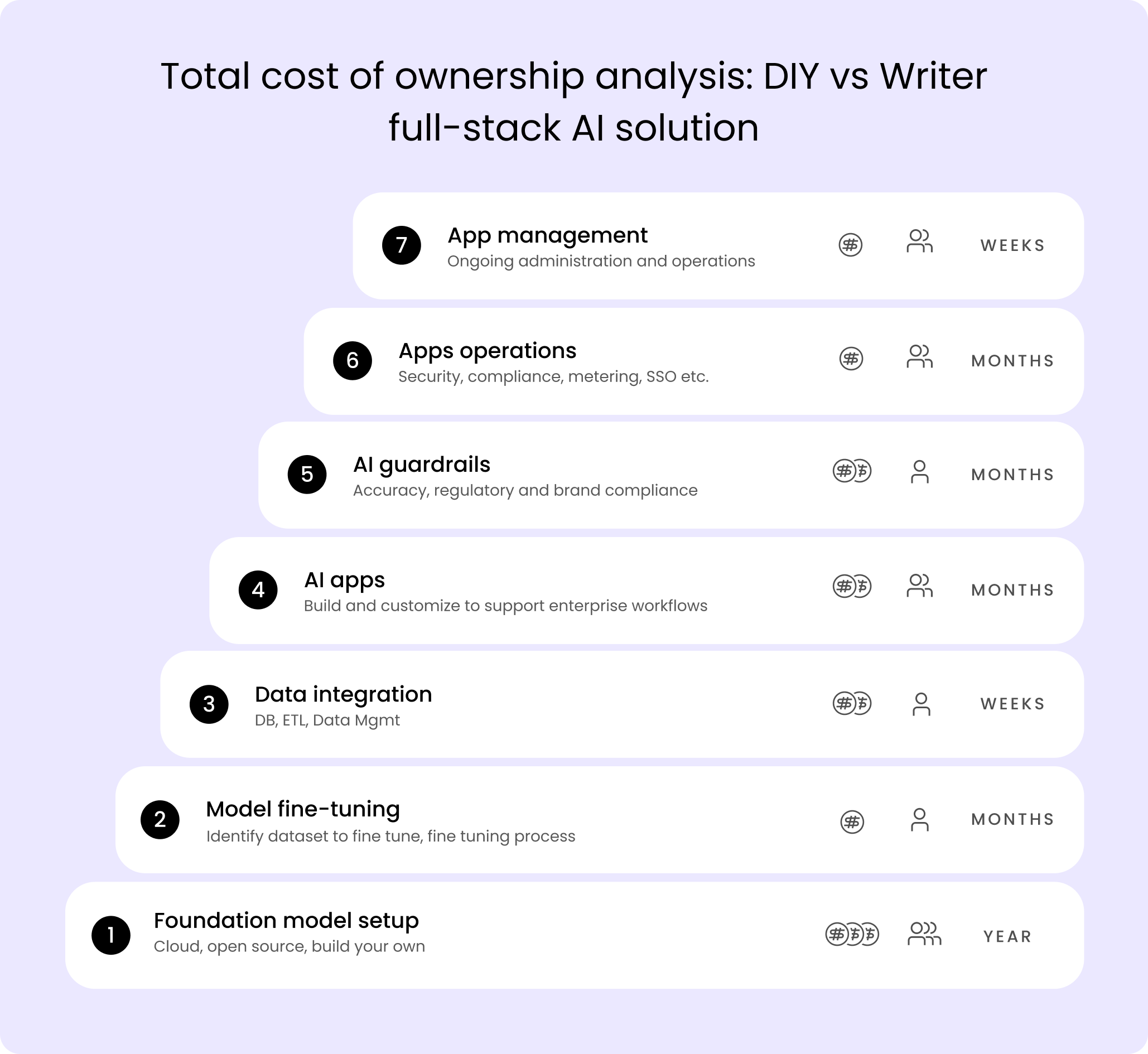
Time requirements
The hardest component to measure in the equation is time, but it’s an important factor. Building will take you time and, in many cases, a lot of time. If you’re going it alone, it can take months to build a production-ready application and up to a year or more to develop a foundation set up, depending on your LLM.
You could be up and running in a matter of weeks when you buy a product — particularly if you’ve done the internal work to determine some initial use cases.
As a full-stack generative AI solution, WRITER can be customized to your organization’s needs and workflows, all while keeping your data safe and secure.
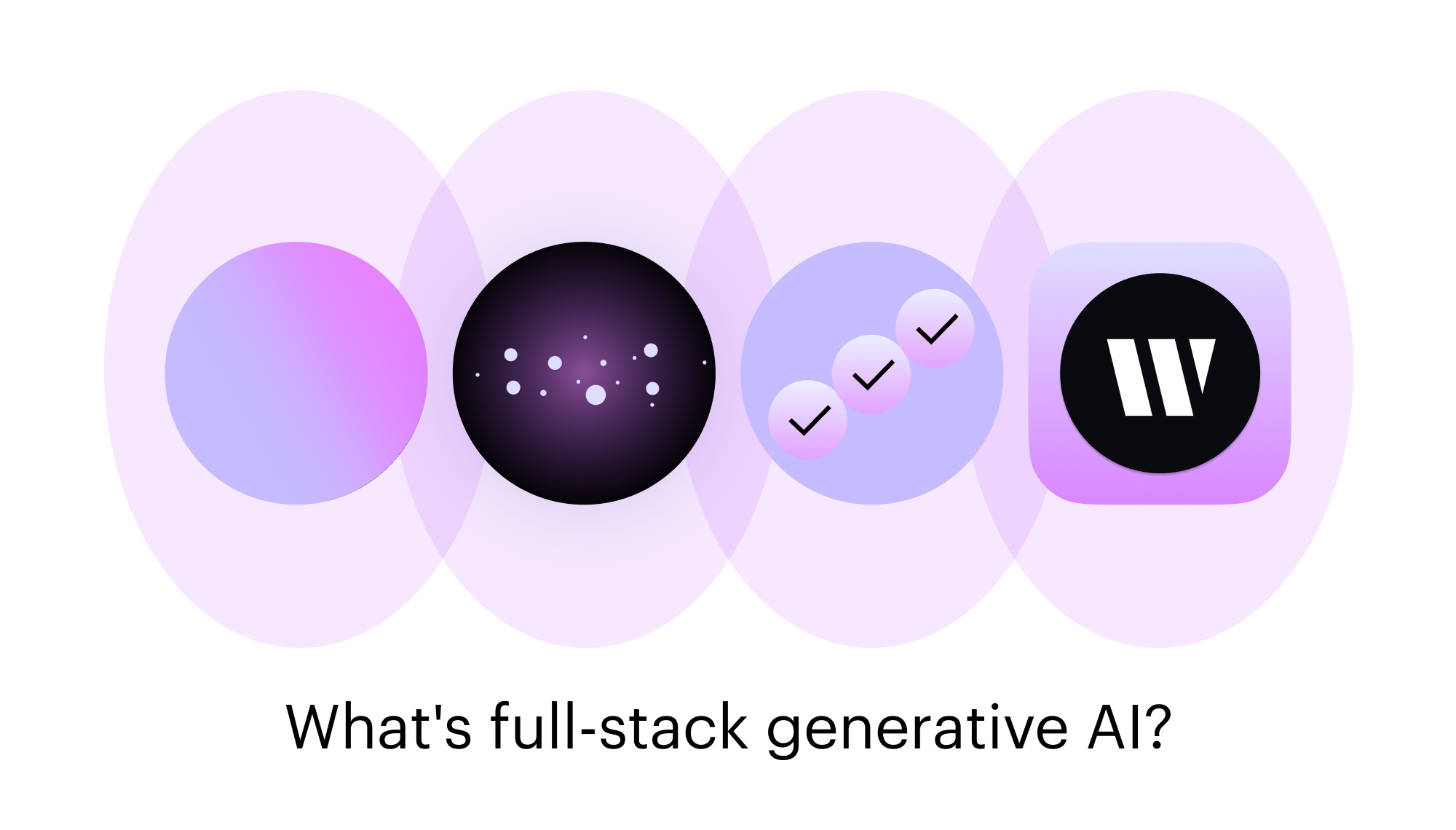
Learn more: What’s full-stack generative AI?
A product needs to support the rapidly changing world of AI
Generative AI is moving very fast. If you build, you’ll need to stay current with rapidly changing technology, which may mean making more frequent modifications than you might with other custom solutions.
The legal landscape is undetermined as well. In the US, there are several pending copyright lawsuits that might impact the data LLMs can use. The White House also recently issued guidance around the responsible use of AI. Though the full impacts have yet to play out, an internally-built product may need to follow the guidance, including security standards and consumer protection.
If you buy, any vendor you select would also need to adhere to the regulatory landscape and be well-equipped to handle changes in the future.
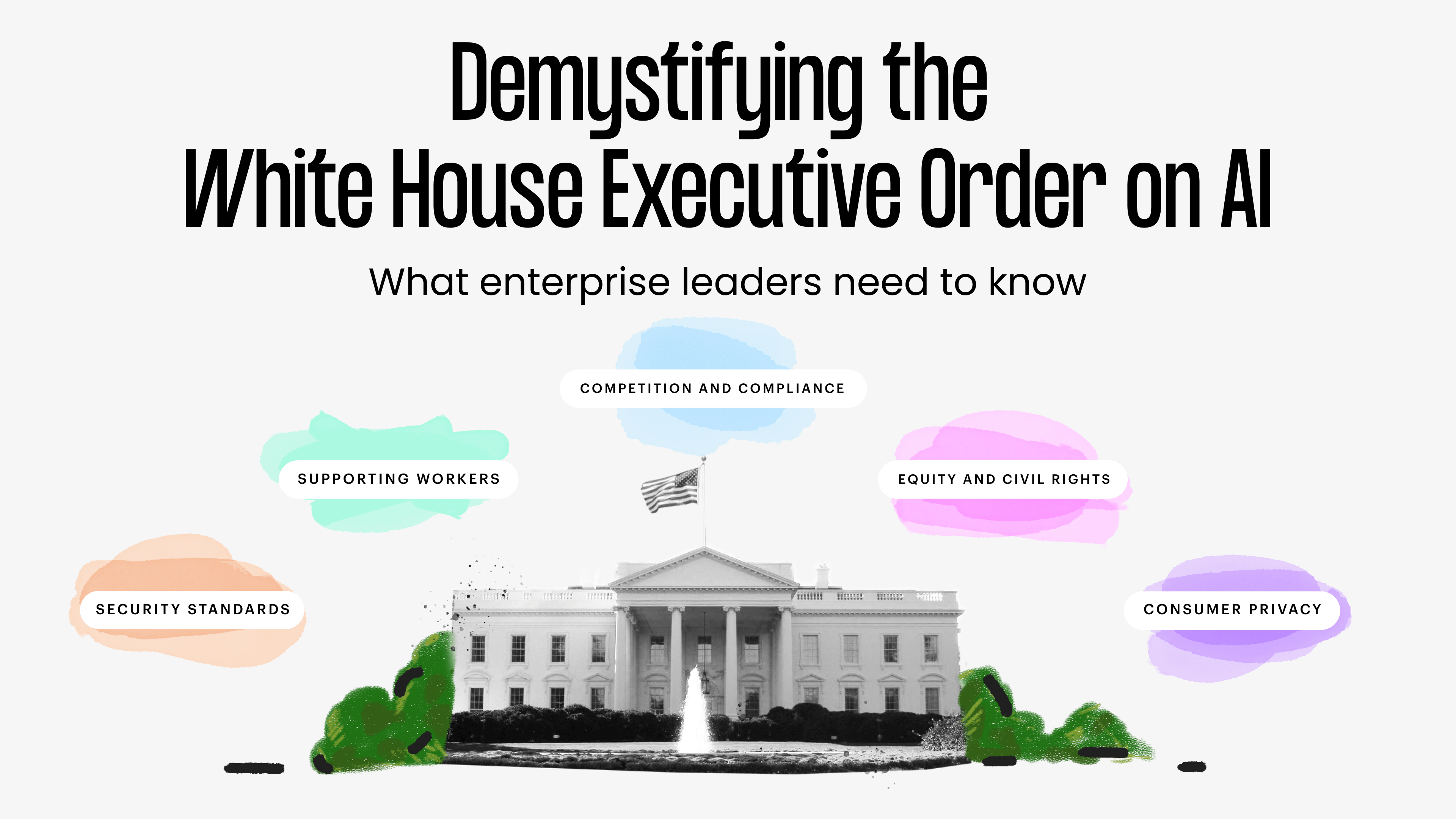
Learn more: Demystifying the White House Executive Order on AI
Intuit began using generative AI several years ago, beginning with an internally built tool called Elroy. While Elroy’s capabilities were very basic, it was a way for Intuit to begin operationalizing generative AI within its workflows. However, the output wasn’t great and it took a long time to generate any usable content. Over time, as more employees needed to use generative AI, Intuit created guidelines for voice, tone, style, accessibility, and inclusive language.
Intuit wanted a solution that would help writers make decisions while using generative AI and not disrupt the flow of work. Intuit chose WRITER as a solution, rather than continuing to rely on an internal product that didn’t meet its content principles.
This is a great example of a “build then buy” scenario, which I anticipate we’ll see a lot more of in the coming months as enterprises explore in-house generative AI solutions. The lesson here: it’s never too late to change your mind, and enterprise-focused vendors like WRITER can help ease the transition from “build” to “buy.”
How to mitigate risk with your investment
Generative AI needs to be approached with a well-informed perspective. This arena is too large and too much of a long-term investment to “go it alone.” If you don’t have the internal expertise, find the right external experts, consultants, or partners to help you research and make decisions. At Perficient, I always like to start with a client’s concept of value and their broader objectives. Those answers inform the build-versus-buy discussion.
You can introduce risks without the right security and privacy guardrails: your sensitive data needs to stay secure. You should also revisit your governance practices and update your policies to include generative AI.
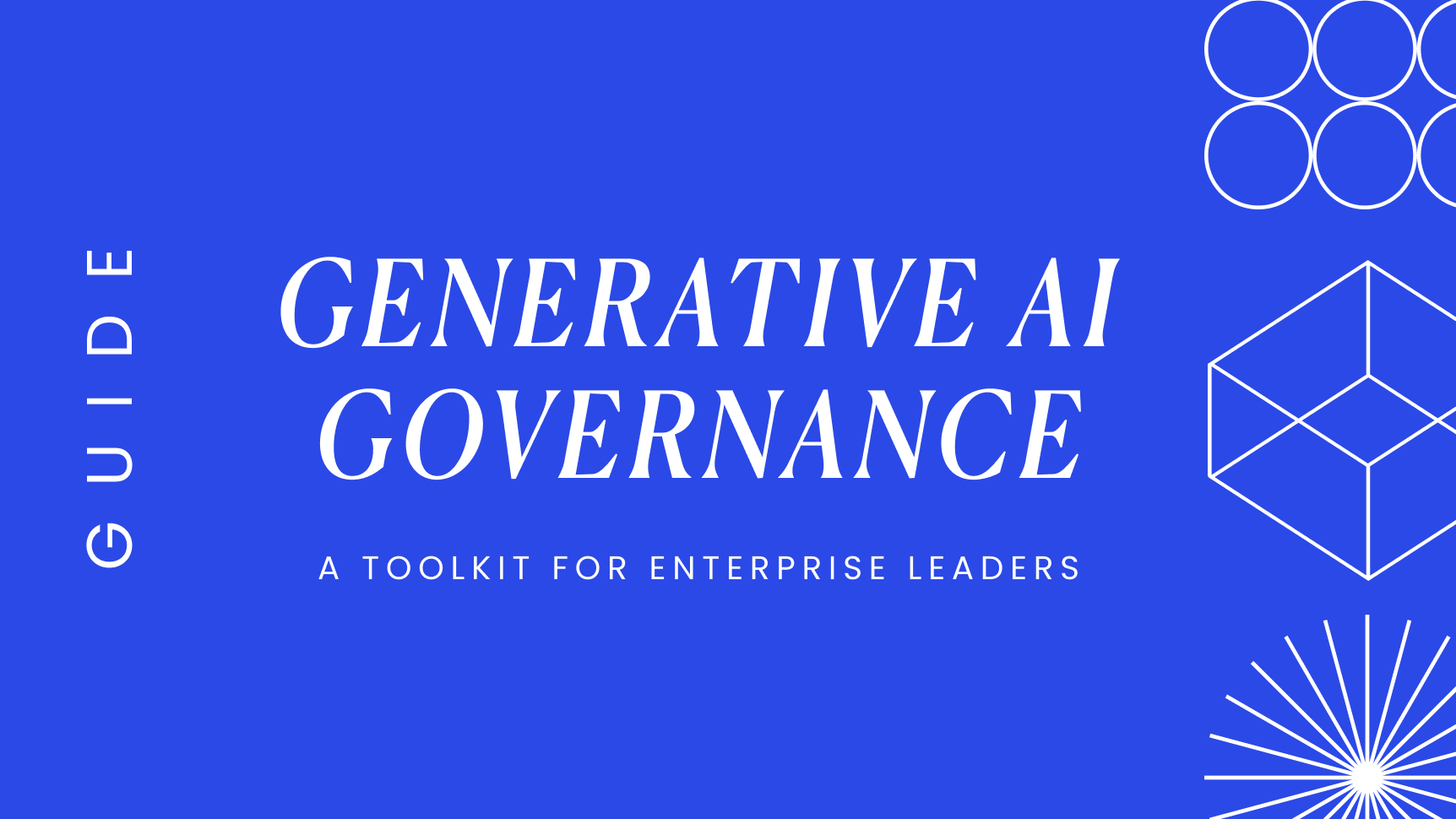
Learn more: Generative AI governance: a tool kit for enterprise leaders
If you’re considering building, start with a very small proof of concept to see what’s involved. Do this quickly. If you can’t build a proof of concept, you shouldn’t be building.
With buy, you can also start with a very small sample or use case. This will help you outline the requirements you’d need from a vendor. Vendors can also help you “prove out” their product as a solution to your business needs.
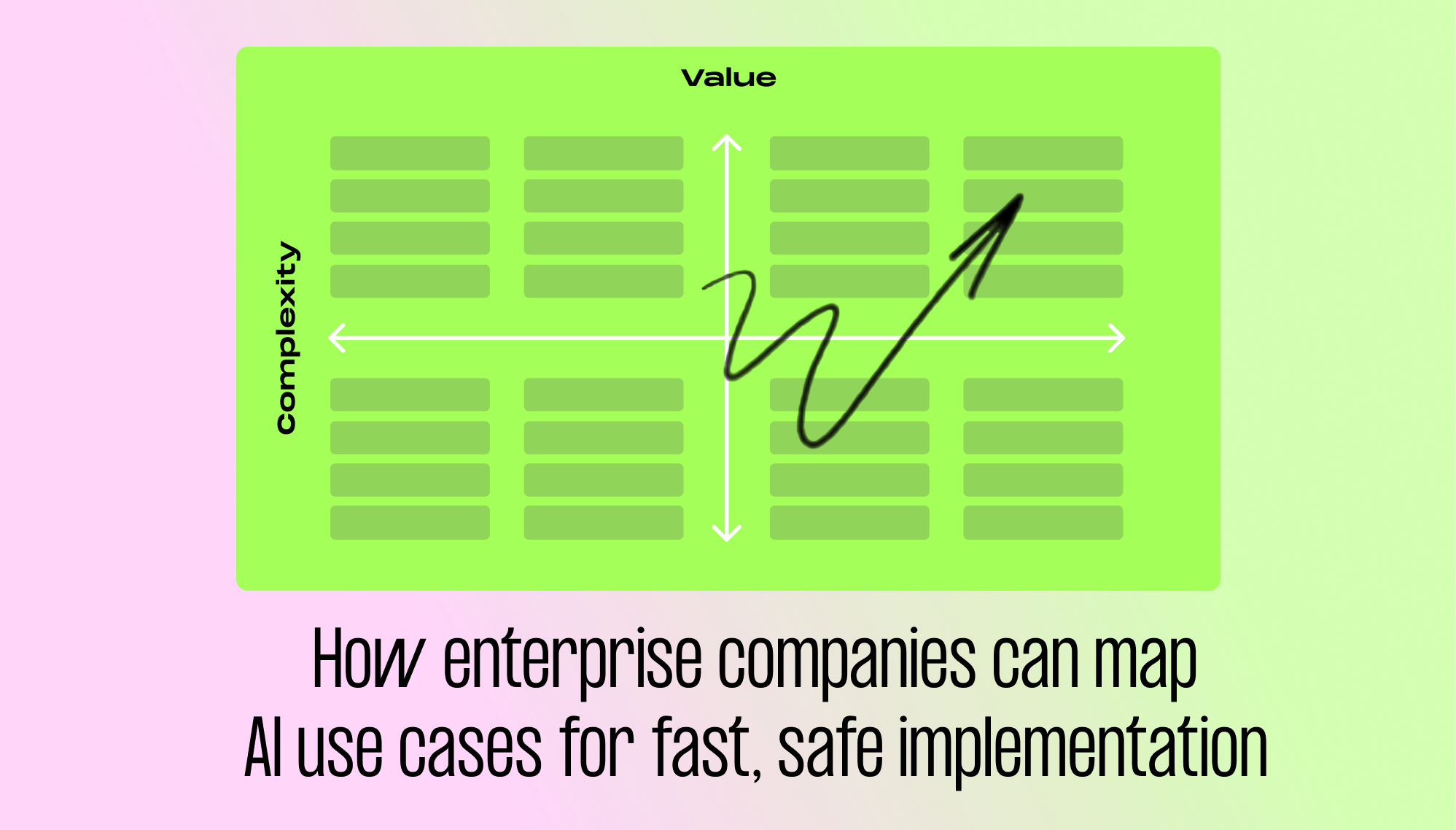
Learn more: How to map enterprise generative AI use cases
The answer isn’t “do nothing”
Build versus buy is often framed as an either-or, and some organizations are considering a third option: do nothing.
Some companies come to the conclusion that they should take a wait-and-see approach, rather than make an investment. In the fast-moving world of AI, that might feel tempting. It’s also a mistake. You’ll miss out on months (or years) of short-term ROI gains and long-term differentiation from your competitors.
Since implementing generative AI should be a sooner-rather-than-later decision and since building is so resource-intensive, the best option for most enterprise companies is to partner with a vendor like WRITER or a firm like Perficient that empowers you with its existing technology and works with you to build custom solutions unique to your business needs.
The baseline table stakes expected by your users and employees are changing very dramatically in the generative space, and the biggest risk is falling behind.
Check out The Business Leader’s Guide to Adopting Generative AI, to learn more about prioritizing use cases, change management, and more.
Robert Bagley is the Director of Data Solutions at Perficient. He leads the delivery of data-driven and machine learning-enabled products to create revenue opportunities and enhance customer experiences for various clients and industries.