AI in the enterprise
â 12 min read
AI guardrails: How to build safe enterprise generative AI solutions, from day one
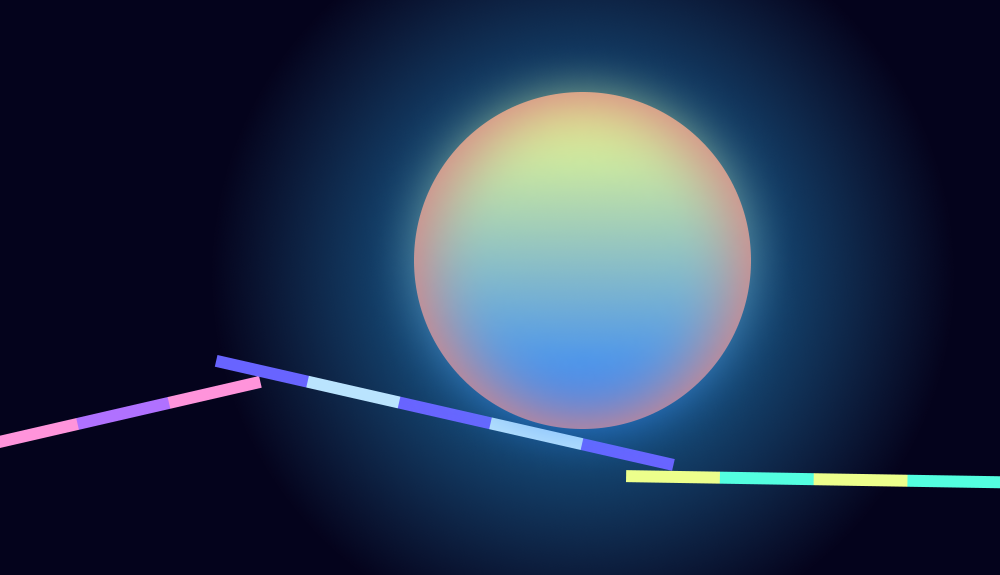
In the early 20th century, society was introduced to the automobile. Initially a novelty, cars rapidly became essential to everyday life â altering landscapes, economies, and behaviors. However, with this innovation came new risks: accidents, environmental impact, and infrastructural challenges. It wasn’t long before people realized they needed comprehensive traffic regulations and safety standards.
Today, we are at a similar crossroads with artificial intelligence, especially large language models (LLMs). In less than a year, AI has gone from âfuturisticâ to âessentialâ, and not without its perils â privacy infringement, bias, and ethical implications. So, the need for AI guardrails is as crucial as traffic regulations. Theyâre not an add-on; theyâre table stakes.
- Artificial Intelligence (AI), specifically large language models (LLMs), have rapidly become essential but also pose risks such as privacy infringement, bias, and ethical concerns, emphasizing the need for AI guardrails.
- AI guardrails are crucial for enterprise AI, as LLMs have intrinsic limitations that can lead to inaccuracies, biases, and data breaches.
- Enterprises face significant risks when using LLMs due to data sensitivity, compliance requirements, brand reputation concerns, financial implications, and ethical considerations.
- AI guardrails help ensure data security, regulatory compliance, brand reputation, and ethical AI usage by mitigating biases, providing verification systems, and ensuring alignment with enterprise values.
- When selecting an LLM for enterprise operations, it’s essential to assess safety, security, compliance, and output quality through a comprehensive evaluation process.
- Writer offers a full-stack approach to AI guardrails with built-in compliance, factual accuracy, and brand alignment features, addressing enterprise pain points and demonstrating successful implementations in various industries.
The importance of AI guardrails in enterprise AI
Traditionally, artificial intelligence has been built on rules, rubrics, or algorithms. LLMs mark a significant advancement with their real-time recognition, learning, and iteration capabilities â making them a building block for enterprise knowledge systems.
LLM-powered chatbots, language translators, and virtual assistants have already become commonplace in enterprises. As the technology develops, autonomous systems become likely use cases.
But since theyâre intrinsically probabilistic, LLMs let bias and accuracy slip by. So you canât just take any business problem and throw any LLM at it. For an enterprise solution, you need to make careful considerations like:
- Is what the system is outputting safe for the user?
- How is bias being mitigated?
- How do we ensure the output is factually correct?
- How do we ensure the LLMâs output accurately reflects our brand?
- How do we ensure that the output follows legal compliance?
A large language model cannot navigate these concerns on its own.
Risks and challenges of implementing AI in enterprise environments
If left to its own devices, an LLM poses expensive risks to enterprises. Hereâs why:
- Data sensitivity
Enterprises often handle sensitive data, including personal information, proprietary data, and intellectual property.
For every 10,000 enterprise users, 22 post source code on ChatGPT every month, according to a 2023 Netskope study. Regulated data, intellectual property, and passwords follow close behind at four, three, and two posters per month. Tallying up the average prompts per user, thatâs 184 instances of possible data breaches, disclosures, and regulatory leaks for enterprises.
AI guardrails prevent this data from being misused or exposed.
- Compliance and legal requirements
Enterprises are subject to stringent regulatory compliance standards. The White House Executive Order on AI enforces even more thorough protocols for the use of AI. These include:
- New Standards for AI Safety and Security: Developers of powerful AI systems must share safety test results and critical information with the U.S. government.
- Protecting Americansâ Privacy: Federal support for developing and using privacy-preserving AI techniques.
- Advancing Equity and Civil Rights: Guidance against using AI algorithms for discrimination in various sectors.
- Standing Up for Consumers, Patients, and Students: Responsible AI use in healthcare and education to protect consumers and enhance benefits.
- Supporting Workers: Developing best practices for AI’s impact on labor and workforce training.
The European Union’s provisional agreement on the Artificial Intelligence Act almost mirrors the White House AI Executive order, both setting rigorous AI standards:
- Banned applications: Prohibits AI for biometric categorization, social scoring, and exploiting vulnerabilities.
- High-risk AI obligations: Mandates impact assessments for AI affecting health, safety, and fundamental rights.
- General AI Guardrails: Requires transparency and compliance for general-purpose AI.
- Innovation and SME support: Promotes regulatory sandboxes for AI development.
- Sanctions: Sets fines for non-compliance based on company size and infringement severity.
LLMs designed for general, commercial use often produce outputs that do not adhere to industry-recognized security and privacy standards (even when prompted otherwise). Therefore, industries under strict codes of conduct like healthcare, banking, and finance are subject to even stricter scrutiny.
AI guardrails and built-in verification systems ensure that enterprise AI systems adhere to industry-recognized privacy laws and security regulations, reducing the risk of legal penalties.
- Brand reputation and trust
Brand and reputation are major enterprise differentiators and take decades to build. Missteps in AI usage can burn all of it to the ground.
For example, The Guardian recently blamed Microsoft for adding an insensitive AI-generated poll to one of its news articles. The Guardian claims that it suffered âsignificant reputational damageâ when the experimental generative AI feature invited readers to vote on the reasons for a womanâs death (murder, accident, or suicide). Hosts of readers expressed disappointment and rage at the audacity of the ask.
The Guardianâs incident is not an isolated one. Amazon recently faced backlash for training Alexa on user conversations â damaging Amazonâs reputation since it suggested a disregard for user consent and data security. This undermined public trust in Amazonâs commitment to ethical AI practices.
AI guardrails help prevent these incidents. Enterprises can maintain and enhance customer trust by adopting AI systems that are ethical, transparent, and trainable on the enterpriseâs messaging.
- Financial and legal implications
Even though all enterprises face financial implications due to misuse of AI, these industries face a far greater risk:
- Human resources and recruitment: risk discriminatory hiring practices, violating employment laws, and damaging company reputation.
- Healthcare orgs are susceptible to misdiagnoses that could lead to malpractice suits and patient harm.
- Finance and banking are vulnerable to unfair lending practices or inaccurate risk assessments, leading to financial losses and regulatory penalties.
Also, since LLMs canât self-regulate, they canât ensure their output is under a certain grade level. This can make critical healthcare and financial information inaccessible, undermining consumer trust.
- Ethical considerations
There’s growing awareness and concern about the ethical implications of AI. In fact, 52% of Americans say that they feel more concerned than excited about the increased use of AI.
Nick Bostrom, the director of University of Oxfordâs Future of Humanity Institute and author of Superintelligence: Paths, Dangers, Strategies, emphasizes the importance of ensuring that AI systems align with human values and goals. In his book, he states, “The alignment problem is the task of building AI systems that reliably pursue the objectives we intend, even if these objectives are difficult to specify.”
Generative AI and LLMs are not suited for environments that typically require human values such as emotional intelligence or compassion.
âGenerative AI is typically not suited for contexts where empathy, moral judgment, and deep understanding of human nuances are crucial,â says Saurabh Daga, associate project manager at GlobalData.
AI guardrails ensure that AI usage is restricted or limited in circumstances where emotional intelligence is critical or ensure AIâs alignment with ethical standards and societal norms.
Methods and approaches for ensuring AI guardrails in enterprise systems
Selecting an LLM for enterprise operations is a big task. Besides ensuring that the systems are legally and ethically compliant, executives need to understand the extent, security, and recovery planning procedures.
Here are ten questions you should ask to ensure AI guardrails are in place:
Safety and security
- What safeguards exist for personal data?
- Does your system support the redaction of sensitive data?
- What measures do you have for data anonymization, encryption, and access control to protect sensitive information?
- What measures prevent malicious actors from injecting harmful prompts or code?
- What safeguards and monitoring mechanisms identify and mitigate jailbreak attempts?
LLM output compliance
- What mechanisms mitigate bias and inappropriate content?
- Is there any automated post-output filtering you should be aware of?
- Do you have any industry standards, benchmarks, or thresholds you adhere to for toxicity detection? How are these updated and refined over time?
- What sources of data bias exist, and what mechanisms and methodologies do you have for training and fine-tuning the AI model to manage bias?
- Are there other types of content or policies you can implement to identify and warn/block inappropriate or harmful content?
Want a detailed breakdown on LLM vendor evaluation? Download our complete checklist.
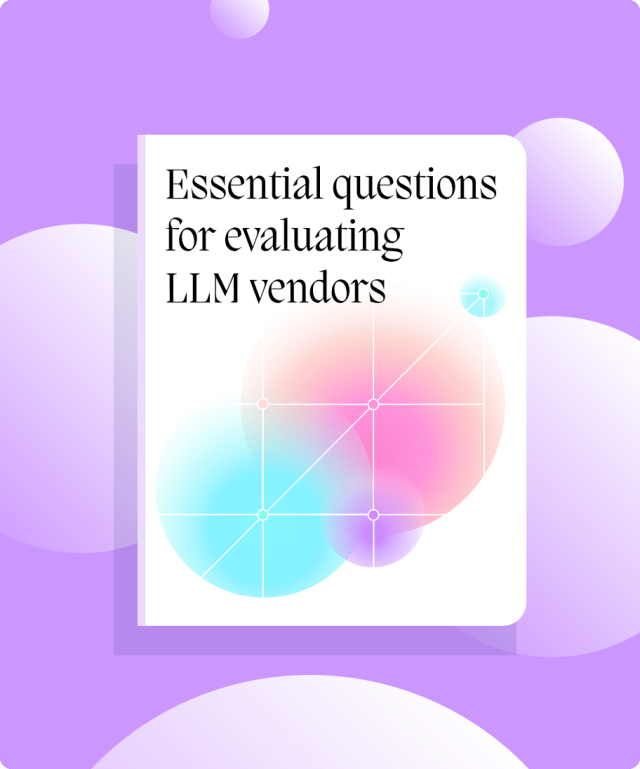
Download the checklist
The Writer approach to AI guardrails and addressing enterprise pain points
Our AI guardrails had been in development for three years prior to AI’s mass commercial availability. This made us deeply aware of how LLMs function â what theyâre good at and what they canât do. To circumvent and mitigate LLM loopholes such as hallucinations, biases, and mathematically lax output, enterprises need a full-stack solution. Not just a set of tools they can plug and play.
We realized how important AI guardrails are for enterprises, and we decided to build these layers at the very beginning. Itâs also what gets us through the door on compliance.
We’ve built tools and applications that sit on top of LLMs, which we customize for enterprises’ unique requirements.
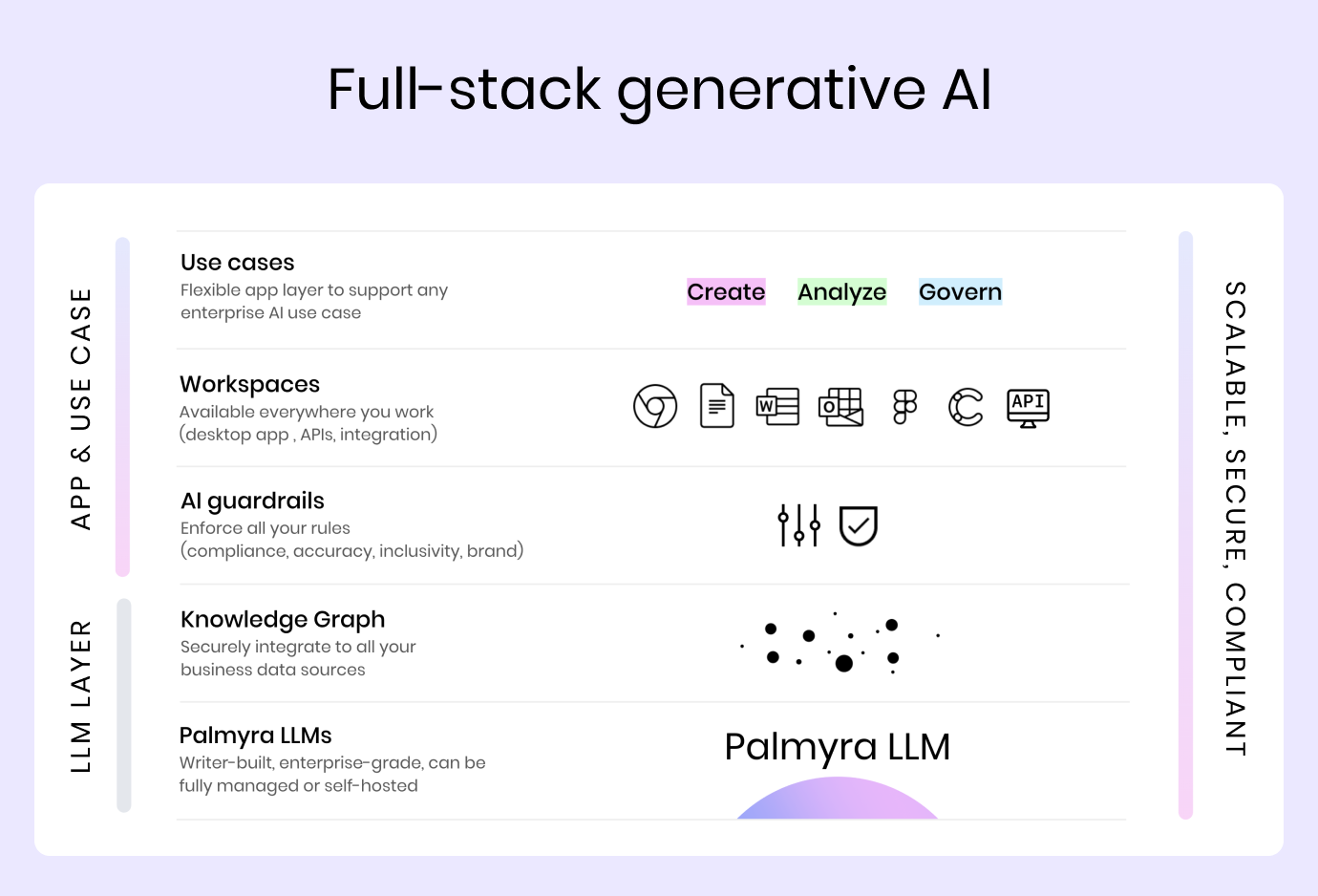
AI guardrails at Writer include:
- Compliance: We flag language that runs afoul of legal and regulatory rules, including incorrect terminology and statements, and have features for data loss protection, global compliance, and plagiarism detection.
- Factual accuracy: We detect and automatically highlight claims that need to be fact-checked.
- Brand alignment: We ensure all work reflects your brand, messaging, and style guidelines, and uses inclusive and unbiased language.
We use a combination of rules, deep learning models, rules-based models, transformer-based classifier models, and third-party APIs. For example, we implement claims detection using transformer-based classification models and run inclusivity checks using rules-based models.
Case studies: successful implementation of AI guardrails in enterprise AI solutions
While every industry is hyper-focused on different AI guardrails, two use cases surface frequently.
Case 1:
At financial and healthcare companies, ensuring communications read at a certain grade level is table-stakes for regulatory compliance. Adhering strictly to an accessible grade level is crucial for knowledge transfer, and also builds trust and ensures clarity in life-or-death situations.
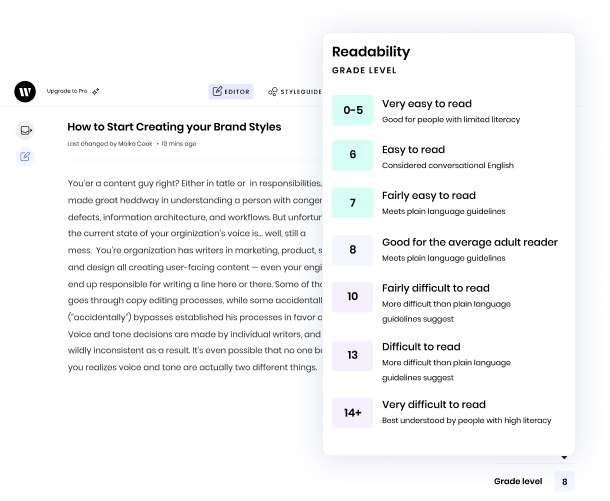
Healthcare and financial companies like Vanguard, Real Chemistry, and CoverMyMeds use Writer to create content that communicates the expertise of their team while following regulatory requirements.
As a result, they can personalize plans for every financial client and patient and identify opportunities for improving cross-channel patient care.
Case 2:
Ecommerce companies need to maintain accurate character counts for product descriptions since it directly impact sales.
However, traditional large language models (LLMs) struggle with self-monitoring character counts. This is because LLMs process and output information in inconsistent chunks of words known as tokens, making it challenging to generate product descriptions with precise character counts.
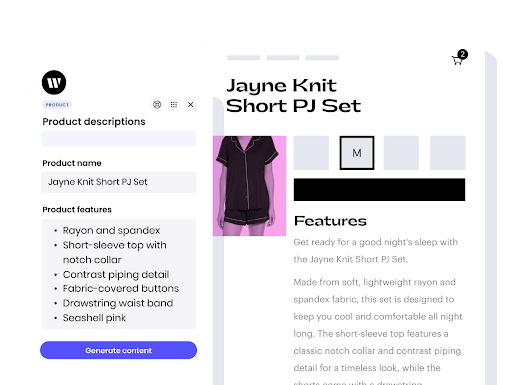
To overcome this challenge, leading retail companies like AdoreMe rely on Writer to optimize their product descriptions. With Writer’s AI-powered guardrails, these companies can ensure consistent character counts without manual intervention.
Writer has developed a range of AI guardrails, microservices, and applications specifically tailored for enterprise use cases. Addressing character counts is one of the significant functionalities offered by Writer, enabling businesses to save valuable time and resources.
Considerations for building safety into generative AI solutions
As AI innovation and adoption grow at breakneck speed, enterprises need to ascertain three things:
- Output needs to originate from enterprise data. Your LLM canât hallucinate or exaggerate.
- Your LLMs need to make full use of proprietary enterprise data correctly and safely.
- LLMs need to ensure that data, inputs, and outputs are accurate and referenceable.
AI guardrails can’t be an add-on or a feature, they need to be foundational for both development and deployment. Careful LLM orchestration and integration with enterprise data assets is what separates a full-stack solution from an off-the-shelf plug-in.
Read our complete guide on generative AI governance for a holistic overview of responsible AI adoption.