Inside Writer
– 6 min read
RAG benchmarking: Writer Knowledge Graph ranks #1
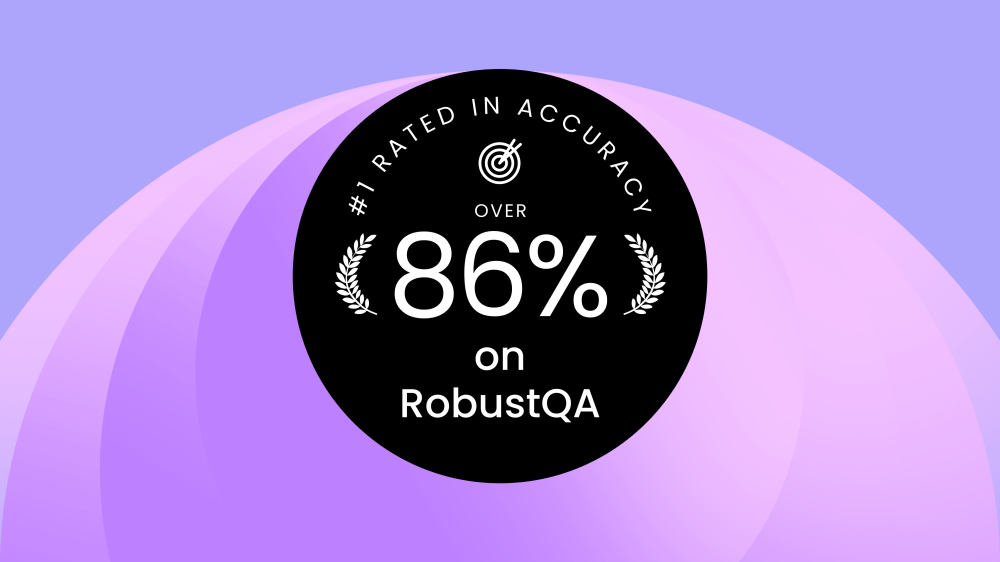
Retrieval-augmented generation (RAG) is transforming the way generative AI interacts with enterprise data. By grounding generative AI in data, RAG makes outputs more relevant and accurate.
At Writer, we’ve taken a different approach with our graph-based RAG. Unlike traditional vector retrieval methods, our Writer Knowledge Graph is more accurate, has fewer hallucinations, is fast and cost-effective to implement, and provides a scalable and easy-to-deploy solution for enterprises.
Today, we’re excited to share our RAG benchmarking report. This report compares Knowledge Graph with other RAG approaches on the basis of accuracy. Knowledge Graph achieved an impressive 86.31% on the RobustQA benchmark, significantly outperforming the competition, which scored between 75.89% and 32.74%. The evaluation included vector retrieval RAG solutions such as Azure Cognitive Search Retriever with GPT-4, Pinecone’s Canopy framework, and various LangChain configurations.
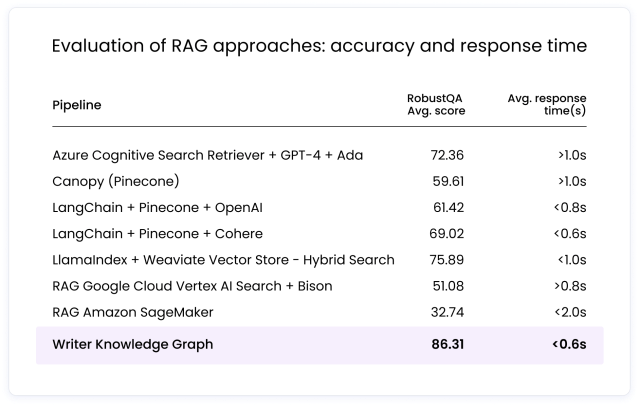
Writer Knowledge Graph is the clear winner with the fastest average response time of less than 0.6 seconds, making it an ideal choice for real-world applications.
- Writer Knowledge Graph uses retrieval-augmented generation (RAG) to improve the interaction of generative AI with enterprise data, ensuring outputs are more relevant and accurate.
- In a recent benchmarking report, Writer Knowledge Graph achieved an impressive 86.31% on the RobustQA benchmark, significantly outperforming competitors like Azure Cognitive Search Retriever with GPT-4 and Pinecone’s Canopy framework, and demonstrated the fastest average response time of less than 0.6 seconds.
- The RobustQA benchmark evaluated eight different RAG approaches on 50,000 questions across eight domains and over 32 million documents, reflecting real-world complexity and variations in query phrasing.
- Writer Knowledge Graph uses a specialized large language model (LLM) to create semantic relationships between data points, which improves accuracy and reduces the occurrence of hallucinations compared to traditional vector-based retrieval methods.
- Knowledge Graph is designed to handle both structured and unstructured enterprise data efficiently, overcoming challenges like dense mapping that can affect vector retrieval methods, making it scalable and suitable for enterprise applications.
Understanding the benchmarking study
RobustQA is a benchmark created by the Amazon team to evaluate open-domain question-answering across eight different domains — from biomedicine to technology — with 50,000 questions covering over 32 million documents. The size of the dataset ensures that the evaluation reflects real-world complexity and variations in query phrasing, which is really useful for assessing the accuracy and efficiency of RAG solutions.
We evaluated eight different RAG solutions, including our graph-based Writer Knowledge Graph and seven vector-based retrieval RAG approaches, with a focus on two key metrics:
- The RobustQA Score to measure the accuracy of a RAG approach
- Response time to evaluate its efficiency and scalability
The goal was to identify solutions that provide accurate and timely responses, as delays in answering queries can negatively impact user experience and scalability. These metrics help evaluate each RAG solution’s ability to handle different query types, ensuring that the selected solution will be able to meet the demands of real-world applications.
The benchmarking study results
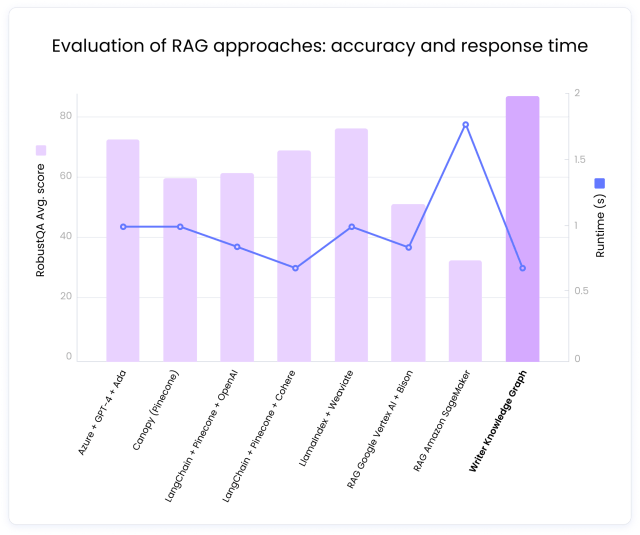
The benchmark results demonstrate that Writer Knowledge Graph is the top performer among the eight evaluated RAG approaches:
- It achieved an impressive accuracy score of over 86% on the RobustQA benchmark
- It delivered the fastest average response time of less than 0.6 seconds
By comparison, other RAG approaches fell short.
The next best implementation, using LlamaIndex with Weaviate Vector Store’s hybrid search, scored 75.89%, while Azure Cognitive Search Retriever with GPT-4 scored 72.36%. Pinecone’s Canopy framework and various configurations of LangChain with Pinecone and different language models scored between 59.61% and 69.02%.
This high score is important given the comprehensive nature of the RobustQA benchmark and the increasing difficulty of making incremental improvements as accuracy rates rise.
For example, moving from 70% to 80% accuracy is more difficult than moving from 60% to 70%.
3 key advantages of Writer Knowledge Graph
Higher accuracy and lower hallucinations
Traditional vector-based retrieval methods convert text data into numerical vectors and perform similarity searches.
Writer Knowledge Graph uses a specialized LLM trained to split structured and unstructured data into nodes and build rich semantic relationships between data points as edges in a graph structure. This allows Writer Knowledge Graph to complete question-answering tasks more accurately than vector retrieval RAG approaches.
Our retrieval-aware compression technique intelligently condenses data and indexes it with metadata for further context. We use advanced techniques with our state-of-the-art LLMs to minimize hallucinations.
Enterprise data at scale
Enterprise data often presents challenges for vector retrieval due to dense mapping. For example, in user manuals for different versions of a device, its name may appear frequently, resulting in a concentration of data points. This can lead to inaccurate vector estimates, causing a vector retrieval RAG to produce inaccurate answers.
Writer Knowledge Graph excels at processing structured and unstructured data, including documents, spreadsheets, charts, presentations, and more. Where a vector-based RAG breaks down with dense mapping, Knowledge Graph can use semantic relationships to pinpoint the most relevant data points. And unlike a vector-based RAG, adding or updating data in Knowledge Graph is easy, fast, and inexpensive.
Easy and secure deployment
Stitching multiple tools to build your own RAG and integrate it into your AI stack can take months and increase security risks.
Writer Knowledge Graph, on the other hand, is part of our full-stack generative AI platform, which includes state-of-the-art LLMs, customizable AI guardrails, and AI Studio, our suite of development tools.
We provide data connectors that allow you to securely and automatically sync data between your important data sources and Knowledge Graph. In addition, our full-stack platform enables the rapid deployment of AI applications built on internal data in just days, while providing the security, transparency, privacy, and compliance benefits of our platform.
Supporting enterprise use cases
Writer Knowledge Graph enables businesses to build AI apps that make internal knowledge easily accessible:
- Sales teams access real-time and accurate sales enablement on their market, product lines, and competitors, to speed up sales processes and increase win rates.
- Customer service agents at retail companies can easily ask questions about their product and policy details to close tickets faster and increase customer satisfaction.
- Financial advisors can quickly tap into internal documentation, industry news, and market research to reduce time required to generate bespoke client outreach.
Revolutionizing data retrieval with Knowledge Graph
With Writer Knowledge Graph, anyone can make AI-generated responses more accurate.
The RobustQA score of 86.31% and the fastest average response time of less than 0.6 seconds demonstrate its ability to handle complex queries, scalability, and efficiency, making it a top choice for enterprises that require knowledge retrieval.
Read the full benchmarking report to see how Writer Knowledge Graph can help you. Improve your data retrieval processes with precise, contextually accurate responses that outperform vector-based RAG approaches such as Azure Cognitive Search Retriever with GPT-4 and Pinecone’s Canopy framework. Experience fewer hallucinations and more reliable outputs with our graph-based approach. Keep your operations running smoothly as your data volume and query complexity grow with our RAG solution’s scalability and speed.
Start transforming your business today: get in touch with our team to learn how Writer can empower your organization with cutting-edge AI technology.