AI in the enterprise
– 11 min read
The future of enterprise AI: A fireside chat with Writer CEO, May Habib
WEBINAR RECAP
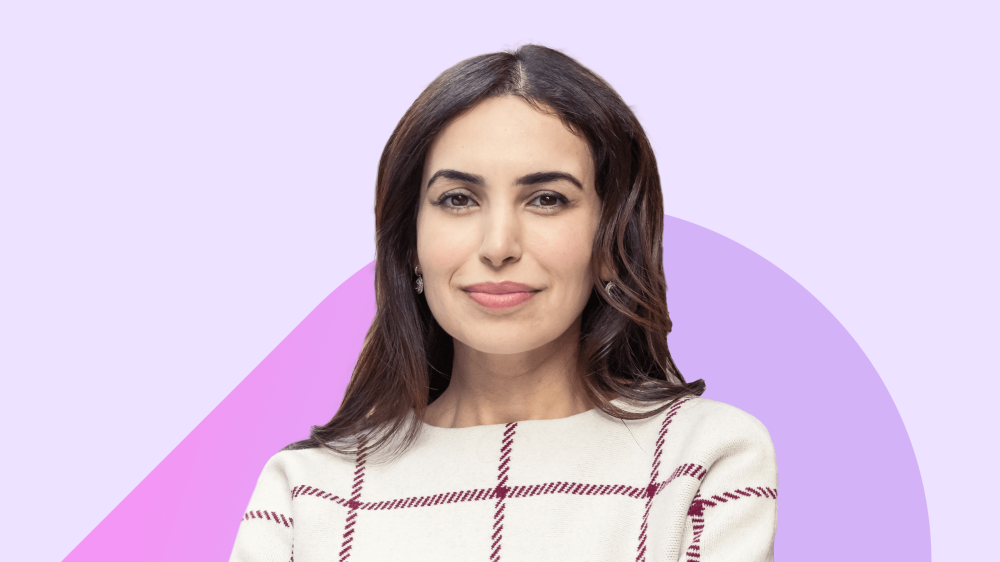
In a world where self-driving cars and autonomous assistants are swiftly transitioning from futuristic dreams to everyday realities, staying ahead of the curve has never been more important. Blink, and you might find your enterprise left behind as competitors adopt these transformative technologies. We recently hosted an insightful fireside chat with Writer CEO, May Habib, and CMO, Diego Lomanto to discuss the future of enterprise AI.
Learn about Writer’s journey from AI upstart to a generative AI powerhouse and how AI is set to become the backbone of mission-critical enterprise workflows — raising $200 million in our Series C. Habib shares her vision for developing intelligent, accurate, and trustworthy AI systems — giving us a glimpse into the near future.
- Our Palmyra LLMs are top-scoring, faster, and more cost-effective than other models, and are fine-tuned for specific industries.
- AI Studio is our platform for scaling the development and deployment of AI applications, enabling “builders” within companies to develop and manage AI-driven applications.
- We define agentic AI as models capable of autonomous action, while AI assistants are dialogue-driven tools tied to data, and AI apps are focused, task-specific tools.
- The most impactful use cases for generative AI vary by industry, and tailored solutions are key to delivering real value.
So, how did we get here? From machine translation to generative AI innovation
Many people are amazed at how much Writer has achieved in just four short years. But much of our success can be attributed to the heritage and the legacy of our founders. Habib and Waseem AlShikh met over a decade ago, a connection that began when Habib reached out to AlShikh on Twitter, intrigued by his work on statistical machine translation problems.
They hit it off immediately and decided to start a machine translation company, Qordoba. Several years later, a group of scientists at Google published a groundbreaking research paper titled “Attention is All you Need.” It introduced a revolutionary deep learning architecture: the transformer.
“What folks sometimes forget, or don’t know, is the Attention paper and the researchers that had those incredible algorithmic breakthroughs really were working on machine translation problems,” Habib says. “And so we were in the heart of it. And it was very, very hard to unsee what we saw.”
It was a difficult decision at the time, but they decided to pivot and start Writer, officially launching in March 2020 with a Series Seed.
The decision to build our own LLMs
Habib and AlShikh recognized early on that building their LLMs in-house was a strategic and necessary decision. You can liken our full-stack approach to Tesla’s — where every layer of the technology is developed internally, ensuring all parts work together seamlessly. Transparency, security, and reliability are the primary reasons for this approach — essential requirements for enterprise customers.
Using open-source or closed-source models would have made it nearly impossible to meet these stringent enterprise needs, especially regarding data retention and residency policies. By developing our own LLMs, we could tightly integrate them with our RAG approach, achieving a level of accuracy and quality that wouldn’t be possible with third-party models.
“We’ve used LLMs to solve all sorts of problems — from eval to guardrails to autonomous action to the very big breakthrough we’ve had on the transformer architecture,” Habib explains. “All those things would have been impossible if we didn’t see ourselves as a state-of-the-art LLM company.”
What makes Writer unique
Writer is the full-stack generative AI platform for enterprises. Our integrated system of LLMs, graph-based RAG, AI guardrails, and development tools makes it easy for organizations to deploy AI apps and workflows that deliver impactful ROI.
Palmyra LLMs
There’s no such thing as an LLM-agnostic application, which makes maintenance efficiency crucial for multimodal programs. We’ve developed a family of models that are backward-compatible, so customers don’t notice any degradation when upgrades are made.
Palmyra, our family of transparent LLMs, is top-scoring on key benchmarks like Stanford HELM, faster and more cost-effective than other models, and fine-tuned for specific industries. (Check out Palmyra Med and Palmyra Fin.)
This isn’t a one-time achievement — we’ve been at the forefront for the past two and a half years. What drives this success is our dual focus on building state-of-the-art models internally and continuously advancing our capabilities. We excel in data, algorithms, and compute, thanks to our talented team and unique data approaches.
“We’ve been doing this literally longer than just about anybody,” Habib says. “And the ability to maintain, that is really just a matter of strategy, right. We have to remain state-of-the-art and push the boundaries on data and algorithms and computing.”
We’re also not focused on simply throwing more GPUs and scaling up in size. Instead, we prioritize advancements in architecture and the way models are built.
A graph-based approach to RAG
We recognized early on the potential for transforming how LLMs connect to data. LLMs need to access important company data in real time. This is particularly crucial for customers in sectors like financial services and healthcare, where accuracy is paramount.
We pioneered a graph-based RAG solution, becoming one of the first to offer a commercial implementation of this approach. This method involves training LLMs to create a graph-based structure of nodes and edges on the data used for retrieval and augmented generation. The process is continuous and real-time, seamlessly integrated into our platform.
Users can create a data repository and connect it to a use case without ever seeing the word “RAG.” Instead, they interact with data connectors, APIs, file uploads, and syncs with platforms like Google Drive or SharePoint. Under the hood, we handle all the preprocessing, ensuring that users receive accurate answers with detailed citations in a low-maintenance, user-friendly manner.
AI Studio
AI Studio emerged from our internal frameworks and interfaces designed to help build highly specific applications for our customers. Over a couple of years, we learned how to effectively wrap LLMs with business logic and connect various tasks in workflows to these models, data, and examples.
We realized that as our customers built dozens and hundreds of applications, they could significantly contribute to this process. So, we introduced AI Studio, encapsulating these learnings and providing a platform for scaling the development and deployment of applications.
“The hallmarks of a Writer implementation is everything you build will go to production, will have an impact, will get adopted, and will change your business,” Habib says. “And AI Studio is a big way to how we figured out how to scale that. The customers love it because the data scientists become builders, the product people become builders, the business people become builders.”
Right now, there’s no better way on the market to maintain the logic, data, and examples for mission-critical applications than with AI Studio. And AI Studio is a big part of our next phase of development. We’re building agentic functionality that’ll give enterprises more automation and agency by identifying and empowering ”builders” inside the company who can develop and manage AI-driven applications.
What we mean by AI agents, AI assistants, and AI apps
With so much terminology floating around and little consensus on what it all means, it’s important to have clear definitions. Here’s how we define a few key terms at Writer.
Agentic AI
In the research community, agentic AI means models capable of independent reasoning and action taking. At Writer, we call this autonomous action.
Agents are AI assistants capable of using reason to achieve a goal. Namely, they can:
- Perceive their environment. Example: user inputs (text, image, voice etc.), tools, data stores
- Reason through a plan or sequence of actions to achieve a goal. This could include interacting with other agents, workflows, apps, tools, models, etc. (see definitions below)
- Execute the plan
AI assistant
An AI assistant is a dialogue-driven tool that’s tied to data via a RAG application. It uses knowledge graphs and LLMs to assist with data curation, making it smarter, more maintainable, and scalable for enterprise workforces.
AI app
An AI app is a focused, task-specific tool that processes and transforms data, often accepting unstructured inputs through uploads or free-form entries. Unlike AI assistants, these micro apps typically don’t rely on chat interfaces. They can be composable or one-time applications.
AI workflow
An AI workflow is the stringing together of multiple applications, sometimes with or without a systems integration. These workflows can include autonomous actions (which gets confusing, because this is what others might call agentic AI).
Some killer AI agentic use cases to start with
The most impactful use cases for generative AI vary significantly by industry. Not every vertical is going to benefit from agentic AI — for some, AI assistants provide the most value. A one-size-fits-all approach to generative AI doesn’t work, and tailored solutions are the key to delivering real value.
Pharmaceutical sector
In pharmaceuticals, the top agentic use cases are centered around medical and legal review processes. These are crucial for bringing new drugs to market and ensuring compliance across multiple teams.
In medical affairs, it’s AI assistants that are key to empowering desk research and supporting researchers. But in commercial affairs, the focus shifts more towards agentic functions, such as managing market entries for new products.
Investment banking
In investment banking, there are strong use cases for autonomous action, particularly in maintaining and analyzing target lists for M&A transactions.
Consumer packages goods
For CPG companies, the agentic use cases are robust when it comes to launching new SKUs, while product development benefits more from AI assistants for deeper, more specialized tasks.
How humans fit into the agentic equation
We’re putting a lot of trust in AI, so where do humans fit into this? There are two main areas: inspection and architecture. Inspection involves checking and reviewing the AI’s outputs or processes to make sure they’re correct and meet the goals set. Architecture is about designing and setting up the AI workflow itself, deciding the steps involved and where humans need to step in.
When autonomous action workflows are in place, the human “architects” of these workflows need the flexibility to decide how and where human inspection is required. For example, let’s say in the future you’re using Writer’s agentic workflow to send personalized emails through Marketo. The business user might want to edit the email inline and inspect a few personalized versions — but not all of them — to build trust in the AI’s capabilities.
So, the “architect” of the process would design the workflow with this in mind, creating a step in the process for a business user to “inspect” a few of the outputs.
Habib envisions a future workplace where most jobs involve shaping these workflows, rather than the current hands-on, manual input, and inspection processes. AI will handle the repetitive tasks, freeing us to focus on higher-value, strategic work.
“If we’re rebuilding the flow for how we create investor reports, that is a very exciting architect type of position,” Habib says. “You are architecting the flow of human work and where you want your colleagues’ attention to go in this new generation of AI driven workflow.”
The UX patterns that’ll emerge from this have yet to be discovered, so a lot of these are hypotheticals. But they’re on the horizon, and we’re all part of that future.
What’s next in enterprise AI
Folks need to approach enterprise AI with a thoughtful and user-centric mindset. Forcing AI tools, like chatbots, on employees without considering their actual needs and productivity leads to burnout and disillusionment.
Our approach to shattering the disillusionment with generative AI is to go to a customer and show an explosive show of value really quickly, earning everyone’s trust. And these aren’t low-stakes use cases — they’re powerful applications. We aim to achieve this in about 10 to 12 weeks.
If it takes six to 12 months and people are still asking about the ROI of generative AI, then something has gone wrong.
“Architecture matters in generative AI and it’s what allows us to show that value so fast,” Habib explains. “But this is the most transformative technology that’s ever been invented. If you’re asking about the ROI of it, then you have not done it right and we’d love to help.”
Schedule a demo with Writer to see how we can help embed generative AI into any of your processes and help you achieve ROI quickly.
Watch the full recording of our fireside chat: The future of enterprise AI: A fireside chat with Writer CEO, May Habib