AI in the enterprise
– 11 min read
The five must-haves of trustworthy generative AI
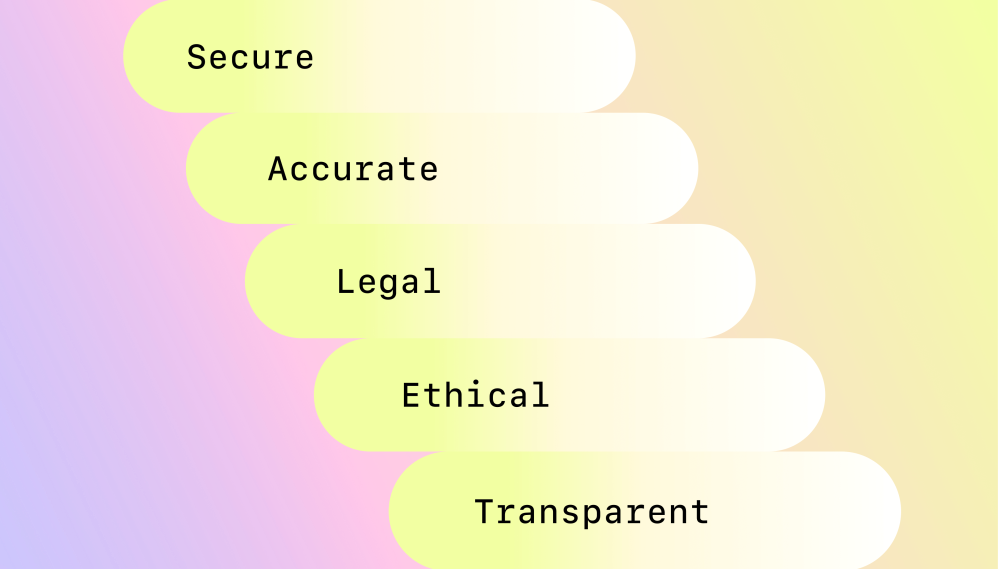
Deploying AI in an enterprise environment is a marker of growth and efficiency.
According to IDC’s Worldwide C-Suite Survey 2023–2024, more than half of C-suite executives feel that generative AI is top-of-mind for new investment, and 87% say they are at least exploring potential use cases.
While this sets the stage for executives to experiment, it also pressures them to prove their investment’s merit — quickly.
And what falls through the cracks? Due diligence on reliability. Trustworthy AI ensures that while executives experiment with use cases, there’s no long-term detriment from aggressive implementation or diverse user inputs.
But what makes generative AI trustworthy and responsible?
In this piece, we talk about what enterprises define as ‘trustworthy’, the five must-haves for AI solutions to qualify, and advanced enterprise requirements.
- Trustworthy AI prioritizes privacy, safety, transparency, and responsible design.
- Key requirements for trustworthy generative AI include security, accuracy, legal compliance, ethical considerations, and transparency.
- Security features should be built into the infrastructure and include data confidentiality, flexible deployment options, comprehensive data access control, and data privacy agreements.
- Accuracy is crucial, especially in specialized industries like medicine, finance, or law, and should be ensured at every step.
- Ethical considerations involve creating a structured hierarchy of AI ethics, correcting for biased inputs, promoting fairness, and preventing misuse.
What does it mean for AI to be trustworthy?
Trustworthy AI means a commitment to privacy, safety, transparency, and responsible design. At its core, it prioritizes the protection of individual privacy and transparency. Every user understands how their data is used, and owns their data. Secondly, trustworthy AI has safeguards to prevent malicious actors from injecting harmful prompts or code. Thirdly, trustworthy AI walks the fine line between continuous self-learning and improvement, without using customer information as a knowledge base.
There are five key requirements to run a trustworthy generative AI program:
Secure
Security in AI mirrors the CIA triad: data confidentiality, integrity, and availability. A trustworthy ‘data confidentiality and integrity’ policy means that customer data never becomes a learning path for AI, even if it’s anonymized.
AI has to have security principled into the deep learning mechanisms — built in, not bolted on. Unlike add-on software where you can check a box to share certain permissions, AI needs security features baked into the infrastructure in four key ways:
- Customer data isn’t stored or used to train or improve language models: this has to be architected into the model — not toggle-able.
- Flexible deployment options (multi, single-tenant, and cloud and on-prem): This allows you to choose a suitable hosting environment for your security, privacy, and operational needs.
- Comprehensive data access control: This gives you the option to build access control and limit sensitive data to authorized personnel.
- Data privacy agreements: Your AI vendor should offer data privacy agreements and be willing to sign data privacy agreements that come from customers.
Accurate
According to multiple studies, including a 2023 report by Stanford, accuracy in general-access AI models like ChatGPT has wavered — and steeply fallen. Initially, these models showed promise in understanding and generating human-like text, images, and even music. However, as multiple users crowded the platforms, the outputs started varying — and this variance became concerning. Factors such as data bias, task complexity, and the general-purpose nature of these models have contributed to fluctuating accuracy.
When it comes to specialized industries like medicine, finance, or law, inaccuracy isn’t just a minor hitch. It’s a deal-breaker for AI adoption. If the output isn’t accurate, it’s essentially redundant. It’s conjecture at best, and a lawsuit-attracting hallucination, at worst.
Even sectors that might not be penalized for inaccurate data and content stand to lose customer trust and reputation points. What’s worrying is that nearly 9 in 10 tech leaders believe it may not be possible to know if their company’s AI output is accurate, according to a survey by Juniper.
Trustworthy generative AI ensures accuracy at every step — aiding internal teams with research or customer-facing teams with chatbots and outgoing messages. It gets facts about the world and your organization right.
For example, Commvault had to make the most of their resources in the face of a recession. Each sales or marketing message needed to accurately reflect the brand, without someone having to spend hours crafting it. With the Writer Knowledge Graph, our graph-based RAG solution, they built digital assistants that enabled salespeople by giving them accurate, on-brand insights on objection handling, competitive differentiation, and personas — in real time.
Legal
Legal compliance is a cornerstone of trust — not only in terms of privacy laws, but also:
- Use of content: A responsible AI vendor adheres to copyright laws and ensures that all generated content respects intellectual property rights and doesn’t infringe on existing copyrighted materials.
- Security architecture: To protect sensitive information, the architecture should be designed to safeguard confidential data. For example, Writer employs a model where sensitive data never enters its system in a manner that could be traced back to any individual or entity. This sets it apart from systems where data may be anonymized yet still be linked to customer identifiers.
- Legal considerations of outputs: AI models often produce biased outputs because they’re trained on general data, which is often discriminatory. Trustworthy AI has an overcorrection mechanism designed to identify and correct bias to ensure all outputs are fair and equitable.
Carta, an equity management company, needed an AI solution that was legally bulletproof. Since they serve the venture capital, tax, and compensation industries, security architecture and compliance were non-negotiable for them. Their GTM team built a proof-of-concept with Writer for a generative AI that would aid their new product-suite rollout. They ultimately adopted it company-wide because it assisted their demand-gen, product, and marketing teams, in a legally safe manner.
Ethical
Ethical guidelines are flat and ambiguous. For instance, while many guidelines advocate for “fairness” and “non-discrimination,” they rarely detail how to operationalize these principles in algorithmic decision-making processes or data-handling practices.
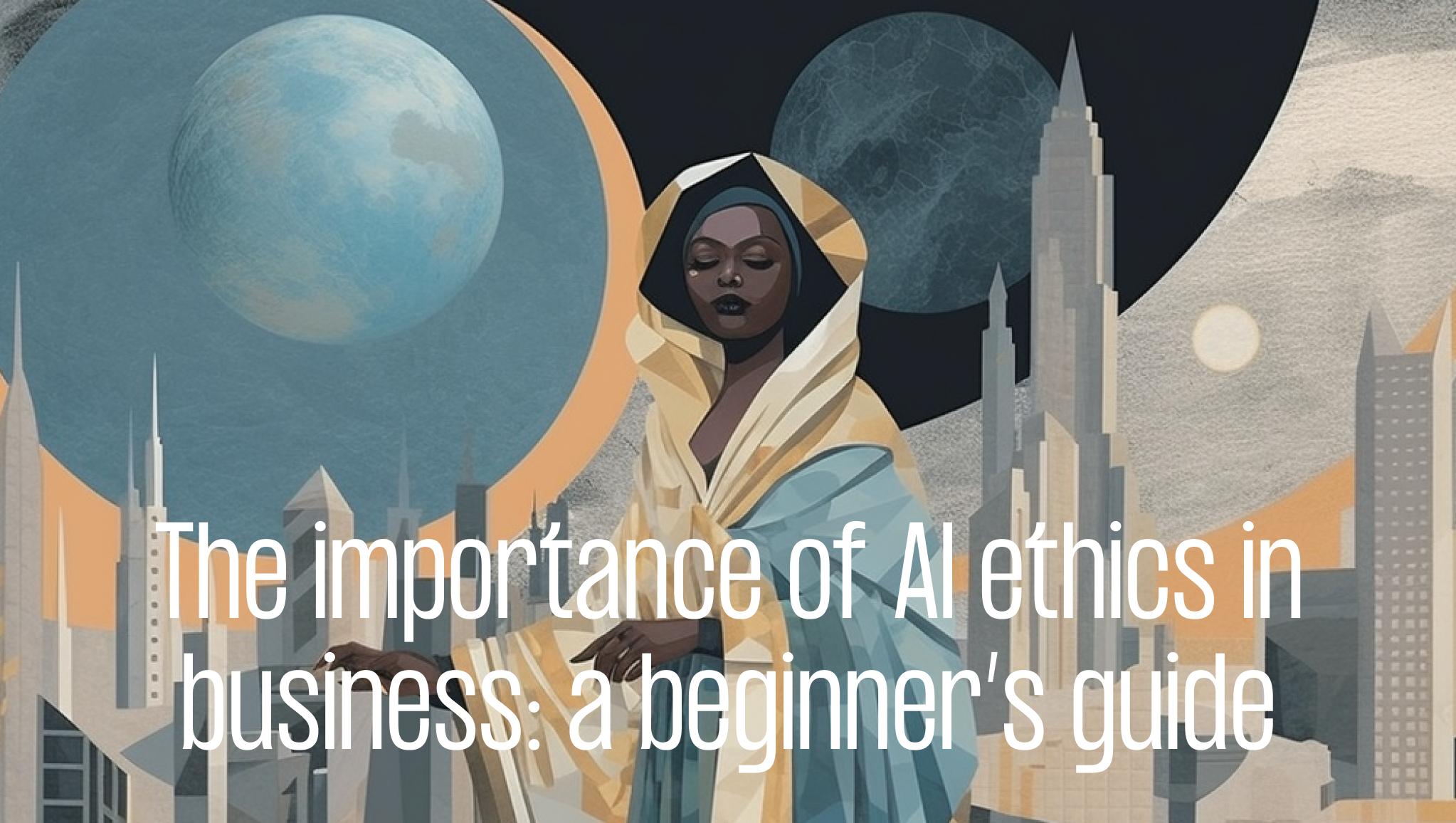
It’s up to enterprises and partnering AI vendors to translate these broad ethical considerations into concrete, actionable policies and practices.
This involves creating a structured hierarchy of AI ethics, including but not limited to:
- Correcting biased data: If we want people to trust artificial intelligence (AI), we need to make sure that the information it uses is fair and accurate. This means getting rid of any biased sources before they can cause problems.For example, the Writer team uses preprocessing techniques, such as removing sensitive content or flagging potential hotspots for bias, before the data is used for training. This further mitigates bias by adding layers of scrutiny and control, both algorithmic and human, on the data used for training.
- Fairness to employees and contractors: Trustworthy AI systems should include features that promote equity, such as algorithms that support unbiased decision-making in HR processes like hiring, promotions, and performance evaluations.
- Misuse: The generative potential of AI also makes it a prime candidate for misuse. Deepfakes and unauthorized duplications are prime examples. A trustworthy model should implement stringent content guidelines and use advanced detection algorithms to identify and prevent deceptive or harmful content.
For example, Intuit needed their messaging to be inclusive across the organization, but they didn’t have enough editors or writers to draft or cross-check every single item. They built style guides with Writer which had revised vocabulary and used the platform API to explain the changes to over a 1000 users across six functions. They updated 800 terms across the terminology, even those that weren’t explicitly problematic but had biased roots, like “master admin” and “white-glove service.” And they built a Writer-powered bot to recommend alternative, unbiased language to employees engaging in workplace communications in Slack.
Learn more about mitigating AI bias and the importance of training data for successful generative AI adoption in the enterprise.
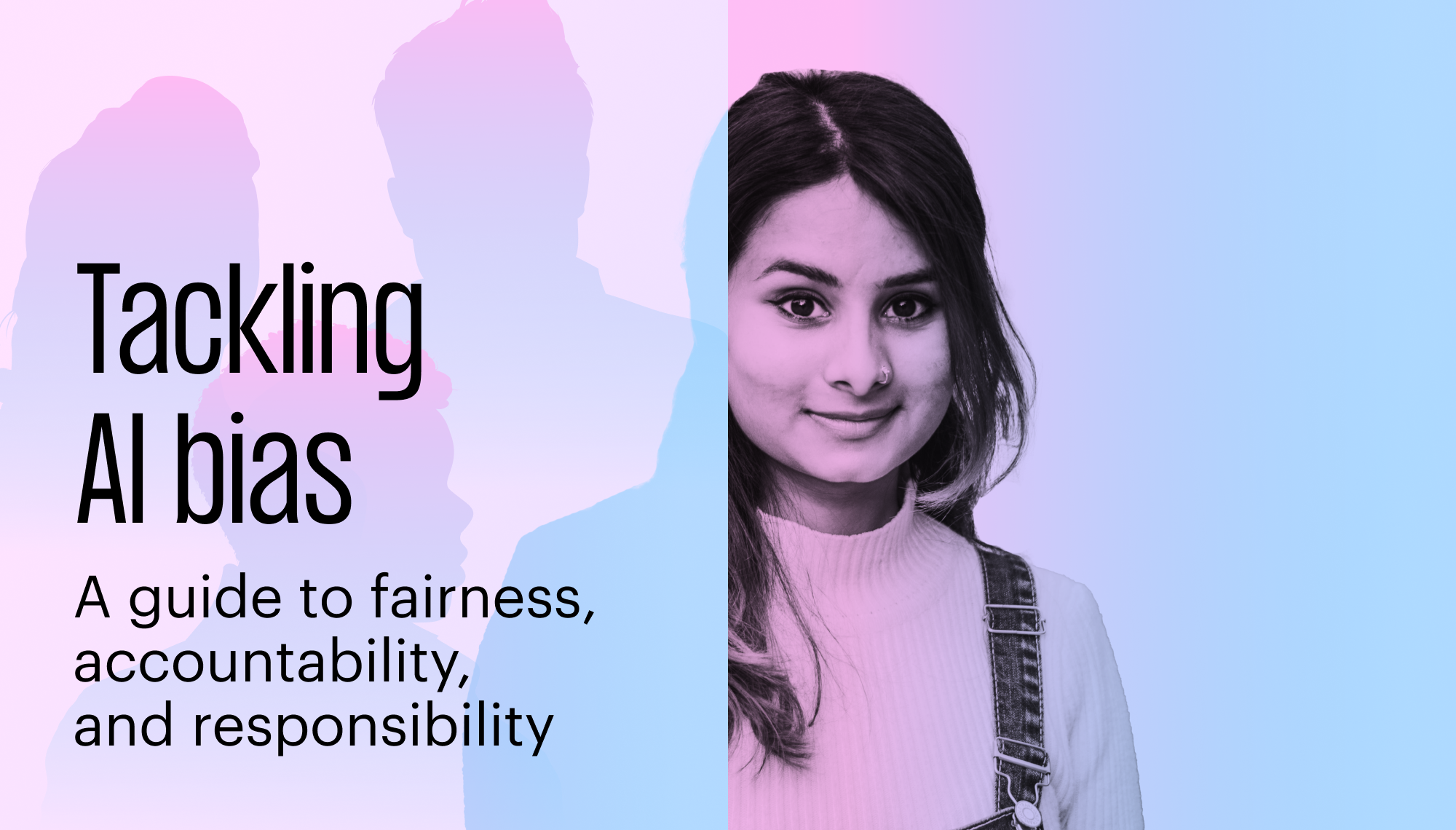

Transparent
Transparency in generative AI goes beyond providing references and trickles down to the molecular structure of the model — creating a two-pronged challenge. First, deep learning mechanisms create a black box where you can’t trace the origins of an output. This means that stakeholders and legal departments can’t perform audits on the system — opening themselves up to liability. Second, the complexity and scale of training data for an LLM can obscure how information is weighted and interpreted. This means that even if evaluators manage to identify sources, they can’t distinguish an influential statistic from an illustrative one.
To evaluate the transparency of your AI model, ask your vendor:
- What is the corpus of data used to train the model?
- How are you measuring the accuracy and quality of your AI outputs?
- What kind of developer resources have you scoped to meet your specific needs and enterprise requirements? Have you scoped out how long it’ll take to build a proof of concept (POC)?
At this stage, you should ask for a comprehensive support system that includes dedicated implementation, account management, user training, technical support, AI program management, and onsite reviews.
- What’s your AI policy around using LLMs that train on copyrighted materials?
Often, public-access AI solutions accidentally circumvent privacy regulations in the name of transparency. For example, they might not fully anonymize data in AI models and create a potential re-identification risks from training datasets, research by KPMG, explains. Truly trustworthy AI, such as the Writer Knowledge Graph, ensures transparency while respecting customer privacy by strict data anonymization, encryption protocols, and output screening.
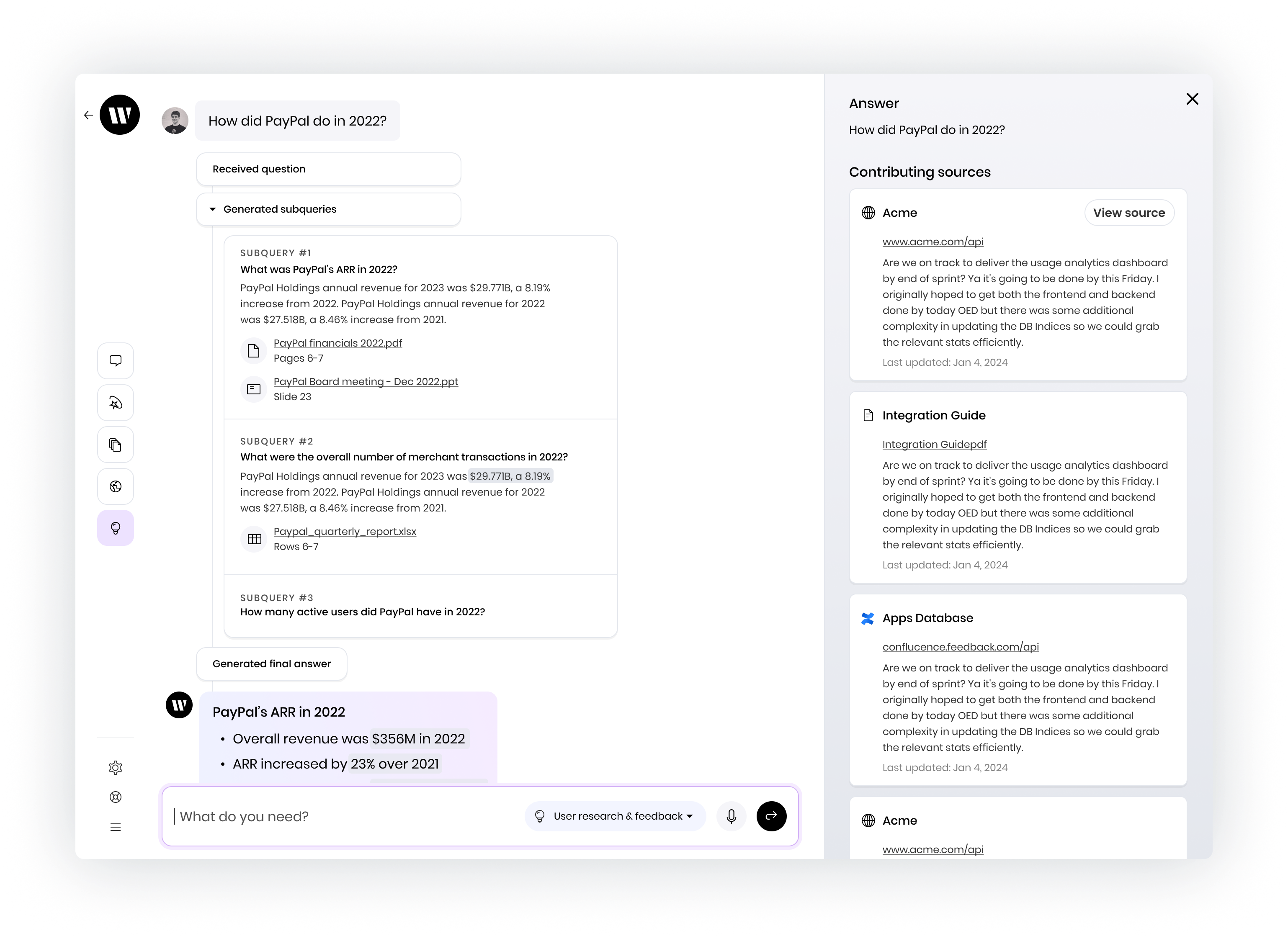
Enterprise considerations for trustworthy AI design
The five must-haves for trustworthy AI also have implications for the architectural and operational design of AI systems within enterprises. Specifically, these considerations pivot around the need for a full-stack approach and the nuances of training Large Language Models (LLMs).
- Full-stack platform approach: To build a high-quality AI solution for businesses, you need a complex and reliable system that includes language models, company-specific knowledge retrieval, AI guardrails and a composable UI. Building an in-house solution that meets benchmarks for reliability and accuracy is a considerable and costly challenge. Since implementing generative AI should be a sooner-rather-than-later decision and since building is so resource-intensive, the best option for most enterprise companies is to partner with a vendor like Writer that empowers you with its existing technology and works with you to build custom solutions unique to your business needs.
- How LLMs are trained: An important question to ask is if the models are trained on information closely related to the field they’re used in. Most of the models available to everyone aren’t fine-tuned to specific industries or situations. They don’t have the specialized skills that models like the Writer-built family of Palmyra LLMs have.
- Tuned to your specific needs: One-size-fits-all isn’t good enough. For generative AI to achieve efficiency and scale, it must be customized to your business processes and use cases. For the output to have value, it must comply with your legal, regulatory, and brand guidelines.
- Integrated: On their own, LLMs have a limited understanding of your company. For output to be useful, your generative AI platform must reflect your internal data. Using generative AI in a single app or closed ecosystem can’t support the needs of the entire organization. A true AI platform must embed easily into an organization’s processes, tool ecosystems, and applications.
Build a foundation of trust into your enterprise generative AI program
To build trustworthy generative AI, privacy, safety, transparency, and responsible design are essential. It must meet key requirements such as security, accuracy, legal compliance, ethical considerations, and transparency. We can make AI systems safer by: 1. Building security measures into the system from the start. 2. Making sure that the AI system is accurate at every step. 3. Thinking about ethical issues, like biased inputs and people using the system to harm others.
To dig deeper into what makes Writer the trustworthy choice for enterprise generative AI, request a demo.