Humans of AI
– 11 min read
Dispelling magical thinking and the black box of AI with GWU’s Patrick Hall
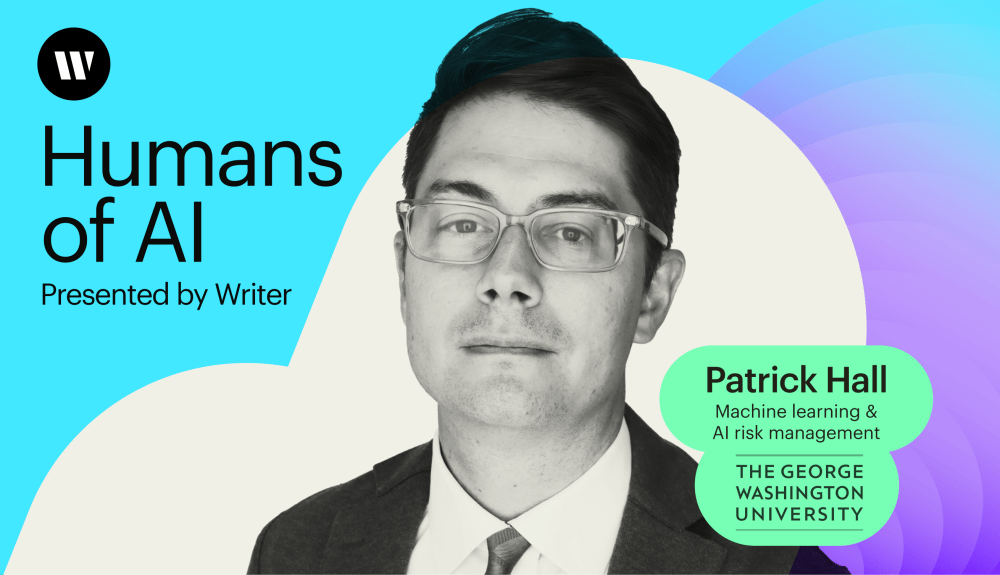
There’s a famous quote by sci-fi author and futurist Arthur C. Clarke: “Any sufficiently advanced technology is indistinguishable from magic.” The waves of generative AI innovation in recent years — and the accompanying marketing and media hype — epitomize this sentiment. Articles about AI liken the technology to a genie ready to grant any wish a user can imagine. Software apps have even adopted a fantasy-inspired icon to represent AI features: the starry remnants of a spell cast by an unseen magician.
But while the capabilities of AI inspire wonder in many users, it’s crucial to recognize that there’s no magic going on behind the scenes. It’s important that we figure out how to explain the seemingly unexplainable. To do this, we need humans like Patrick Hall challenging the allure of black-box technology.
In this episode of Humans of AI, we delve into the story of Patrick Hall, an assistant professor of Decision Sciences at George Washington University. Patrick also advises large banks and government agencies on AI risk management, and he’s on the board of directors of the AI Incident Database.
With a deep-rooted belief in the scientific method, Patrick navigates the complex world of AI, striving to demystify its inner workings and ensure responsible practices. Join us as we explore his insights on the importance of asking the right questions, the challenges of unexplainable models, and the need for transparency and governance in the AI industry.
- Patrick highlights the importance of asking the right questions and using deductive reasoning in solving mysteries and understanding AI.
- He explains the challenges posed by unexplainable black-box models in the AI industry and the need for transparency and governance.
- The scientific method plays a huge role in demystifying AI and making real progress in AI risk management.
- Patrick brings attention to the need for audits and responsible AI governance to manage risk in complex AI systems.
- He stresses the significance of understanding the sociotechnical aspects of AI systems and the importance of collaboration between different entities for effective AI governance.
Unraveling mysteries with the scientific method
The solution to a good mystery doesn’t rely on magic or parlor tricks. It all comes down to a detective asking the right questions, using deductive reasoning, and a little bit of science. In the world of AI, Patrick is playing the part of the detective, and he’s armed with his favorite tool: the scientific method.
Patrick believes that if anything is ever to save us from climate change, failing democracies, or nuclear proliferation, it’s the scientific method. These are the more burning issues of our time, but he recognizes AI’s potential to worsen those problems.
“If I can spend my time getting some problems with AI under some amount of control, then I find that very motivating to prevent the acceleration of what I would say are more pressing social or global issues,” Patrick says.
In the rush to bring new products to market and stay ahead of competitors, many AI development companies undermine the scientific method with confirmation bias in testing.
“AI governance says to stop doing stuff like that and start applying the scientific method to make real progress,” Patrick explains. “I think that’s probably the part of it that’s most inspiring to me and the part that I feel like I can focus on and make progress on.”
Still, many AI companies offer little transparency about their training data. OpenAI’s CTO, Mira Murati even recently admitted she wasn’t sure where Sora’s (OpenAI’s video-generating AI tool) training data came from.
And some AI companies conflate their model benchmark scores by announcing testing results on models before they undergo a process known as quantization. Quantization prepares models for deployment at scale, but studies show that the accuracy of models decreases post-quantization.
The challenge of unexplainable models
Patrick says a hallmark of machine learning is that the models are unexplainable. Models operate as black boxes, where the internal workings and decision-making processes aren’t easily discernible or explainable. These models — although often perceived to have performance advantages — present significant risks due to their complexity and lack of transparency.
As Patrick aptly puts it, “If a system is so complex that no one understands how it works and it’s causing problems — say it has some non-discrimination issue or it has some data privacy issue — you have to go back to the drawing board. You can’t fix it because nobody understands it. And if a system is so complex that no one understands how it works, it’s hard to know if it’s been altered.”
AI engineering teams sometimes deviate from the traditional scientific method in the pursuit of developing AI systems, which leads to confirmation bias. Instead of following the traditional method of using past studies to formulate a hypothesis, they explore the data to come up with a hypothesis specific to that dataset. This is then tested within the same dataset. Patrick argues for a return to traditional science and fieldwork to truly understand how these systems impact communities and users.
“We’re going to have to buckle down and do some more traditional science and field work, and understand how these systems impact communities and users,” Patrick explains. “And not just think of some test data metric as the end-all, be-all assessment of whether a system is good.”
That’s what the AI Incident Database is for.
“It has 600 and some examples now, which is way too few,” Patrick says. “There’s clearly been many more AI incidents than that, but we have about 600 examples of automated systems going wrong. There’s this whole range of failure modes to learn from if people are interested in that.”
Humans are drawn to ‘magical thinking’
From a technical standpoint, the issue of transparency has been solved, Patrick says. Researchers have already proven it’s possible to build machine learning models that are completely explainable.
Cynthia Rudin — a professor at Duke who focuses on machine learning tools — entered the Explainable Machine Learning Challenge in 2018 with a team of other Duke members. While the participants were tasked with creating a black-box model and explaining how it worked, Rudin’s team instead created a fully interpretable model. This ethical alternative gives us transparency behind how variables are combined to form a final prediction in machine learning models.
And with a generative AI platform supported by graph-based RAG, it’s possible to have a visual representations of a model’s “thought process.” The model shares the data sources and the subqueries it created to deliver an answer to a question.
But that doesn’t stop consumers from being drawn to hype around unexplainable models. Attention-grabbing headlines herald the emergence of a “superintelligence” with every new LLM released by tech giants.
“I think life is hard and boring and we all want some kind of magic thing to come save us,” Patrick surmises. “And I think there’s a self-aggrandizing element here as well. It’s like, ‘I made a system that’s so complex, no other humans can understand it.’ Basically, it’s just unscientific magical thinking.”
Without transparency, we risk the excuse of ignorance posing severe threats. Even individuals with good intentions can claim they were unaware of the presence of XYZ information in the training data due to its intricate nature.
“That’s the real reason we have to talk about transparency and governance,” Patrick stresses. “Generally, when people are acting in good faith, transparency is important. When people are acting in bad faith, then we really have to have transparency.”
The role of audits in AI governance
Patrick believes that audits are an essential tool for managing the risks associated with AI systems. He warns, however, that the requesting, execution, and objectives of these audits can greatly vary. But the beauty of an AI audit, as opposed to a technical evaluation, is that there’s generally never any technology problems with an AI system.
“AI systems are sociotechnical systems,” Patrick explains. “All the problems arise from people. All technology problems are people problems. So in that audit report, we might get into sort of the governance processes and cultural issues. I think that’s a really important aspect of the audit, the sociotechnical side of the outcome.”
Mere curiosity or proactive measures alone rarely ever trigger audits, Patrick notes. They’re often prompted by whistleblowers, Senate hearings, enforcement actions, or lawsuits. It typically comes from legal or governance teams within an organization — and rarely business or technology — because there’s more money to be made by highlighting the outlandish things AI can do rather than spotlighting what it can’t do.
Patrick likens governance around misleading AI technology claims to that of the ESG movement in the retail and consumer products industry. “Think about the whole ESG movement, and how there’s so much greenwashing. [Black-box AI] is the same phenomenon as greenwashing,” Patrick emphasizes. “This can feel depressing, right? But I think once you accept reality, it can be empowering, because then you can make real progress.”
The dawn of a new industry
In Patrick’s view, the emergence of the AI industry isn’t special. He sees parallels with any other industry before it, such as the plastics industry. What we’re witnessing now is akin to DuPont rolling out plastic bottles for the first time.
“Big industries start and they grow and good things happen and bad things happen and people are harmed and people make a lot of money, and sometimes the products are useful,” Patrick says. “We’re witnessing the start of a new industry. It takes constant self-education and constant study and constant work to cut through magical thinking and the hype around this stuff.”
Speaking of hype, it’s no secret the tech industry thrives on hype — Gartners even documented a phenomenon known as the technology adoption hype cycle.
As a tech consumer or technology decision-maker at an enterprise company, continuously sifting through exaggerated claims requires effort. Even if you limit your reading to peer-reviewed articles, there’s no guarantee of receiving entirely accurate information. Still, Patrick says sourcing information from academic journals and reputable industry publications is far better than getting your information solely from TikTok or other social media platforms.
“What we need to get through all this is an information diet where we’re all very conscious about the information we consume,” Patrick says.
To help people get started on a healthy information diet, Patrick has created a resource library, where he maintains and curates a list of practical and responsible machine learning resources.
Responsible AI requires taking responsibility
As we strive toward creating fair AI systems and using AI responsibly and ethically, it’s key to work with traditional functions rather than trying to forge a new direction.
“The reality is that companies don’t want to be responsible with AI — they want to make money with AI. That’s their first job,” Patrick explains. “They already have these internal functions that are there to provide governance that they’ve accepted, and you will just make more progress working with them.”
Humans that work inside organizations using AI need to remember it’s not all glitz and glam. It takes real, human work to make progress and change.
“Experiments use cases, studies — that’s what it takes to get it right,” Patrick believes. “That’s boring, hard work, which doesn’t have the fun automation and AI vibes that are out there in the market today.”
The essence of a captivating mystery — whether it’s in a detective story or in the realm of machine learning interpretability — lies not in the mystery itself, but in the logical solutions we uncover through thoughtful questioning. It’s time for more of us to join Patrick and put on our detective caps. Equipped with powerful tools like the scientific method and critical thinking, we can pioneer innovative approaches to ensure the responsible use of AI in this emerging industry.
Want to hear more stories from the humans working at the crossroads of business and generative AI? Subscribe to Humans of AI wherever you listen to podcasts.