Evaluating
generative AI solutions
for enterprise
A step-by-step guide
for CIOs
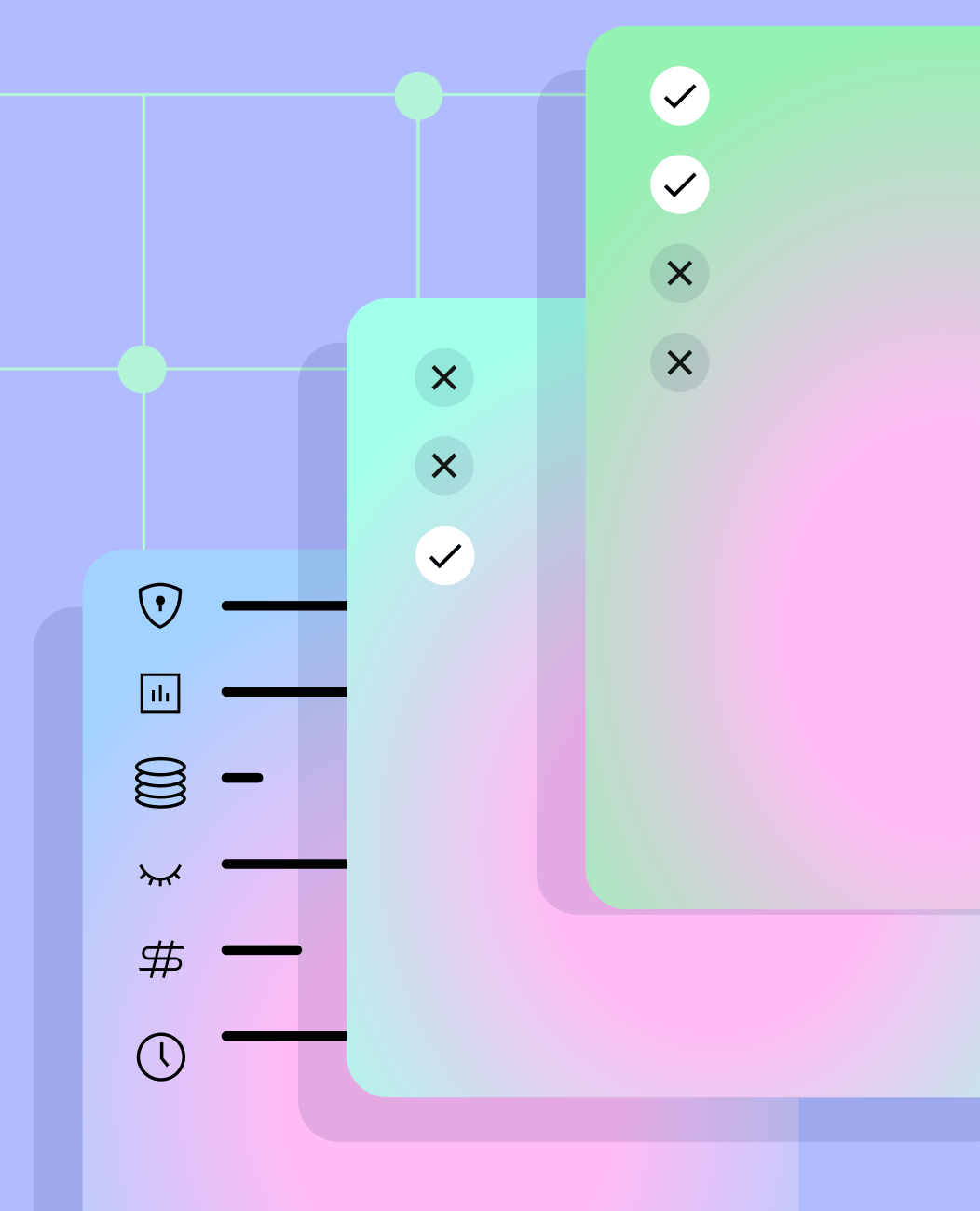
Introduction
Generative AI is fueling a new wave of innovation for enterprise companies. Our recent global survey of technical leaders and decision-makers found that 96% of companies expect generative AI to be a key enabler for their business, with 82% anticipating rapid growth in adoption. If youâre an enterprise technical leader, now is the time for you to prioritize adopting and integrating generative AI into your companyâs overall business strategy. And now is also the time for you to keep an eye on how this technology is implemented to ensure quality and minimize technical debt.
Traditionally, CIOs have been responsible for keeping the lights on, reducing risk, and managing the status quo. But with the advent of generative AI, CIOs understand they have to be more proactive, do more with less, and drive business outcomes at scale. As CIOs adopt this shift, they take on the role of driving transformative progress within their organizations.
Thatâs why weâve created a step-by-step guide specifically for CIOs to evaluate generative AI for enterpriseâ use.
At its core, this guide is designed to empower you as an enterprise technical leader with a roadmap for evaluating generative AI solutions with confidence. Through a comprehensive, step-by-step process, youâll gain the insights needed to make strategic decisions and guide your organization to success. From demystifying the technology and its capabilities to identifying the right vendors and implementing solutions, this guide will equip you with the knowledge and tools you need to navigate the complex world of generative AI. So let’s dive in and discover how generative AI can transform your business and how you can successfully evaluate and integrate it into your enterprise strategy.
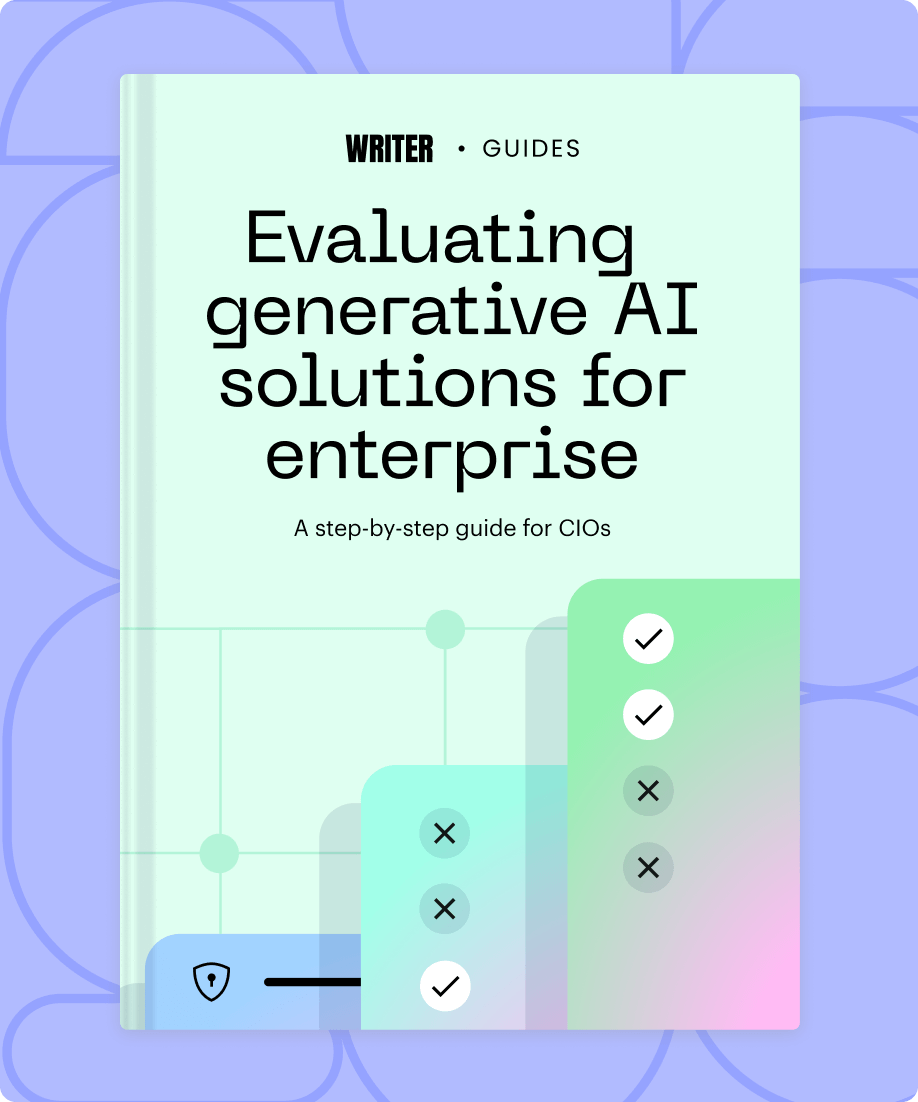
Download the ebook
Understanding the generative AI landscape
Generative AI is an exciting, fast-evolving technology thatâs already changing the way we do business. As youâre no doubt aware, an explosion of generative AI vendors has flooded the market over the past few years.
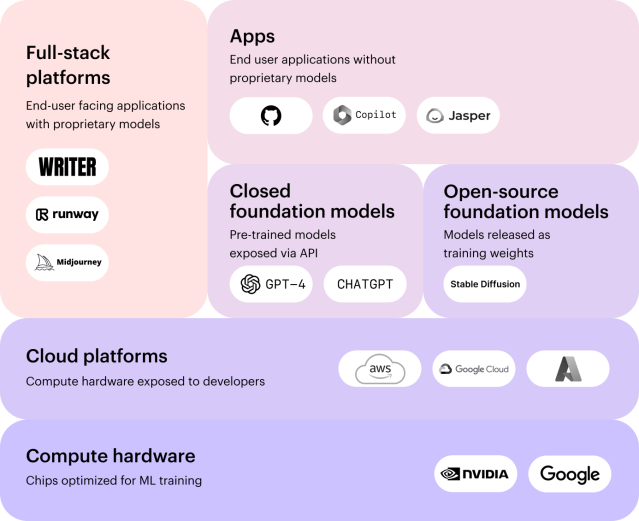
There are three main types of generative AI solutions to consider for enterprise use:
- Custom stacks are built in-house and can be personalized to the exact needs of your organization.
- AI assistants and point solutions are great for incremental productivity and personal work.
- Full-stack platforms support more complex, organization-wide applications.
Benefits and challenges of adopting generative AI solutions
Adopting generative AI solutions offers a host of advantages. It boosts business growth by ramping up production, delivering more insightful analyses, and enhancing the quality of outputs. These improvements can help streamline operations and elevate customer experiences, making everything more efficient and effective. Generative AI speeds up how quickly products reach the market. Plus, it helps make sure that work sticks to brand and compliance rules, cutting down on mistakes or rule-breaking.
But integrating AI into your business isnât easy. It requires a commitment to change at every level of your organization. You need to educate and train your employees on how to use AI and the new processes and technology that it brings. You also need to address any concerns or resistance to AI that might come up. And, if youâre building in-house, you need to invest engineering time and resources to build and maintain your AI stack, which can be a significant commitment.
Going beyond chat bots: full-stack generative AI as a strategic business initiative
If youâve experimented with generative AI tools like ChatGPT, you already know that it speeds up time-consuming, manual work, like generating personalized content and summarizing reports. But chat interfaces built on top of LLMs are only part of the story.
With a full-stack solution, the capabilities go beyond content generation and personal productivity. Retrieval-augmented generation (RAG) delivers company-specific knowledge for customer support, sales, and employee training. AI guardrails drive consistent, compliant outputs for public-facing documents. When integrated with existing workflows, generative AI improves data analysis and operational decision-making. Full-stack generative AI opens the door for enterprises to streamline operations, drive efficiency, and provide outstanding customer experiences.
Our 2024 survey of CIOs and technical decision-makers showed that IT, customer support, and security are the top business areas for generative AI adoption. In the next two years, 97% expect that new teams will be using generative AI. This data shows the need for a shift in focus from AI assistants, which have limited overall business impact, to full-stack solutions that deliver significant, measurable business growth.
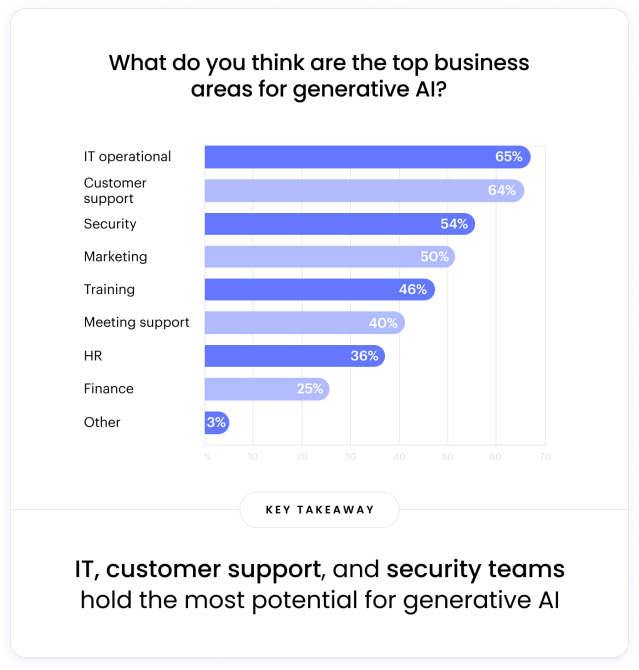
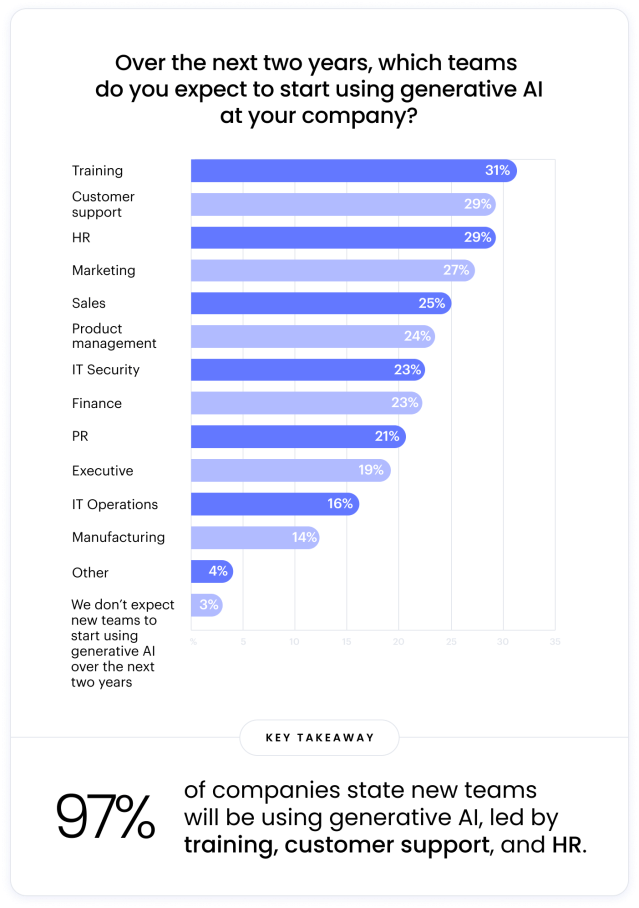
In general terms, hereâs what you need for an enterprise-grade, full-stack AI platform:
- A large language model (LLM)
- A way to connect LLMs to your business data (RAG)
- A way to apply AI guardrails
- A way to build AI apps
- Ways for your people to use the apps
- The ability to ensure security, privacy, and governance for all of it
When these pieces come together, you can use AI to accelerate business growth, increase productivity across every team in your organization, and more effectively govern the data and content your company puts out into the world.
A custom stack will have all these parts, but you’ll have to stitch them together. You get some added flexibility, but at the cost of a higher integration cost and longer time to market.
Weâll cover the technical requirements for a full-stack generative AI solution â and balancing build vs. buy decisions â later in this guide.
Evaluating AI and LLM vendors: a quick framework for CIOs
As you evaluate generative AI vendors for your enterprise solutions, here are the most important factors to consider. By doing so, you can be sure to make the most informed decision and select the vendor that best meets your organizationâs needs.
- Technical feasibility
Assess a vendorâs foundational technology, deployment options and infrastructure support. Understanding a vendorâs technical architecture is critical to ensuring the solution is scalable and that you can maintain control and security of your data. Evaluate whether the vendor relies on open source, uses a wrapper approach or has developed its own proprietary technology. Also look at deployment options like single-tenant or private cloud and the quality of their infrastructure support. - Cost
Consider the cost implications of implementing generative AI solutions. Evaluate the pricing models offered by vendors, including licensing fees, maintenance costs, and any additional expenses associated with customization or integration. - Integration customization
Look for vendors that offer customization options and seamless integration with existing systems. This is essential to tailoring generative AI solutions to your specific organizational needs and ensuring smooth collaboration and productivity within the existing infrastructure. Additionally, consider the vendorâs compatibility with third-party services and applications commonly used in your industry to enhance productivity and collaboration. - GRC (Governance, risks, and compliance)
Evaluate how vendors handle data separation, anonymization, and compliance with privacy regulations. Prioritize vendors with strong security measures and compliance with legal requirements. Assess their data lifecycle management practices and their approach to data ownership. Look for features like redaction capabilities to maintain compliance and protect sensitive data.
We’ve put together a checklist to help you evaluate vendors and select the right solution. This checklist is based on âquestions that CIOs at global brands have asked us in their own exploration processes.
Best practices for selecting the right generative AI solution
To pick the perfect generative AI solution for your business, youâll need to kick things off with some key decisions:
- Do we build our own generative AI solution from scratch?
- If not, do we go for a full-stack platform or a point solution?
Here are some steps to guide you through these choices and ensure youâre following best practices:
Balance build-vs-buy decisions
Should you buy an off-the-shelf enterprise solution, or should you roll up your sleeves and build something custom? This decision is tricky enough when youâre dealing with something like project management software or an enterprise CRM. But throw generative AI into the mix, and things get even trickier and a bit more overwhelming.
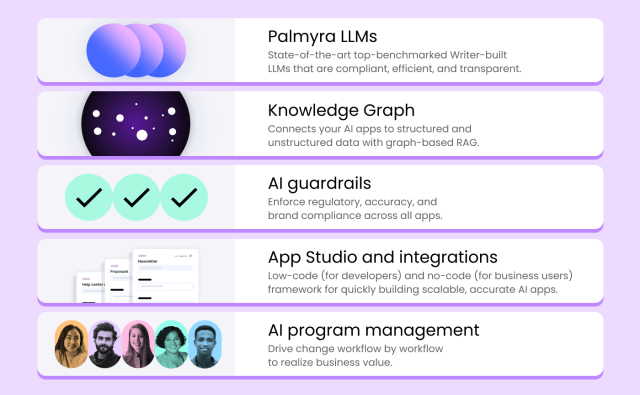
Buying a solution
If youâre like most companies, buying a generative AI solution is probably your best bet. Itâs faster and simpler than building one from scratch, and it doesnât lean heavily on your teamâs tech skills. Youâll still need someone like an AI program director to handle setup and keep things running smoothly.
- Flexibility: If your company has specific requirements, buy a product that can be customized or integrated with your existing systems.
- Vendor support: Choose a vendor who knows your industry inside and out. The right partner can make everything easier and more effective.
Building a solution
If your companyâs needs are unique, crafting a custom solution might be the way to go. This route lets you address specific challenges head-on but be ready for a long-term commitment to tweaking and improving the system.
- Ongoing commitment: Youâll need to keep refining the solution, fixing bugs, and adding new features.
- Resource-intensive: Be prepared to bring in new talent if your current team doesnât have the skills needed.
What are the tradeoffs of building a custom stack?
If your company is contemplating building a generative AI solution versus buying a product, you have to think beyond the output. Building involves input as well, in terms of time and expertise. The tradeoff is a solution fully tailored to your needs, but it still comes with quantifiable costs:
- Complexity and integration challenges
Stitching together various technology vendors can be slow, risky, and resource-intensive. Integration may not achieve the desired accuracy, and the evolving technology requires ongoing maintenance. - Development and maintenance costs
Building generative AI requires investment in servers, databases, storage, and skilled engineers. These costs can quickly add up. - Cost of LLM and RAG
Using large language models (LLMs) and retrieval augmented generation (RAG) approaches can be expensive, especially at scale. RAG solutions, like vector retrieval, require significant computational power. - Time to deployment
Building a generative AI solution takes time (many companies report six months or more), while commercial solutions enable faster deployment within weeks. - Quality of output
In-house generative AI solutions often fall short of expectations, with 61% of companies experiencing accuracy issues and only 17% rating their in-house solutions as excellent in overall performance. - Security and compliance
Integrating an LLM into an in-house solution raises data protection and compliance concerns. - Change management and adoption
In-house solutions may require additional investment in change management efforts, impacting ROI.
Whether you decide to buy or build your generative AI solution, youâll need some internal support to manage the project. Consider whether your team is up to the task of handling ongoing maintenance and if you have the right expertise on board. When buying, itâs critical to choose a vendor that not only has a solid track record with businesses like yours but also deeply understands the specific needs of your industry.
Compare the options: full-stack platform vs point solutions only
Letâs say youâve decided that buying a solution is the way to go. Youâll now need to decide on the kind of solution that’ll work best for your organization. The two main types of generative AI solutions on the market today are full-stack platforms (like Writer) or stand-alone point solutions (think chat assistants like ChatGPT or use-case specific AI tools like Grammarly).
Letâs weigh the pros and cons of each:
Full-stack generative AI platform
- Offers a centralized and scalable solution for implementing generative AI across multiple departments and use cases within an enterprise business.
- Provides a comprehensive set of tools and resources for data management, model training, and app deployment.
- Enables collaboration and knowledge sharing among different teams and departments.
- Allows for customization and integration with existing systems and processes.
- May require a larger upfront investment, but can result in long-term cost savings and efficiency gains.
- Offers continuous support and updates from the platform provider.
Point solutions and AI assistants
- Offer a more targeted and specific solution for a particular use case or department.
- Can be quicker and easier to implement compared to a platform approach.
- May lack the scalability and flexibility of a platform approach.
- Can result in a fragmented and siloed approach to implementing generative AI within an enterprise business.
- May have a lower upfront cost, making them more accessible for smaller businesses or teams.
- May require multiple apps to be used together to cover all use cases, leading to higher costs and security risks in the long run.
DISCO’s journey with generative AI: Insights for CIOs
DISCO, a trailblazer in legal technology, embarked on integrating generative AI to enhance their e-discovery processes. E-discovery involves finding, collecting, and processing electronic data for legal cases. DISCO aimed to streamline this process, making it faster and more efficient through AI and retrieval augmented generation (RAG).
Experimentation and initial challenges
DISCO’s initial foray into generative AI began with exploring various large language models (LLMs). They recognized the potential of these models to transform their e-discovery services but faced significant challenges:
- Understanding AI capabilities: Grasping the unique capabilities of generative AI compared to traditional AI systems, particularly in handling natural language processing and generating relevant legal document summaries.
- Technological adaptation: Adapting existing systems to integrate new AI technologies while ensuring data security and operational efficiency.
Engineering challenges
Engineering a generative AI solution that met specific legal standards and operational needs was daunting. DISCO needed a solution that could:
- Handle sensitive data: Ensure the utmost security and privacy of sensitive legal data.
- Scale efficiently: Manage vast volumes of data without compromising performance.
Choosing the right solution
After rigorous testing and evaluation, DISCO chose a generative AI platform that excelled in security, performance, and cost-effectiveness. This decision was driven by:
- Data security: The chosen platform provided robust security features essential for handling sensitive legal information.
- Customization and integration: The platformâs ability to integrate seamlessly with existing systems and its adaptability to legal contexts made it the ideal choice.
Transformative vs. incremental value
Jim Snyder, Chief Architect at DISCO, emphasizes the importance of seeking transformative value from AI investments. Key insights include:
- Look beyond incremental improvements: Focus on generative AI applications that offer significant leaps in productivity and decision-making, rather than just incremental improvements.
- Focus on strategic implementation: Ensure that AI tools are implemented strategically to address specific business challenges and enhance overall operational efficiency.
Lessons for CIOs
When youâre picking a generative AI solution, make sure it fits what your business really needs. Take a cue from DISCOâ â âthey chose a platform that could securely manage tons of data and mesh well with their existing tech, which was crucial for their work in legal tech.
Keep these tips in mind:
- Tailored solutions: Choose AI solutions that are specifically tailored to the industry and operational needs of the business.
- Comprehensive evaluation: Thoroughly evaluate potential AI solutions focusing on long-term benefits and alignment with business goals.
- Aim for transformation: Don’t settle for minor improvements â choose AI that fundamentally enhances your business processes and decision-making capabilities, much like DISCO did by significantly reducing the effort required in e-discovery.
DISCOâs experience with generative AI is a real playbook for CIOs wanting to bring this technology to their own organizations. By zeroing in on strategic use and truly transformative impacts, companies can weave AI into their operations in a way that meets their specific needs and drives serious business growth.
The time is now to embrace enterprise-ready generative AI
If thereâs one piece of advice we can offer to CIOs, itâs this: donât wait to embrace AI in your organization. The truth is, your employees are likely already experimenting with AI technologies, even if theyâve been told not to. Now is the time to empower them with a secure, full-stack AI platform like Writer.
While building custom solutions may seem tempting, starting from scratch isnât necessary. There are various entry points, including the developer-friendly Writer AI Studio, that can get you to solutions faster. Additionally, consider platforms that offer seamless integration with existing data sources and workflows. These platforms can be a significant accelerator for value creation.
Donât miss out on the opportunities that AI can bring to your business. Embrace it now by partnering with an enterprise-grade vendor like Writer and discover its potential for growth and innovation.