Humans of AI
– 11 min read
Balancing tech and human touch: JD Sanchez, CSAA Insurance Group
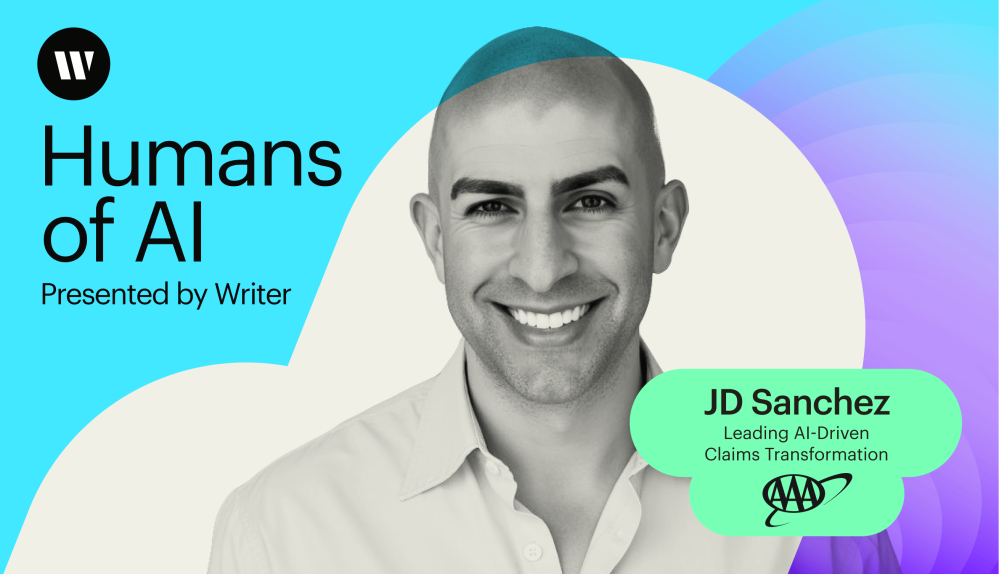
In the dynamic realm of insurance, AI-driven claims transformation isn’t just a buzzword or a fad — it’s a catalyst for significant change. Our latest guest on Humans of AI, JD Sanchez, explains exactly how he uses AI — and characterizes it — in his day-to-day work.
JD is the AI-driven claims transformation lead at CSAA Insurance Group. His journey into AI began with a healthy dose of skepticism but quickly evolved into a passionate exploration of its potential. Join us as we learn how JD connects technologists and subject-matter experts (SMEs), and how he thinks AI can revolutionize how we think about problem-solving in complex industries.
- JD was initially skeptical of AI but became a passionate user after experimenting with it for a business plan.
- He uses AI to improve efficiency and accuracy in daily professional tasks, including emails, SQL queries, and PowerPoints.
- JD created personas representing different AI agent use cases to illustrate responsible AI use within his organization.
- He emphasizes human focus, accountability, and transparency when using generative AI — especially in complex decision-making situations.
- JD highlights the significance of subject-matter expertise and effective prompting in building meaningful AI solutions.
From AI skeptic to powerhouse user
Like many others, JD was initially skeptical about AI. So, in early 2023, he decided to challenge AI with a task that used his extensive marketing experience: building a high-level business plan for an outpatient rehab facility in a specific city. He started broadly, with just some general marketing and branding ideas.
“And then I’m like, ‘Oh my goodness, this is actually quite good,’” JD says. “This isn’t just a gimmick. Let me dial it in further. And so I don’t know how many hours I lost.”
He dove deeper and deeper, asking for more detailed information — like the town’s demographics. AI gave him data on national disease rates, interventions, emergency medicine visits, and other relevant statistics.
“I finally got to a point where I was so deep into it, I got so upset because it couldn’t give me traffic patterns and like walking flow for a particular intersection, for a brick-and-mortar location,” he says. “My life has not been the same ever since.”
Transforming day-to-day tasks with AI
Right now, JD leads an AI-driven claims transformation project — but he also uses AI in other professional areas, including emails, SQL (structured query language) queries, and PowerPoints.
Emails
He uses AI to draft emails, but the key is critiquing and refining these drafts to make sure they resonate with the recipient.
“[AI] makes sure that anything that I might have written in haste — or the types of emails that historically you should sleep on and send tomorrow — you can evaluate,” he says. ”You can have the refinement and get it out in a more timely capacity.”
SQL queries
Another way JD uses AI in his daily work is to help with SQL queries. When dealing with a database, extracting specific insights can be challenging. JD found that using a semantic interface, like an LLM, greatly simplifies this process.
By giving AI information about the database structure — such as the names of the tables, columns, and what data they have — JD can easily ask for the information or insights he needs.
“I’ve discovered large language models are very, very helpful in constructing those queries,” JD says. “And being able to let, maybe, a non-tech technical person get those insights on demand that they need on an ad hoc basis.”
Palmyra, our family of transparent LLMs, allows non-technical individuals to run SQL queries without writing code, just like JD says.
PowerPoints
Rather than simply drafting slides, JD uses AI to improve his presentation’s visual and conceptual aspects. For example, when he needs to convey specific information, he asks AI to suggest icons that resonate with the message or to provide ideas for visualizing complex process flows.
“Even just through a language interface, large language models can be very descriptive,” JD explains. “And then essentially hold your hand and guide you to produce something. That’s impactful and efficacious for the business.”
Creating a storybook of AI agentic characters
JD notices that many people tend to anthropomorphize AI, treating it as a person or creating avatars. This can lead to confusion about what AI agents are and how they function, especially in agentic workflows.
To navigate this complexity, JD crafted personas representing different use cases and vantage points for autonomous AI systems.
“That exercise of crafting these personas in an illustrative type of way — joining them with their function — has allowed me to kind of solidify potential ways to apply AI throughout the enterprise,” JD says.
For enterprises, compliance is crucial. Enterprises must avoid introducing bias, and they need to make sure that AI-driven decisions or determinations are objective and repeatable. By associating AI functions with these illustrative personas, JD better understands and communicates the responsible use of AI technology within his organization.
The firefighter
The firefighter persona is a reactive AI agent. This type of agent makes quick, on-the-fly decisions based on the data it receives.
Use cases: High-pressure situations like real-time trading, predictive healthcare monitoring, or social media sentiment monitoring.
The adventurer
The adventurer is an AI agent that can make its own decisions, train, and refine itself over time. It navigates uncharted territory and adapts to new challenges in real-time.
Use cases: Logistics, route optimization, data mining, or even space exploration.
The student
The student is an AI agent that can learn, change, and fine-tune its responses on the fly.
Use cases: Personalized product recommendations or employee training platforms.
The watchmaker
The watchmaker is an AI agent trained on very specific tasks — much like someone who’s dedicated their career to perfecting one specific craft.
Use cases: Accuracy-dependent situations like fraud detection, image recognition, or contract analysis.
The detective
The detective is an AI agent that uses historical data based on past interactions to identify patterns and uncover insights about hidden relationships across complex situations.
Use cases: Customer relationship management or litigation.
The butler
The butler is an AI agent built to provide personal, ongoing support.
Use cases: A virtual assistant or a customer support chatbot.
The kitchen brigade
The kitchen brigade persona is a multi-agent system where each agent handles specialized tasks.
Use cases: Workflow optimization in eCommerce, healthcare, or smart warehouses.
The referee
The referee is an AI agent that ensures consistency across the board. It delivers unbiased decisions and follows the rules every time. Think of a soccer referee who must make unbiased decisions without favoring any team — regardless of their previous performance, the players’ backgrounds, current news headlines, or the fans in the stadium.
Use cases: For enterprises where clarity, control, and following the rules are non-negotiable.
Debunking the “tech wizard” stereotype
JD built his career by being at the forefront of emerging technologies. This often means that the people around him — potential customers, employers, managers, family, and friends — may not fully understand what he does or how he does it.
One of the challenges JD has faced is the tendency for people to view him as a “wizard,” regardless of the complexity of the task. Whether he’s helping someone with a simple Outlook issue or undertaking a complete digital transformation, the level of perceived wizardry remains the same. People don’t always grasp the difference between these tasks.
“And so I’ve had to explain maybe more advanced concepts succinctly, clearly for those that aren’t maybe well-versed in technology or technical terms or don’t have the context,” he says. “I think that’s led me to some of the storytelling and maybe reading a lot of books when I was a child and not being able to watch TV.”
Turning fictional problem-solving into real-world innovation
JD’s early love for literature and clear communication shaped his approach to problem-solving in the real world. For example, JD had the entire Hardy Boys collection — featuring Joe, Frank, and Chet in their iconic blue hardcover books. He’d often visit used bookstores to hunt for any missing volumes in the series.
We don’t think it’s too far of a reach to say that the Hardy Boys influenced him, as now he’s always trying to solve problems for the enterprise.
“You have to be a forward-thinking problem solver,” JD explains. And you’re trying to figure out complex problems, not complicated problems. Complicated problems are those that other folks have figured out before you, and they might require a lot of steps. There might be some intricacies, a variety of ways to go wrong, and you might need documentation to actually get to the solution.”
But complex problems demand novel solutions. Just like the Hardy Boys solving mysteries on their bicycles, JD uses AI to address intricate challenges in large enterprises.
The three guiding principles of AI strategy
JD sees significant opportunities for impact by using generative AI and AI technology — particularly in its synergistic relationship with automation, advanced AI, and predictive analytics. Many people feel comfortable with safer use cases, such as using generative AI for data extraction and summarization.
But, JD notes that the real challenge lies in more complex use cases where AI begins to generate output that resembles decision-making. His organization is cautious about these applications and adheres to guiding principles emphasizing a human focus, accountability, and transparency.
Human focus
AI must augment the human touch, not replace it. JD says they aim to improve customer and employee experiences by emphasizing human knowledge and interaction. It’s a sentiment we share here at Writer like our CTO and Co-founder Waseem AlShikh explained on his episode of HoAI.
Accountability
JD’s organization is highly regulated, varying state by state. So, they make sure AI solutions comply with existing regulations and anticipate future ones. They’re committed to protecting customer data and avoiding bias in their models and outputs.
Transparency
One of the biggest challenges with generative AI is ensuring its output’s accuracy, reproducibility, and transparency. JD’s organization aims to make AI-driven solutions explainable by providing an audit trail and provenance, so users can clearly understand decision-making processes. (No unexplainable models, please.)
“Is the output accurate?” JD asks. “Can you reproduce the output? Is it transparent? How’d we get here? And then essentially, in our case, is it explainable also?”
Bridging technological and operational expertise
JD highlights that there will be a fundamental knowledge gap between technologists and SMEs, no matter what industry you’re in. On one side, tech experts speak in technical terms, programming languages, and algorithms. On the other side, operational experts are well-versed in industry standards, regulations, and workflows. This creates multiple barriers, including a language barrier and misaligned expectations. JD says SMEs often get caught up in the hype and marketing around AI, seeing it as a magical fix for everything.
Framing the problems that need solving is tricky because technologists have a hard time understanding the subtleties of real-world business workflows. And SMEs struggle to explain their challenges in a way that technologists can tackle directly.
“So you see folks like me that come in and need to navigate these waters, speak these languages, and be able to be a conduit or a liaison and basically shepherd everybody towards these solutions, these implementations,” JD explains.
Prompting and problem-solving at the heart of AI usage
JD encourages folks to build hands-on experience and push the limits with AI. Don’t just take others’ word for it. Try to replicate and refine what they’ve done. To do this, JD lets us in on a secret: It’s all about prompting and subject matter expertise.
To build meaningful solutions, you must know your industry and the real-world problems that need solving. Understanding the pain points of businesses, employers, managers, and customers is crucial. Then, you have to become a proficient prompt engineer.
“Understand what you can get out of these large language models so that the outputs of the technology aren’t generic — they’re specific, useful, and impactful,” JD says. “The better that you can prompt these models, the better you’ll be able to build solutions or ideate and create initiatives that address problems nobody has been able to address until this technology came along.”
While many technologists can integrate AI into businesses and vendors with fantastic platforms, the core challenges and the art of prompting will remain constant. The technology will only improve, but the ability to articulate and solve real-world problems will always be essential.
Want to hear more stories from the humans working at the crossroads of business and generative AI? Subscribe to Humans of AI wherever you listen to podcasts.