AI in the enterprise
– 9 min read
Tackling AI bias
A guide to fairness, accountability, and responsibility
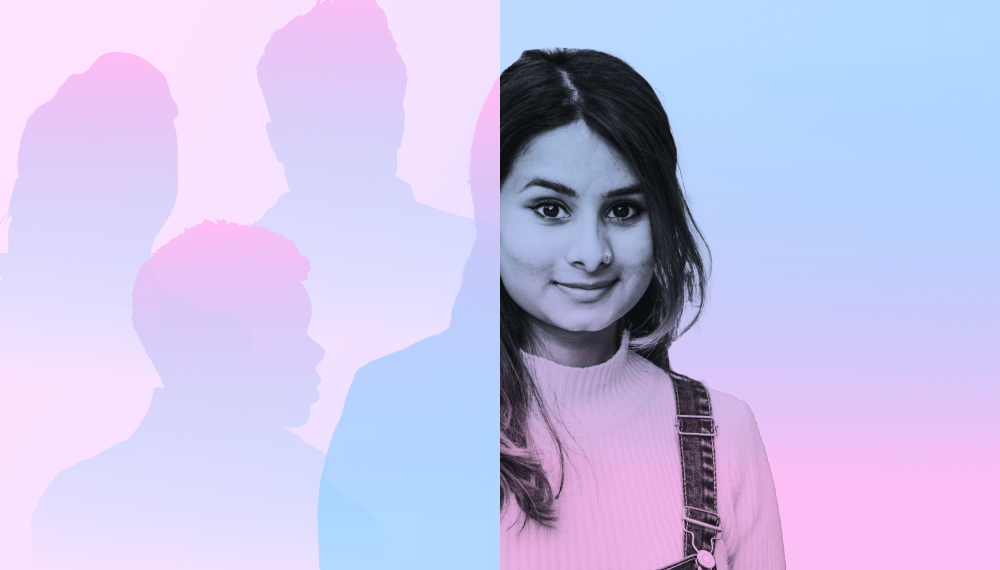
AI bias can perpetuate and amplify stereotypes, skew decision-making, and even result in unfair practices. For businesses, it’s a serious issue that goes beyond ethics to affect decision-making, threatening reputations and posing potential legal risks.
Here’s a little test to show just how biased AI can be.
Question — what stands out to you in these AI-generated images of a lawyer?
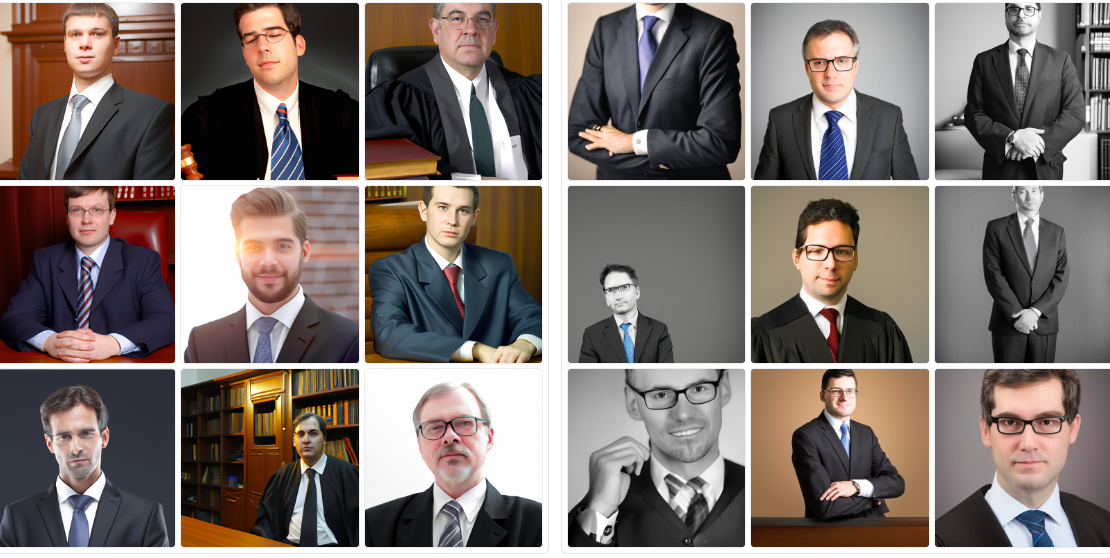
Answer — there’s no women or people of color in sight.
What about these images of nurses? Notice any men?
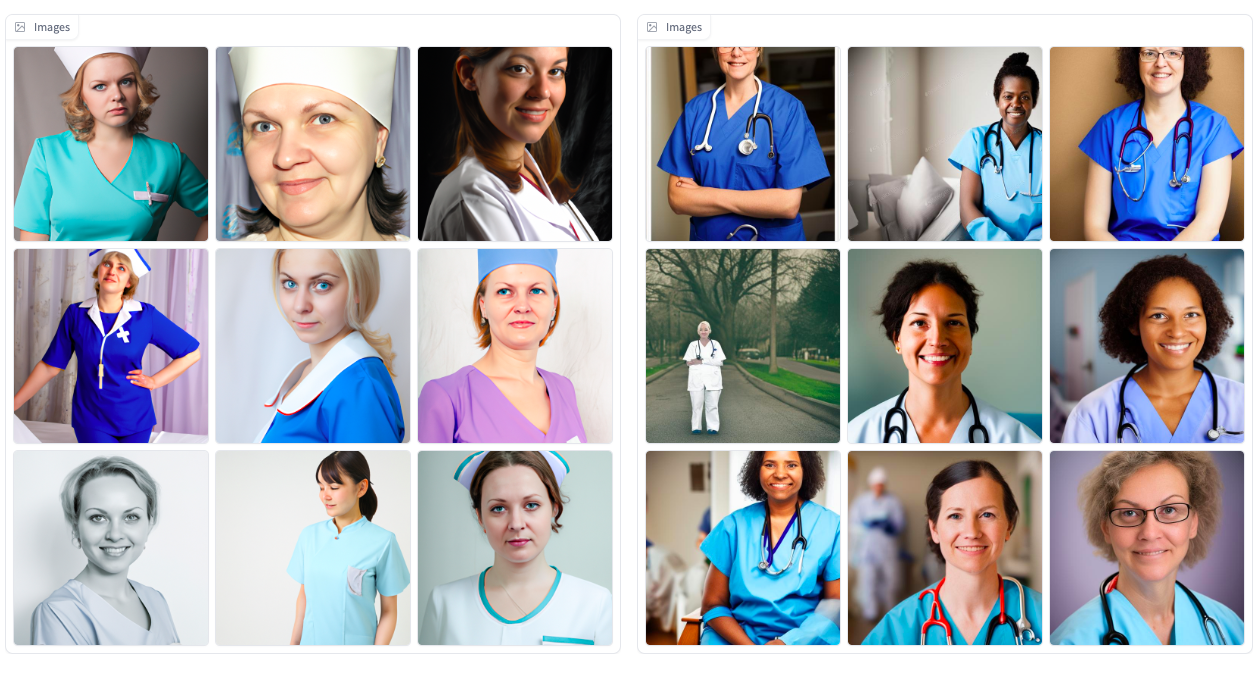
And what about here? How many women can you count among these scientists?
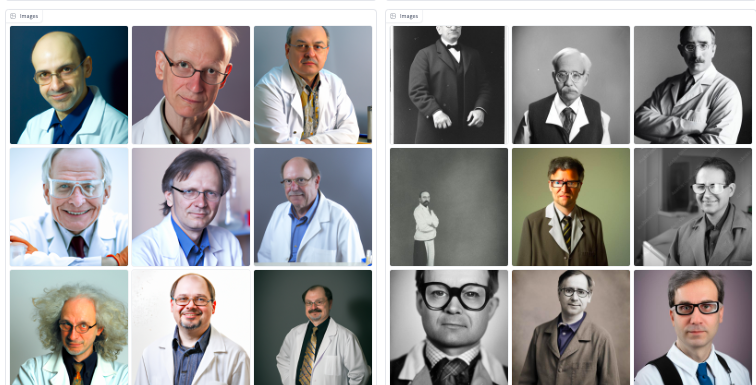
Zero. Zip. Zilch. Nada. Despite the fact that 44% of all scientists are women.
These images, taken from an online tool that examines biases in popular AI image-generating models, reveal the dark side of AI. For all of AI’s brilliance and transformative potential, its specter of bias casts a long shadow.
It’s a sobering reality that enterprises must face as they increasingly adopt AI into their operations.
Let’s confront this shadowy aspect of AI together. We’ll also provide guidelines for mitigating AI bias, ensuring that as your business ventures further into the realm of AI, you do so with fairness, accountability, and responsibility at the forefront.
- AI bias happens when developers create AI algorithms, models, and datasets with built-in assumptions that lead to unfair or inaccurate results.
- AI biases often show up as unfair or incorrect portrayals of people based on race or gender.
- Common types of AI bias include historical, sampling, labeling, and confirmation bias.
- Tools and strategies for preventing AI bias include training AI systems on diverse data sets, identifying and minimizing biases in AI models, regularly auditing and updating AI systems, including diverse teams in the design and decision-making process, and establishing a corporate AI policy.
- Developers, data scientists, and business leaders play a significant role in ensuring AI operates equitably and justly.
What is AI bias?
AI bias occurs when AI algorithms, models, and datasets have built-in assumptions that lead to unfair or inaccurate results. This bias can come from how data is collected, coding errors, or even the influence of a particular team or individual.
International Institute for Management Development (IMD) research finds that 72% of executives are concerned about generative AI bias. And our 2025 enterprise AI adoption report reveals that over half of employees say the information provided by their generative AI tools is regularly biased. Biased AI systems can lead to skewed decision-making, discriminatory practices, damaged reputations, and even legal consequences.
The consequences of this oversight are far-reaching, potentially leading to skewed decision-making, discriminatory practices, damaged reputations, and even legal consequences. Case in point: A lawsuit was recently filed against State Farm insurance, alleging that the company’s artificial intelligence systems are biased against Black homeowners.
The last thing you want for your company is a lawsuit or a tarnished reputation due to unchecked AI bias. For businesses using AI to thrive and be credible, addressing AI bias is vital. It’s not just about your company’s long-term success and credibility — it’s about equality and reducing the amount of biased data harming the world.
The most common types of AI bias
AI biases often show up as unfair or incorrect portrayals of people based on race or gender. They often mirror prejudices and old human biases.
By recognizing the different types of AI bias — whether they lurk in the corners of your datasets or subtly shape the algorithms’ decision-making processes — you can make important strides toward creating AI applications that are more equitable and fair.
Historical bias
This occurs when the training data used for an AI system reflects historical prejudices, deeply ingrained societal norms, or long-standing inequities.
Example:
A 2024 UNESCO study found that a strong association between gender and career or family still exists in current generation models. Female names were associated with “home,” “family,” “children,” and “marriage” — while male names were associated with “business,” “executive,” “salary,” and “career.”
Sampling bias
This arises when the data used to train an AI system doesn’t represent the population that the system will serve.
Example:
In 2018, Amazon’s AI hiring tool was found to be biased against female job candidates. It was trained on 10 years of resumes that were mostly from men. This caused the algorithm to favor male candidates over female ones, making the hiring process unfair.
Labeling bias
This is when a machine learning algorithm labels data inaccurately because the data set it’s trained on is biased.
Example:
BuzzFeed used Midjourney to generate 195 different Barbies from around the world. The images were extremely misrepresentative, amplifying harmful global stereotypes. Even when humans try to correct biased training data by labeling images themselves, they introduce their own biases.
Confirmation Bias
Trainers configure an AI system to confirm pre-existing beliefs or assumptions, often ignoring contradictory data.
Example:
Social media companies design algorithms to profit from humans’ confirmation bias by showing users more of the content they already engage with. This can create an “echo chamber” where users only see what they want to see, regardless of its accuracy or social impact. This can reinforce harmful views or stereotypes, as users may not get to explore different viewpoints or challenge their beliefs.
Tools and strategies for preventing AI bias
Once you’ve identified the ugly specter of AI bias, the next step is to confront it head-on and prevent its resurgence. This calls for the right tools and methods — from sourcing diverse and representative datasets to employing rigorous testing and validation methods. Every step refines your AI, making it more equitable and effective and ensuring it’s a helpful ally rather than a harmful entity.
Train AI systems on diverse data sets
The first line of defense against bias is using a diverse and representative dataset for training your AI models. As Dr. Timnit Gebru aptly points out, “If we don’t have the right strategies in place to design and sanitize our sources of data, we will propagate inaccuracies and biases that could target certain groups.”
If the training data is biased, AI will likely produce biased outcomes. Therefore, machine learning algorithms should include a diverse set of data points that represent different demographics, backgrounds, and perspectives.
For example, let’s say you’re training a sentiment analysis model to detect customer satisfaction from reviews. The training data should include comments from various customers from different backgrounds, ages, genders, and ethnicities.
By using diverse datasets and implementing the right strategies to “sanitize” these data sources, as Dr. Gebru suggests, you can reduce the risk of your AI making incorrect decisions due to implicit biases or lack of context.
Identify and minimize biases in AI models
Spotting and reducing biases in AI models is another key element to ensuring fairness, trust, and accuracy. You can use tools like AI Fairness 360 or What If to detect potential biases and flag questionable results that may lead to unfair decisions.
If your business is using biased algorithms, you can reduce or eliminate them by fine-tuning. This involves training a machine learning model to improve its performance for a specific task. Allowing the model to adapt to new, more varied data can reduce the impact of any biases in the original training data.
Regularly audit and update your AI systems
It’s important to audit your algorithms regularly to ensure they’re not producing unfair outcomes or disparities over time. For example, you can use fairness metrics to measure how fair the AI system is and test things like whether it discriminates against certain groups.
Your audits should also check if anyone has added new datasets or adjustments that could introduce bias into the model’s results.
Include diverse teams in the design and decision-making process
Tech has a diversity problem. That’s not exactly a news flash, it’s been obvious for years. According to the 2019 report on Discriminating Systems — Gender, Race, Power in AI, over 80% of AI instructors are male. Additionally, Latinx and Black workers make up only 7% and 9% of the STEM workforce, even though they represent 18.5% and 13.4% of the US population. And, only 7% of women were given AI upskilling opportunities between 2022-2023, compared to 12% of their male peers.
Given these numbers, it’s hardly surprising that AI systems frequently exhibit biases. Overlooking certain groups and communities from data representation can lead to skewed algorithms.
As Computer Science Professor Jim Boerkoel notes in a Forbes interview, “If the population that is creating the technology is homogeneous, we’re going to get technology that is designed by and works well for that specific population. Even if they have good intentions of serving everyone, their innate biases drive them to design toward what they are most familiar with.”
To address this, including diverse teams in the design and decision-making processes is crucial. This includes age, gender, race, ethnicity, experience, and intellectual background. Diverse teams can foresee and mitigate potential fairness issues, which instills more objectivity and results in less biased outputs.
Establish a corporate AI policy
Create a corporate AI policy on how data is collected, stored, used, and analyzed. Your corporate policy should emphasize ethical AI practices, responsibility, transparency, and a commitment to minimizing AI bias. This ensures that all teams follow best practices regarding the ethical use of collected data. It also helps protect customer data and promote customer trust in your company’s processes.
The road ahead: achieving a bias-free, fair, and equitable AI
Artificial Intelligence holds tremendous potential as a powerful ally in various aspects of business. However, if mismanaged, it can also become a malevolent force that disproportionately impacts women and minority groups.
The task of mitigating AI bias doesn’t rest upon the shoulders of users but rather on those who develop and deploy these systems. Developers, data scientists, and business leaders play a significant role in ensuring AI operates equitably and justly. From training datasets to fine-tuning models, every step in developing AI requires a vigilant eye for potential bias.
Choosing your AI technology partner is also of paramount importance. Ensure that you’re working with an AI company that has models with curated datasets (like WRITER’s Palmyra LLM) and low toxicity scores.
For leaders contemplating AI adoption, we recommend our comprehensive guide on integrating AI into your enterprise. It covers identifying and prioritizing use cases, preparing for change management, introducing governance workflows, and safeguarding brand reputation while employing generative AI and agentic AI. With AI at the heart of your operations, you can drive success while ensuring fairness and equity.